2023-11-05 更新
Exploiting Facial Relationships and Feature Aggregation for Multi-Face Forgery Detection
Authors:Chenhao Lin, Fangbin Yi, Hang Wang, Qian Li, Deng Jingyi, Chao Shen
Face forgery techniques have emerged as a forefront concern, and numerous detection approaches have been proposed to address this challenge. However, existing methods predominantly concentrate on single-face manipulation detection, leaving the more intricate and realistic realm of multi-face forgeries relatively unexplored. This paper proposes a novel framework explicitly tailored for multi-face forgery detection,filling a critical gap in the current research. The framework mainly involves two modules:(i) a facial relationships learning module, which generates distinguishable local features for each face within images,(ii) a global feature aggregation module that leverages the mutual constraints between global and local information to enhance forgery detection accuracy.Our experimental results on two publicly available multi-face forgery datasets demonstrate that the proposed approach achieves state-of-the-art performance in multi-face forgery detection scenarios.
PDF
点此查看论文截图
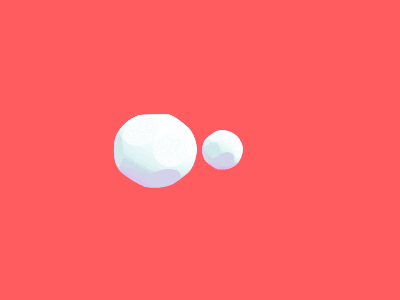
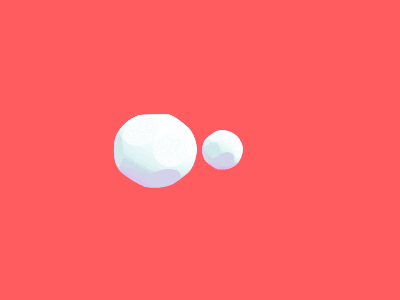
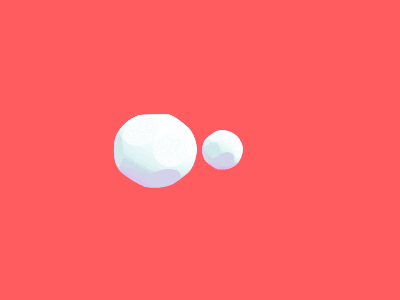
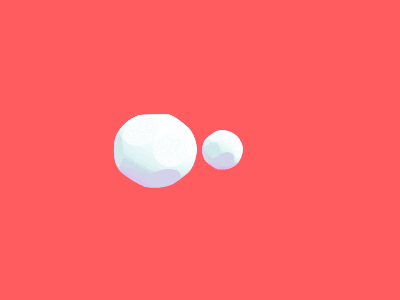
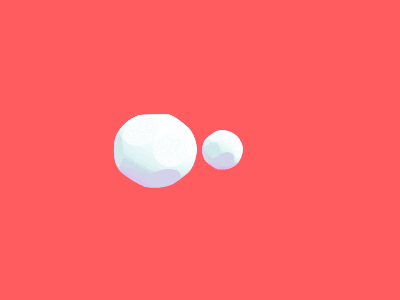
Language-driven Open-Vocabulary Keypoint Detection for Animal Body and Face
Authors:Hao Zhang, Kaipeng Zhang, Lumin Xu, Shenqi Lai, Wenqi Shao, Nanning Zheng, Ping Luo, Yu Qiao
Current approaches for image-based keypoint detection on animal (including human) body and face are limited to specific keypoints and species. We address the limitation by proposing the Open-Vocabulary Keypoint Detection (OVKD) task. It aims to use text prompts to localize arbitrary keypoints of any species. To accomplish this objective, we propose Open-Vocabulary Keypoint Detection with Semantic-feature Matching (KDSM), which utilizes both vision and language models to harness the relationship between text and vision and thus achieve keypoint detection through associating text prompt with relevant keypoint features. Additionally, KDSM integrates domain distribution matrix matching and some special designs to reinforce the relationship between language and vision, thereby improving the model’s generalizability and performance. Extensive experiments show that our proposed components bring significant performance improvements, and our overall method achieves impressive results in OVKD. Remarkably, our method outperforms the state-of-the-art few-shot keypoint detection methods using a zero-shot fashion. We will make the source code publicly accessible.
PDF
点此查看论文截图
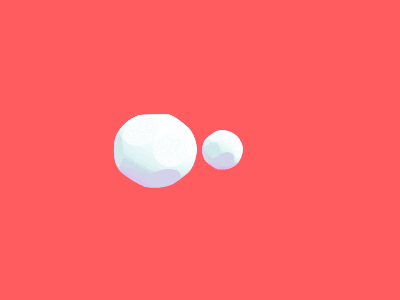
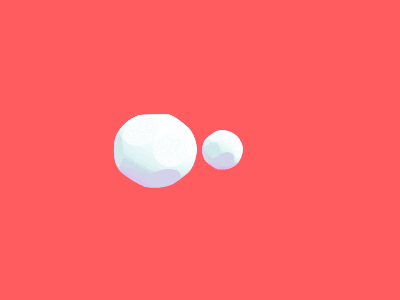
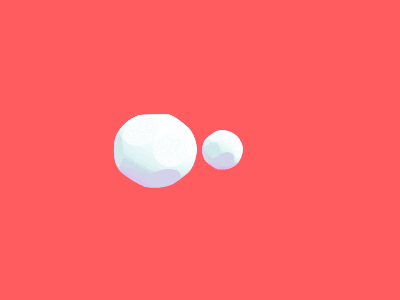
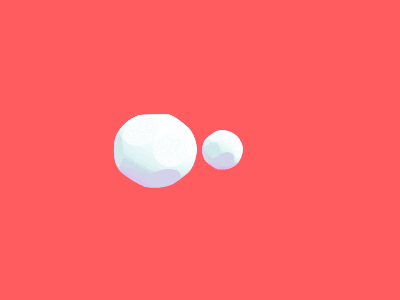
New Benchmarks for Asian Facial Recognition Tasks: Face Classification with Large Foundation Models
Authors:Jinwoo Seo, Soora Choi, Eungyeom Ha, Beomjune Kim, Dongbin Na
The face classification system is an important tool for recognizing personal identity properly. This paper introduces a new Large-Scale Korean Influencer Dataset named KoIn. Our presented dataset contains many real-world photos of Korean celebrities in various environments that might contain stage lighting, backup dancers, and background objects. These various images can be useful for training classification models classifying K-influencers. Most of the images in our proposed dataset have been collected from social network services (SNS) such as Instagram. Our dataset, KoIn, contains over 100,000 K-influencer photos from over 100 Korean celebrity classes. Moreover, our dataset provides additional hard case samples such as images including human faces with masks and hats. We note that the hard case samples are greatly useful in evaluating the robustness of the classification systems. We have extensively conducted several experiments utilizing various classification models to validate the effectiveness of our proposed dataset. Specifically, we demonstrate that recent state-of-the-art (SOTA) foundation architectures show decent classification performance when trained on our proposed dataset. In this paper, we also analyze the robustness performance against hard case samples of large-scale foundation models when we fine-tune the foundation models on the normal cases of the proposed dataset, KoIn. Our presented dataset and codes will be publicly available at https://github.com/dukong1/KoIn_Benchmark_Dataset.
PDF 13 pages
点此查看论文截图
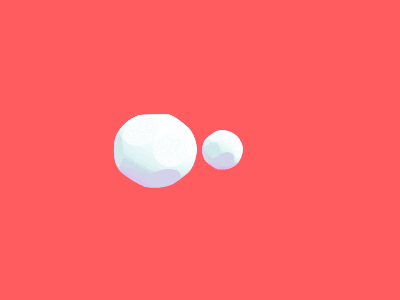
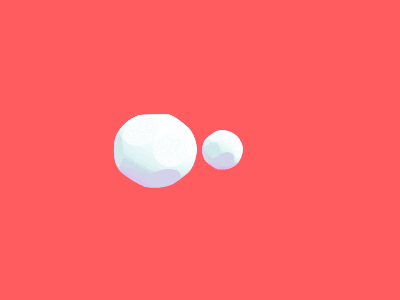
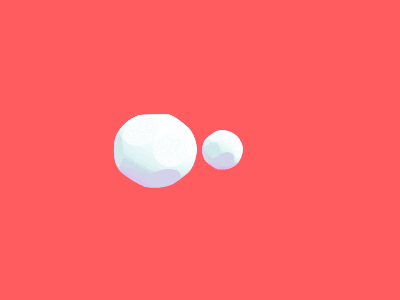
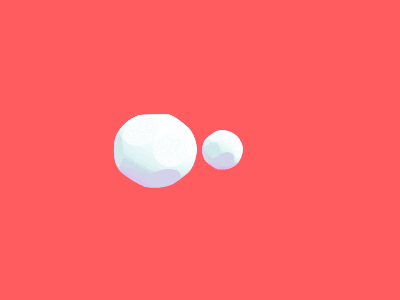
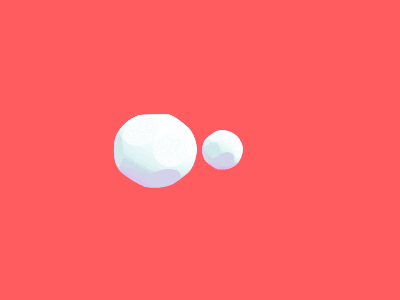
Exploring Decision-based Black-box Attacks on Face Forgery Detection
Authors:Zhaoyu Chen, Bo Li, Kaixun Jiang, Shuang Wu, Shouhong Ding, Wenqiang Zhang
Face forgery generation technologies generate vivid faces, which have raised public concerns about security and privacy. Many intelligent systems, such as electronic payment and identity verification, rely on face forgery detection. Although face forgery detection has successfully distinguished fake faces, recent studies have demonstrated that face forgery detectors are very vulnerable to adversarial examples. Meanwhile, existing attacks rely on network architectures or training datasets instead of the predicted labels, which leads to a gap in attacking deployed applications. To narrow this gap, we first explore the decision-based attacks on face forgery detection. However, applying existing decision-based attacks directly suffers from perturbation initialization failure and low image quality. First, we propose cross-task perturbation to handle initialization failures by utilizing the high correlation of face features on different tasks. Then, inspired by using frequency cues by face forgery detection, we propose the frequency decision-based attack. We add perturbations in the frequency domain and then constrain the visual quality in the spatial domain. Finally, extensive experiments demonstrate that our method achieves state-of-the-art attack performance on FaceForensics++, CelebDF, and industrial APIs, with high query efficiency and guaranteed image quality. Further, the fake faces by our method can pass face forgery detection and face recognition, which exposes the security problems of face forgery detectors.
PDF
点此查看论文截图
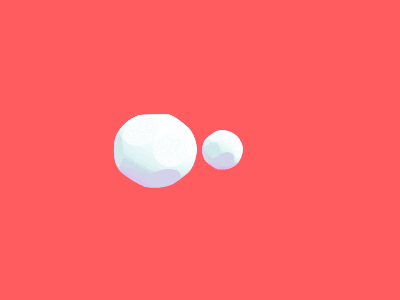
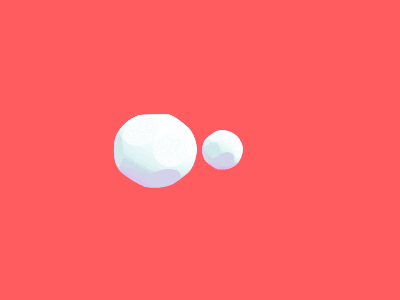
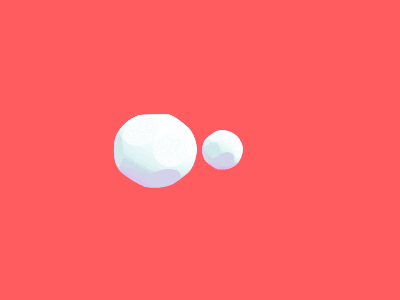
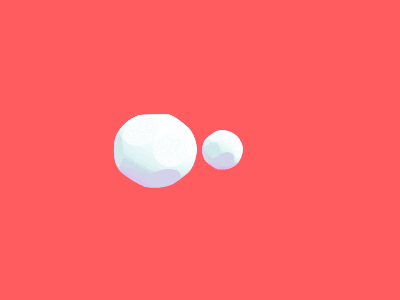
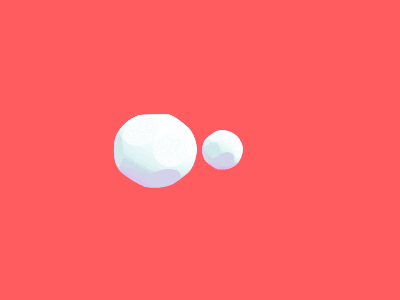
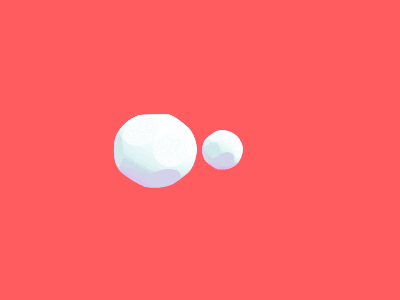
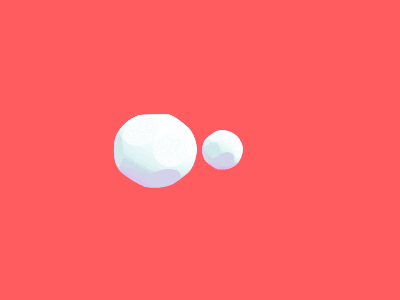
A transfer learning approach with convolutional neural network for Face Mask Detection
Authors:Abolfazl Younesi, Reza Afrouzian, Yousef Seyfari
Due to the epidemic of the coronavirus (Covid-19) and its rapid spread around the world, the world has faced an enormous crisis. To prevent the spread of the coronavirus, the World Health Organization (WHO) has introduced the use of masks and keeping social distance as the best preventive method. So, developing an automatic monitoring system for detecting facemasks in some crowded places is essential. To do this, we propose a mask recognition system based on transfer learning and Inception v3 architecture. In the proposed method, two datasets are used simultaneously for training including the Simulated Mask Face Dataset (SMFD) and MaskedFace-Net (MFN) This paper tries to increase the accuracy of the proposed system by optimally setting hyper-parameters and accurately designing the fully connected layers. The main advantage of the proposed method is that in addition to masked and unmasked faces, it can also detect cases of incorrect use of mask. Therefore, the proposed method classifies the input face images into three categories. Experimental results show the high accuracy and efficiency of the proposed method; so, this method has achieved an accuracy of 99.47% and 99.33% in training and test data respectively
PDF 9 pages, in Persian language, 8 figures
点此查看论文截图
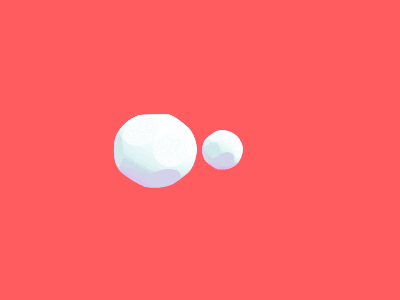
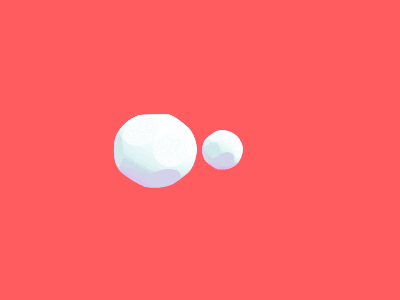
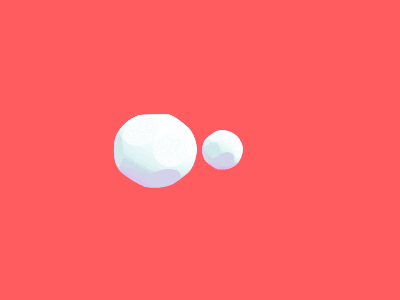
Open-Set Face Recognition with Maximal Entropy and Objectosphere Loss
Authors:Rafael Henrique Vareto, Yu Linghu, Terrance E. Boult, William Robson Schwartz, Manuel Günther
Open-set face recognition characterizes a scenario where unknown individuals, unseen during the training and enrollment stages, appear on operation time. This work concentrates on watchlists, an open-set task that is expected to operate at a low False Positive Identification Rate and generally includes only a few enrollment samples per identity. We introduce a compact adapter network that benefits from additional negative face images when combined with distinct cost functions, such as Objectosphere Loss (OS) and the proposed Maximal Entropy Loss (MEL). MEL modifies the traditional Cross-Entropy loss in favor of increasing the entropy for negative samples and attaches a penalty to known target classes in pursuance of gallery specialization. The proposed approach adopts pre-trained deep neural networks (DNNs) for face recognition as feature extractors. Then, the adapter network takes deep feature representations and acts as a substitute for the output layer of the pre-trained DNN in exchange for an agile domain adaptation. Promising results have been achieved following open-set protocols for three different datasets: LFW, IJB-C, and UCCS as well as state-of-the-art performance when supplementary negative data is properly selected to fine-tune the adapter network.
PDF Accepted for publication in Image and Vision Computing 2023
点此查看论文截图
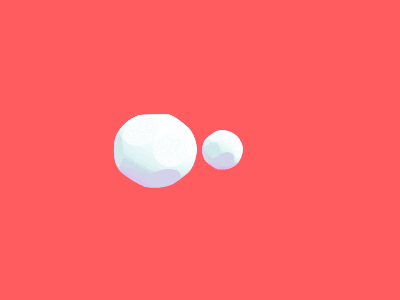
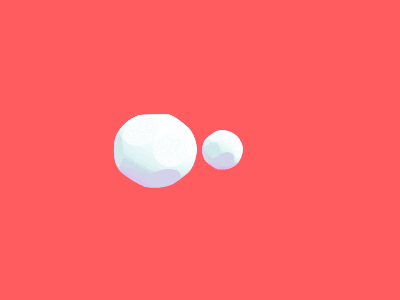
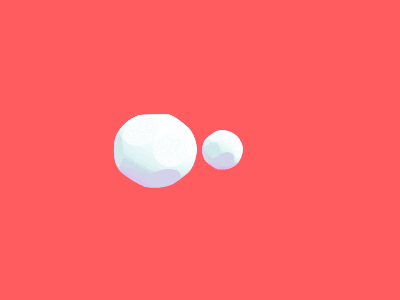