2023-05-05 更新
NaturalSpeech 2: Latent Diffusion Models are Natural and Zero-Shot Speech and Singing Synthesizers
Authors:Kai Shen, Zeqian Ju, Xu Tan, Yanqing Liu, Yichong Leng, Lei He, Tao Qin, Sheng Zhao, Jiang Bian
Scaling text-to-speech (TTS) to large-scale, multi-speaker, and in-the-wild datasets is important to capture the diversity in human speech such as speaker identities, prosodies, and styles (e.g., singing). Current large TTS systems usually quantize speech into discrete tokens and use language models to generate these tokens one by one, which suffer from unstable prosody, word skipping/repeating issue, and poor voice quality. In this paper, we develop NaturalSpeech 2, a TTS system that leverages a neural audio codec with residual vector quantizers to get the quantized latent vectors and uses a diffusion model to generate these latent vectors conditioned on text input. To enhance the zero-shot capability that is important to achieve diverse speech synthesis, we design a speech prompting mechanism to facilitate in-context learning in the diffusion model and the duration/pitch predictor. We scale NaturalSpeech 2 to large-scale datasets with 44K hours of speech and singing data and evaluate its voice quality on unseen speakers. NaturalSpeech 2 outperforms previous TTS systems by a large margin in terms of prosody/timbre similarity, robustness, and voice quality in a zero-shot setting, and performs novel zero-shot singing synthesis with only a speech prompt. Audio samples are available at https://speechresearch.github.io/naturalspeech2.
PDF A large-scale text-to-speech and singing voice synthesis system with latent diffusion models
点此查看论文截图
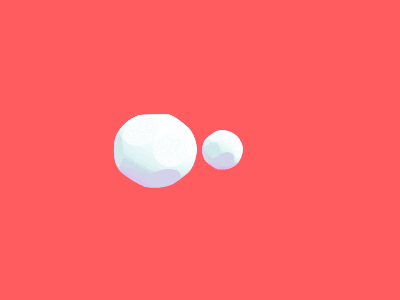
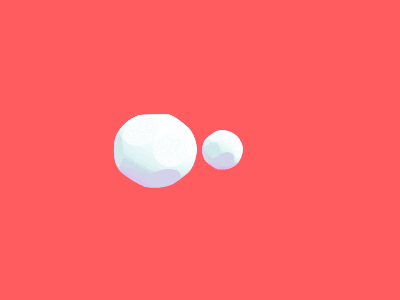
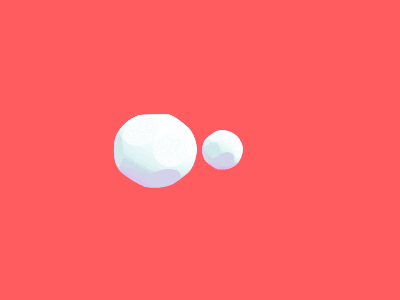
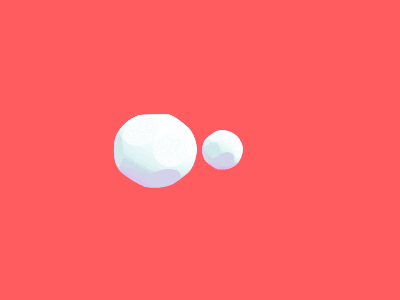
Hybrid AHS: A Hybrid of Kalman Filter and Deep Learning for Acoustic Howling Suppression
Authors:Hao Zhang, Meng Yu, Yuzhong Wu, Tao Yu, Dong Yu
Deep learning has been recently introduced for efficient acoustic howling suppression (AHS). However, the recurrent nature of howling creates a mismatch between offline training and streaming inference, limiting the quality of enhanced speech. To address this limitation, we propose a hybrid method that combines a Kalman filter with a self-attentive recurrent neural network (SARNN) to leverage their respective advantages for robust AHS. During offline training, a pre-processed signal obtained from the Kalman filter and an ideal microphone signal generated via teacher-forced training strategy are used to train the deep neural network (DNN). During streaming inference, the DNN’s parameters are fixed while its output serves as a reference signal for updating the Kalman filter. Evaluation in both offline and streaming inference scenarios using simulated and real-recorded data shows that the proposed method efficiently suppresses howling and consistently outperforms baselines.
PDF submitted to INTERSPEECH 2023
点此查看论文截图
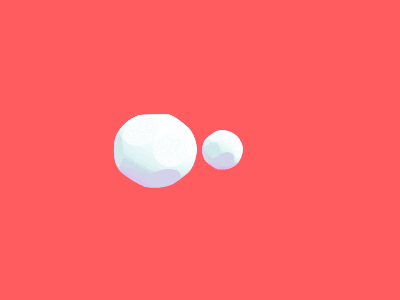
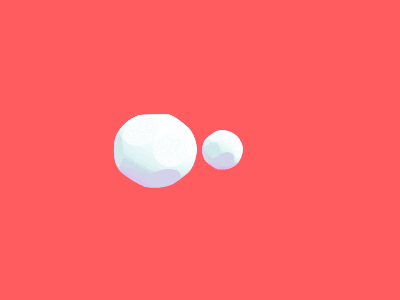
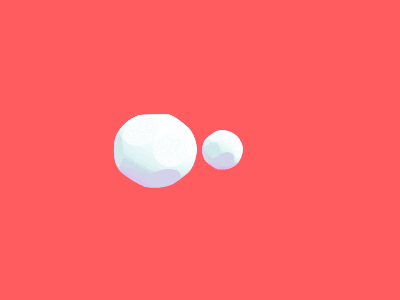
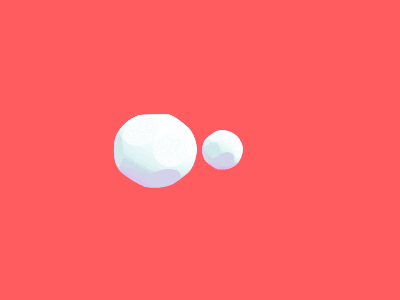
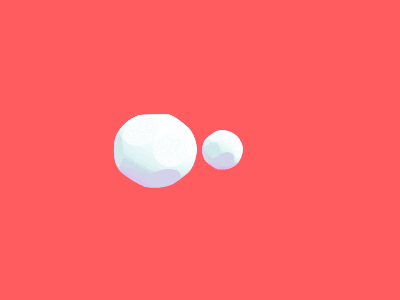
Towards Weakly-Supervised Hate Speech Classification Across Datasets
Authors:Yiping Jin, Leo Wanner, Vishakha Laxman Kadam, Alexander Shvets
As pointed out by several scholars, current research on hate speech (HS) recognition is characterized by unsystematic data creation strategies and diverging annotation schemata. Subsequently, supervised-learning models tend to generalize poorly to datasets they were not trained on, and the performance of the models trained on datasets labeled using different HS taxonomies cannot be compared. To ease this problem, we propose applying extremely weak supervision that only relies on the class name rather than on class samples from the annotated data. We demonstrate the effectiveness of a state-of-the-art weakly-supervised text classification model in various in-dataset and cross-dataset settings. Furthermore, we conduct an in-depth quantitative and qualitative analysis of the source of poor generalizability of HS classification models.
PDF
点此查看论文截图
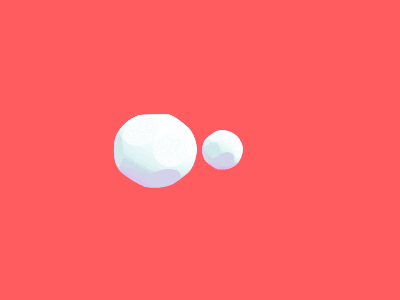
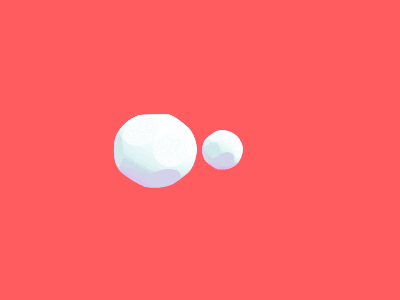
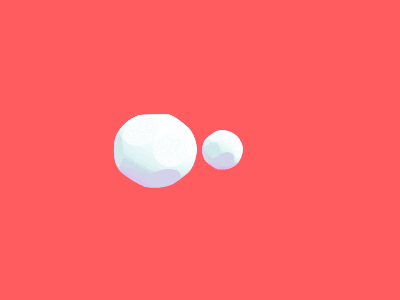
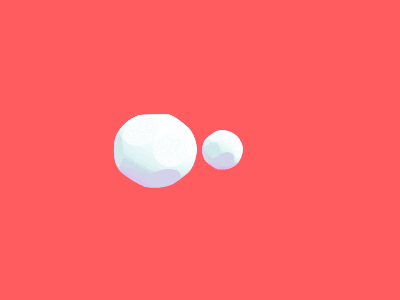
End-to-end spoken language understanding using joint CTC loss and self-supervised, pretrained acoustic encoders
Authors:Jixuan Wang, Martin Radfar, Kai Wei, Clement Chung
It is challenging to extract semantic meanings directly from audio signals in spoken language understanding (SLU), due to the lack of textual information. Popular end-to-end (E2E) SLU models utilize sequence-to-sequence automatic speech recognition (ASR) models to extract textual embeddings as input to infer semantics, which, however, require computationally expensive auto-regressive decoding. In this work, we leverage self-supervised acoustic encoders fine-tuned with Connectionist Temporal Classification (CTC) to extract textual embeddings and use joint CTC and SLU losses for utterance-level SLU tasks. Experiments show that our model achieves 4% absolute improvement over the the state-of-the-art (SOTA) dialogue act classification model on the DSTC2 dataset and 1.3% absolute improvement over the SOTA SLU model on the SLURP dataset.
PDF ICASSP 2023
点此查看论文截图
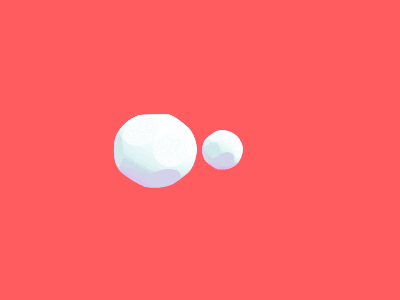
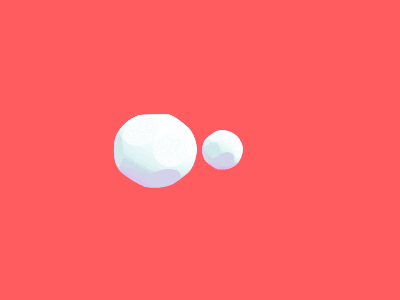
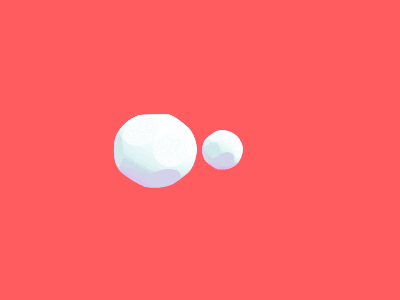
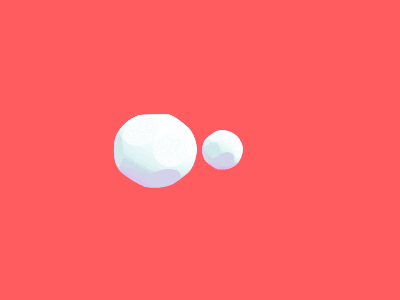
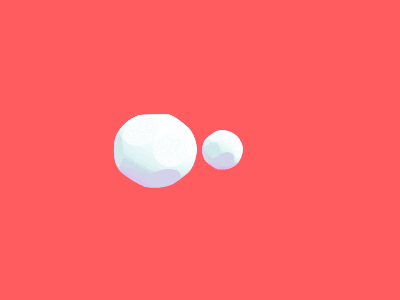