2024-04-03 更新
Condition-Aware Neural Network for Controlled Image Generation
Authors:Han Cai, Muyang Li, Zhuoyang Zhang, Qinsheng Zhang, Ming-Yu Liu, Song Han
We present Condition-Aware Neural Network (CAN), a new method for adding control to image generative models. In parallel to prior conditional control methods, CAN controls the image generation process by dynamically manipulating the weight of the neural network. This is achieved by introducing a condition-aware weight generation module that generates conditional weight for convolution/linear layers based on the input condition. We test CAN on class-conditional image generation on ImageNet and text-to-image generation on COCO. CAN consistently delivers significant improvements for diffusion transformer models, including DiT and UViT. In particular, CAN combined with EfficientViT (CaT) achieves 2.78 FID on ImageNet 512x512, surpassing DiT-XL/2 while requiring 52x fewer MACs per sampling step.
PDF CVPR 2024
点此查看论文截图
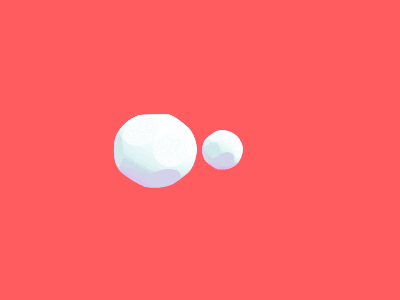
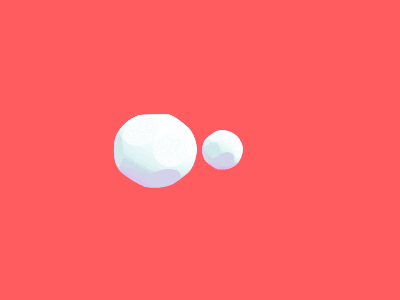
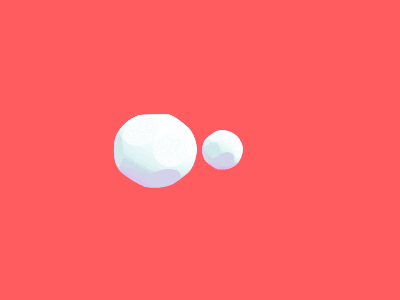
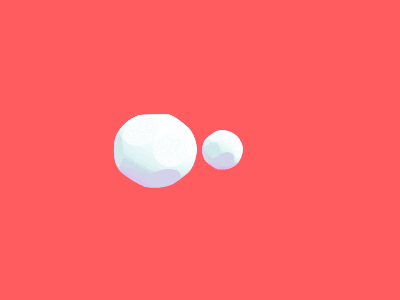
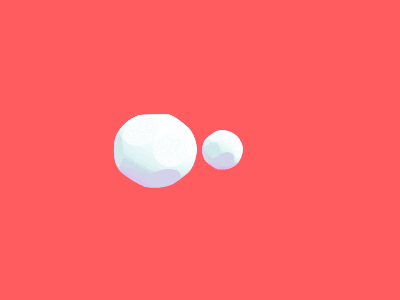
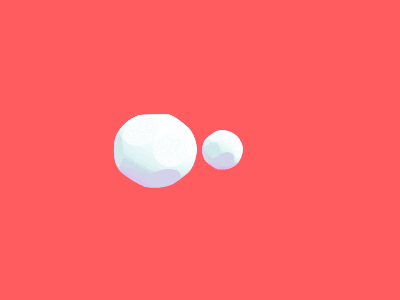
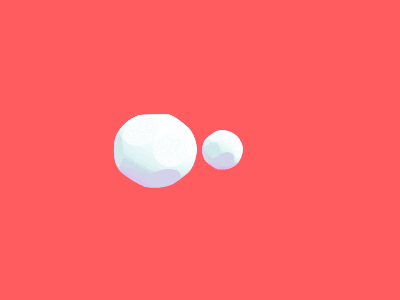
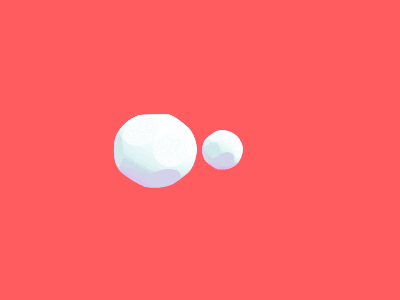
Video Interpolation with Diffusion Models
Authors:Siddhant Jain, Daniel Watson, Eric Tabellion, Aleksander Hołyński, Ben Poole, Janne Kontkanen
We present VIDIM, a generative model for video interpolation, which creates short videos given a start and end frame. In order to achieve high fidelity and generate motions unseen in the input data, VIDIM uses cascaded diffusion models to first generate the target video at low resolution, and then generate the high-resolution video conditioned on the low-resolution generated video. We compare VIDIM to previous state-of-the-art methods on video interpolation, and demonstrate how such works fail in most settings where the underlying motion is complex, nonlinear, or ambiguous while VIDIM can easily handle such cases. We additionally demonstrate how classifier-free guidance on the start and end frame and conditioning the super-resolution model on the original high-resolution frames without additional parameters unlocks high-fidelity results. VIDIM is fast to sample from as it jointly denoises all the frames to be generated, requires less than a billion parameters per diffusion model to produce compelling results, and still enjoys scalability and improved quality at larger parameter counts.
PDF CVPR 2024, Project page at https://vidim-interpolation.github.io/
点此查看论文截图
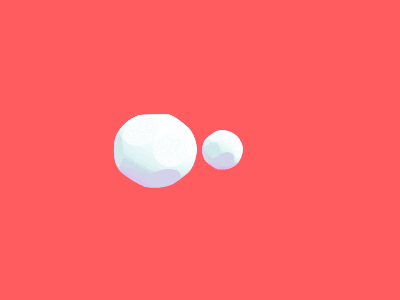
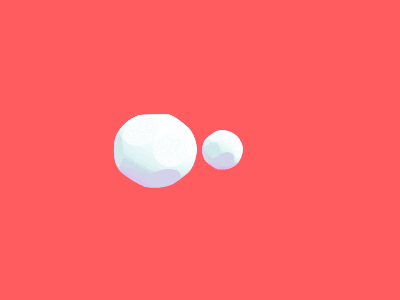
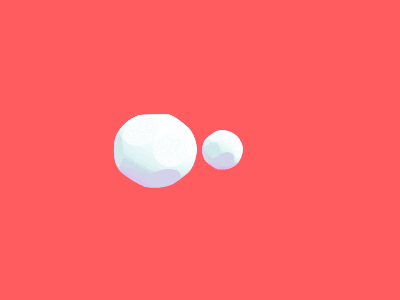
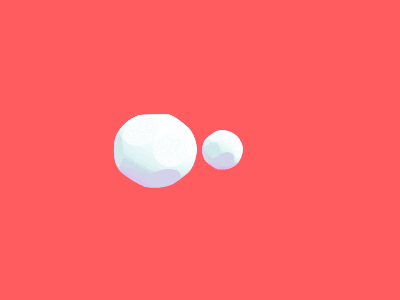
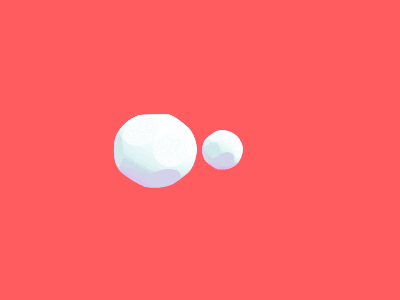
StructLDM: Structured Latent Diffusion for 3D Human Generation
Authors:Tao Hu, Fangzhou Hong, Ziwei Liu
Recent 3D human generative models have achieved remarkable progress by learning 3D-aware GANs from 2D images. However, existing 3D human generative methods model humans in a compact 1D latent space, ignoring the articulated structure and semantics of human body topology. In this paper, we explore more expressive and higher-dimensional latent space for 3D human modeling and propose StructLDM, a diffusion-based unconditional 3D human generative model, which is learned from 2D images. StructLDM solves the challenges imposed due to the high-dimensional growth of latent space with three key designs: 1) A semantic structured latent space defined on the dense surface manifold of a statistical human body template. 2) A structured 3D-aware auto-decoder that factorizes the global latent space into several semantic body parts parameterized by a set of conditional structured local NeRFs anchored to the body template, which embeds the properties learned from the 2D training data and can be decoded to render view-consistent humans under different poses and clothing styles. 3) A structured latent diffusion model for generative human appearance sampling. Extensive experiments validate StructLDM’s state-of-the-art generation performance and illustrate the expressiveness of the structured latent space over the well-adopted 1D latent space. Notably, StructLDM enables different levels of controllable 3D human generation and editing, including pose/view/shape control, and high-level tasks including compositional generations, part-aware clothing editing, 3D virtual try-on, etc. Our project page is at: https://taohuumd.github.io/projects/StructLDM/.
PDF Project page: https://taohuumd.github.io/projects/StructLDM/
点此查看论文截图
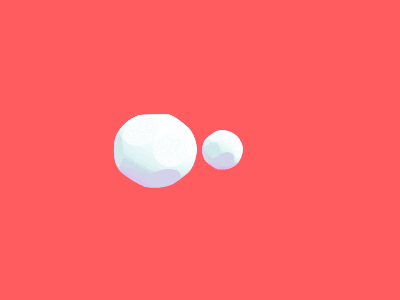
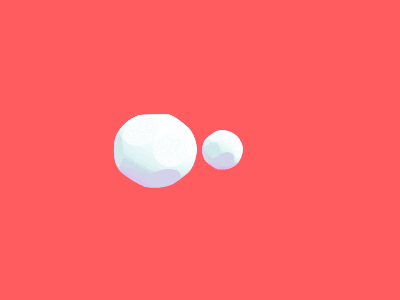
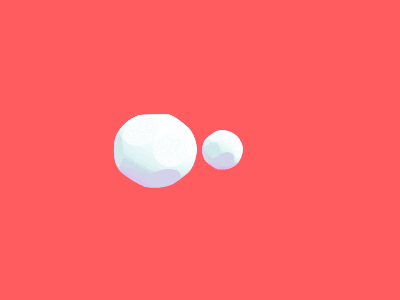
Evaluating Text-to-Visual Generation with Image-to-Text Generation
Authors:Zhiqiu Lin, Deepak Pathak, Baiqi Li, Jiayao Li, Xide Xia, Graham Neubig, Pengchuan Zhang, Deva Ramanan
Despite significant progress in generative AI, comprehensive evaluation remains challenging because of the lack of effective metrics and standardized benchmarks. For instance, the widely-used CLIPScore measures the alignment between a (generated) image and text prompt, but it fails to produce reliable scores for complex prompts involving compositions of objects, attributes, and relations. One reason is that text encoders of CLIP can notoriously act as a “bag of words”, conflating prompts such as “the horse is eating the grass” with “the grass is eating the horse”. To address this, we introduce the VQAScore, which uses a visual-question-answering (VQA) model to produce an alignment score by computing the probability of a “Yes” answer to a simple “Does this figure show ‘{text}’?” question. Though simpler than prior art, VQAScore computed with off-the-shelf models produces state-of-the-art results across many (8) image-text alignment benchmarks. We also compute VQAScore with an in-house model that follows best practices in the literature. For example, we use a bidirectional image-question encoder that allows image embeddings to depend on the question being asked (and vice versa). Our in-house model, CLIP-FlanT5, outperforms even the strongest baselines that make use of the proprietary GPT-4V. Interestingly, although we train with only images, VQAScore can also align text with video and 3D models. VQAScore allows researchers to benchmark text-to-visual generation using complex texts that capture the compositional structure of real-world prompts. We introduce GenAI-Bench, a more challenging benchmark with 1,600 compositional text prompts that require parsing scenes, objects, attributes, relationships, and high-order reasoning like comparison and logic. GenAI-Bench also offers over 15,000 human ratings for leading image and video generation models such as Stable Diffusion, DALL-E 3, and Gen2.
PDF We open-source our data, model, and code at: https://github.com/linzhiqiu/t2v_metrics ; Project page: https://linzhiqiu.github.io/papers/vqascore
点此查看论文截图
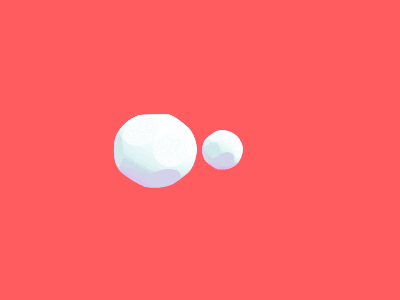
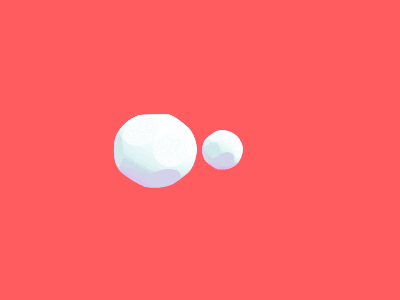
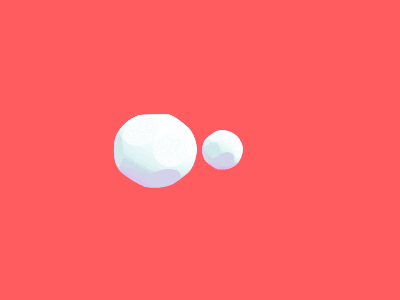
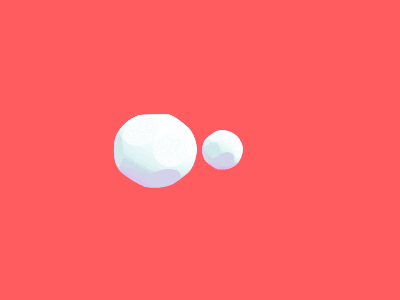
Measuring Style Similarity in Diffusion Models
Authors:Gowthami Somepalli, Anubhav Gupta, Kamal Gupta, Shramay Palta, Micah Goldblum, Jonas Geiping, Abhinav Shrivastava, Tom Goldstein
Generative models are now widely used by graphic designers and artists. Prior works have shown that these models remember and often replicate content from their training data during generation. Hence as their proliferation increases, it has become important to perform a database search to determine whether the properties of the image are attributable to specific training data, every time before a generated image is used for professional purposes. Existing tools for this purpose focus on retrieving images of similar semantic content. Meanwhile, many artists are concerned with style replication in text-to-image models. We present a framework for understanding and extracting style descriptors from images. Our framework comprises a new dataset curated using the insight that style is a subjective property of an image that captures complex yet meaningful interactions of factors including but not limited to colors, textures, shapes, etc. We also propose a method to extract style descriptors that can be used to attribute style of a generated image to the images used in the training dataset of a text-to-image model. We showcase promising results in various style retrieval tasks. We also quantitatively and qualitatively analyze style attribution and matching in the Stable Diffusion model. Code and artifacts are available at https://github.com/learn2phoenix/CSD.
PDF
点此查看论文截图
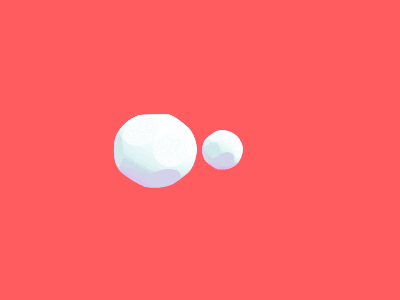
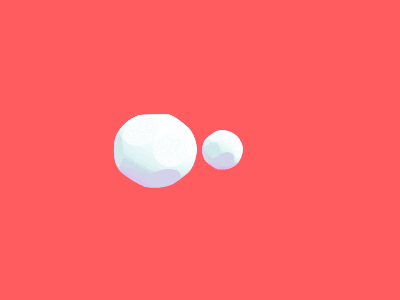
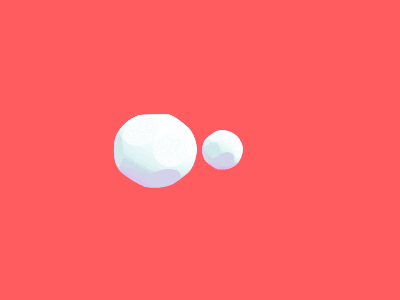
MagicMirror: Fast and High-Quality Avatar Generation with a Constrained Search Space
Authors:Armand Comas-Massagué, Di Qiu, Menglei Chai, Marcel Bühler, Amit Raj, Ruiqi Gao, Qiangeng Xu, Mark Matthews, Paulo Gotardo, Octavia Camps, Sergio Orts-Escolano, Thabo Beeler
We introduce a novel framework for 3D human avatar generation and personalization, leveraging text prompts to enhance user engagement and customization. Central to our approach are key innovations aimed at overcoming the challenges in photo-realistic avatar synthesis. Firstly, we utilize a conditional Neural Radiance Fields (NeRF) model, trained on a large-scale unannotated multi-view dataset, to create a versatile initial solution space that accelerates and diversifies avatar generation. Secondly, we develop a geometric prior, leveraging the capabilities of Text-to-Image Diffusion Models, to ensure superior view invariance and enable direct optimization of avatar geometry. These foundational ideas are complemented by our optimization pipeline built on Variational Score Distillation (VSD), which mitigates texture loss and over-saturation issues. As supported by our extensive experiments, these strategies collectively enable the creation of custom avatars with unparalleled visual quality and better adherence to input text prompts. You can find more results and videos in our website: https://syntec-research.github.io/MagicMirror
PDF
点此查看论文截图
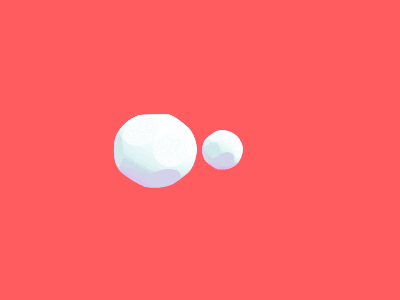
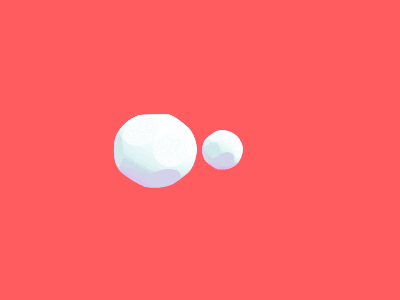
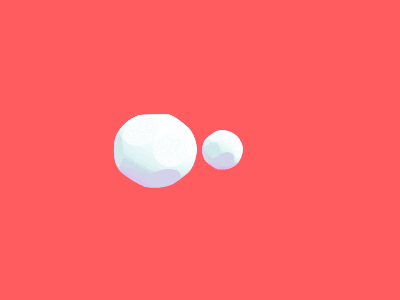
DPMesh: Exploiting Diffusion Prior for Occluded Human Mesh Recovery
Authors:Yixuan Zhu, Ao Li, Yansong Tang, Wenliang Zhao, Jie Zhou, Jiwen Lu
The recovery of occluded human meshes presents challenges for current methods due to the difficulty in extracting effective image features under severe occlusion. In this paper, we introduce DPMesh, an innovative framework for occluded human mesh recovery that capitalizes on the profound diffusion prior about object structure and spatial relationships embedded in a pre-trained text-to-image diffusion model. Unlike previous methods reliant on conventional backbones for vanilla feature extraction, DPMesh seamlessly integrates the pre-trained denoising U-Net with potent knowledge as its image backbone and performs a single-step inference to provide occlusion-aware information. To enhance the perception capability for occluded poses, DPMesh incorporates well-designed guidance via condition injection, which produces effective controls from 2D observations for the denoising U-Net. Furthermore, we explore a dedicated noisy key-point reasoning approach to mitigate disturbances arising from occlusion and crowded scenarios. This strategy fully unleashes the perceptual capability of the diffusion prior, thereby enhancing accuracy. Extensive experiments affirm the efficacy of our framework, as we outperform state-of-the-art methods on both occlusion-specific and standard datasets. The persuasive results underscore its ability to achieve precise and robust 3D human mesh recovery, particularly in challenging scenarios involving occlusion and crowded scenes.
PDF Accepted by IEEE/CVF Conference on Computer Vision and Pattern Recognition (CVPR) 2024
点此查看论文截图
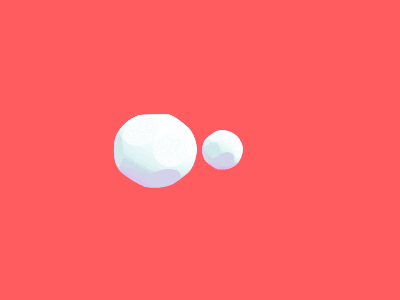
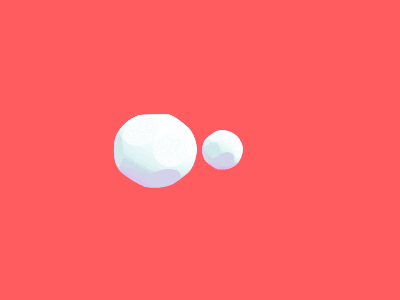
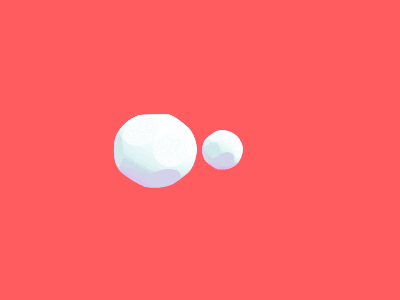
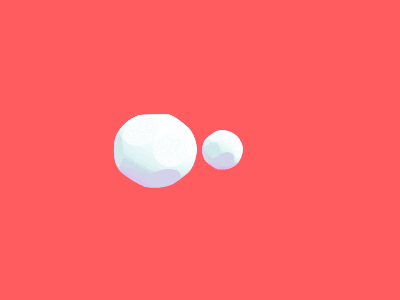
Diffusion Deepfake
Authors:Chaitali Bhattacharyya, Hanxiao Wang, Feng Zhang, Sungho Kim, Xiatian Zhu
Recent progress in generative AI, primarily through diffusion models, presents significant challenges for real-world deepfake detection. The increased realism in image details, diverse content, and widespread accessibility to the general public complicates the identification of these sophisticated deepfakes. Acknowledging the urgency to address the vulnerability of current deepfake detectors to this evolving threat, our paper introduces two extensive deepfake datasets generated by state-of-the-art diffusion models as other datasets are less diverse and low in quality. Our extensive experiments also showed that our dataset is more challenging compared to the other face deepfake datasets. Our strategic dataset creation not only challenge the deepfake detectors but also sets a new benchmark for more evaluation. Our comprehensive evaluation reveals the struggle of existing detection methods, often optimized for specific image domains and manipulations, to effectively adapt to the intricate nature of diffusion deepfakes, limiting their practical utility. To address this critical issue, we investigate the impact of enhancing training data diversity on representative detection methods. This involves expanding the diversity of both manipulation techniques and image domains. Our findings underscore that increasing training data diversity results in improved generalizability. Moreover, we propose a novel momentum difficulty boosting strategy to tackle the additional challenge posed by training data heterogeneity. This strategy dynamically assigns appropriate sample weights based on learning difficulty, enhancing the model’s adaptability to both easy and challenging samples. Extensive experiments on both existing and newly proposed benchmarks demonstrate that our model optimization approach surpasses prior alternatives significantly.
PDF 28 pages including Supplementary material
点此查看论文截图
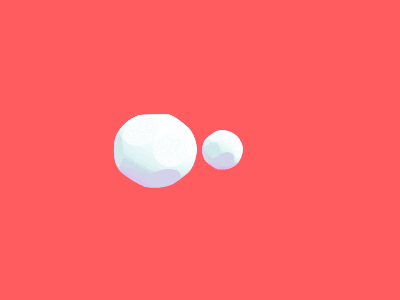
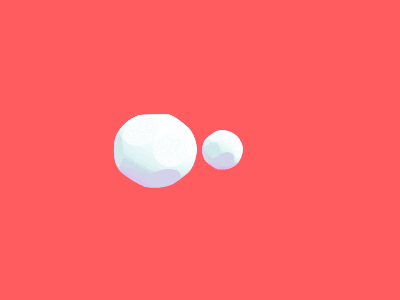
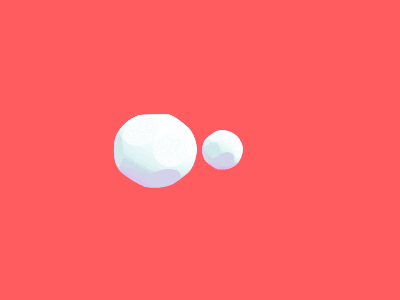
FashionEngine: Interactive Generation and Editing of 3D Clothed Humans
Authors:Tao Hu, Fangzhou Hong, Zhaoxi Chen, Ziwei Liu
We present FashionEngine, an interactive 3D human generation and editing system that allows us to design 3D digital humans in a way that aligns with how humans interact with the world, such as natural languages, visual perceptions, and hand-drawing. FashionEngine automates the 3D human production with three key components: 1) A pre-trained 3D human diffusion model that learns to model 3D humans in a semantic UV latent space from 2D image training data, which provides strong priors for diverse generation and editing tasks. 2) Multimodality-UV Space encoding the texture appearance, shape topology, and textual semantics of human clothing in a canonical UV-aligned space, which faithfully aligns the user multimodal inputs with the implicit UV latent space for controllable 3D human editing. The multimodality-UV space is shared across different user inputs, such as texts, images, and sketches, which enables various joint multimodal editing tasks. 3) Multimodality-UV Aligned Sampler learns to sample high-quality and diverse 3D humans from the diffusion prior for multimodal user inputs. Extensive experiments validate FashionEngine’s state-of-the-art performance for conditional generation/editing tasks. In addition, we present an interactive user interface for our FashionEngine that enables both conditional and unconditional generation tasks, and editing tasks including pose/view/shape control, text-, image-, and sketch-driven 3D human editing and 3D virtual try-on, in a unified framework. Our project page is at: https://taohuumd.github.io/projects/FashionEngine.
PDF Project Page: https://taohuumd.github.io/projects/FashionEngine
点此查看论文截图
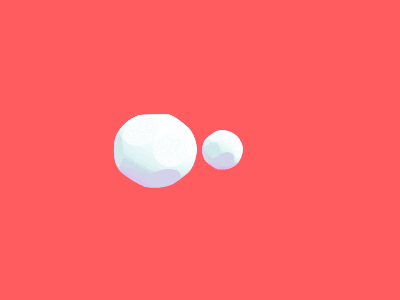
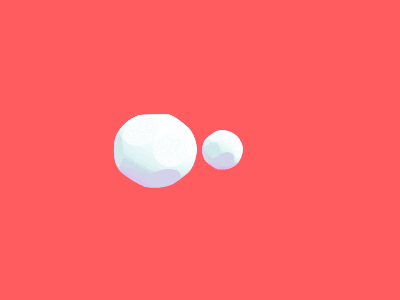
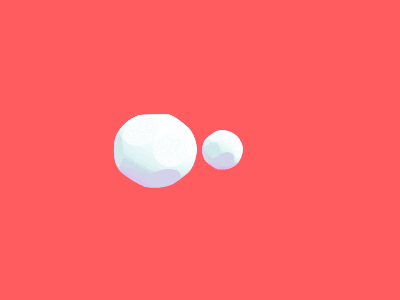
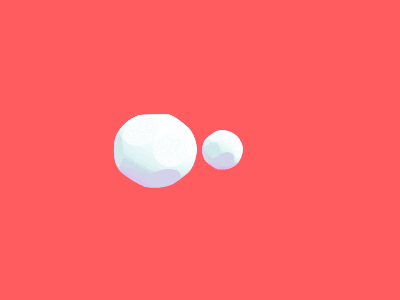
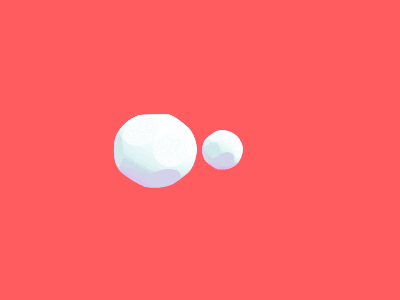
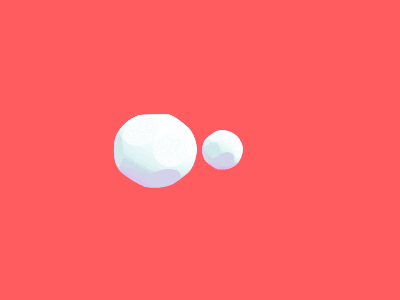
AddSR: Accelerating Diffusion-based Blind Super-Resolution with Adversarial Diffusion Distillation
Authors:Rui Xie, Ying Tai, Kai Zhang, Zhenyu Zhang, Jun Zhou, Jian Yang
Blind super-resolution methods based on stable diffusion showcase formidable generative capabilities in reconstructing clear high-resolution images with intricate details from low-resolution inputs. However, their practical applicability is often hampered by poor efficiency, stemming from the requirement of thousands or hundreds of sampling steps. Inspired by the efficient text-to-image approach adversarial diffusion distillation (ADD), we design AddSR to address this issue by incorporating the ideas of both distillation and ControlNet. Specifically, we first propose a prediction-based self-refinement strategy to provide high-frequency information in the student model output with marginal additional time cost. Furthermore, we refine the training process by employing HR images, rather than LR images, to regulate the teacher model, providing a more robust constraint for distillation. Second, we introduce a timestep-adapting loss to address the perception-distortion imbalance problem introduced by ADD. Extensive experiments demonstrate our AddSR generates better restoration results, while achieving faster speed than previous SD-based state-of-the-art models (e.g., 7x faster than SeeSR).
PDF
点此查看论文截图
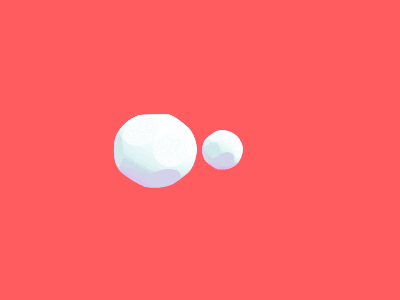
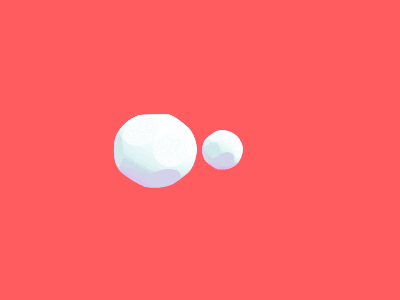
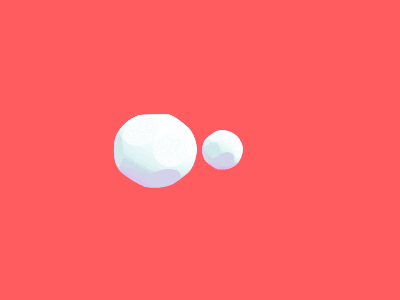
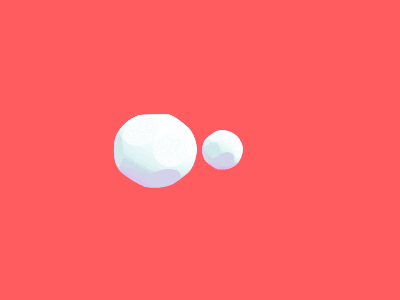
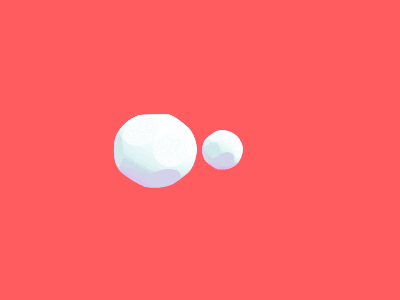
Co-Speech Gesture Video Generation via Motion-Decoupled Diffusion Model
Authors:Xu He, Qiaochu Huang, Zhensong Zhang, Zhiwei Lin, Zhiyong Wu, Sicheng Yang, Minglei Li, Zhiyi Chen, Songcen Xu, Xiaofei Wu
Co-speech gestures, if presented in the lively form of videos, can achieve superior visual effects in human-machine interaction. While previous works mostly generate structural human skeletons, resulting in the omission of appearance information, we focus on the direct generation of audio-driven co-speech gesture videos in this work. There are two main challenges: 1) A suitable motion feature is needed to describe complex human movements with crucial appearance information. 2) Gestures and speech exhibit inherent dependencies and should be temporally aligned even of arbitrary length. To solve these problems, we present a novel motion-decoupled framework to generate co-speech gesture videos. Specifically, we first introduce a well-designed nonlinear TPS transformation to obtain latent motion features preserving essential appearance information. Then a transformer-based diffusion model is proposed to learn the temporal correlation between gestures and speech, and performs generation in the latent motion space, followed by an optimal motion selection module to produce long-term coherent and consistent gesture videos. For better visual perception, we further design a refinement network focusing on missing details of certain areas. Extensive experimental results show that our proposed framework significantly outperforms existing approaches in both motion and video-related evaluations. Our code, demos, and more resources are available at https://github.com/thuhcsi/S2G-MDDiffusion.
PDF 22 pages, 8 figures, CVPR 2024
点此查看论文截图
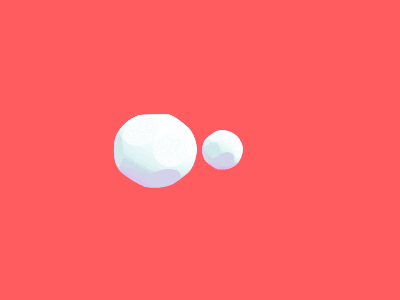
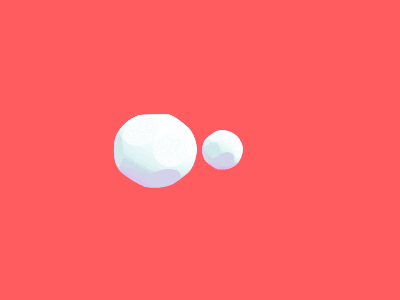
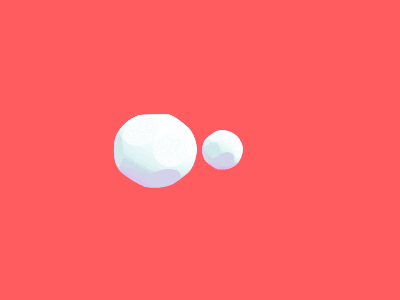
Bi-LORA: A Vision-Language Approach for Synthetic Image Detection
Authors:Mamadou Keita, Wassim Hamidouche, Hessen Bougueffa Eutamene, Abdenour Hadid, Abdelmalik Taleb-Ahmed
Advancements in deep image synthesis techniques, such as generative adversarial networks (GANs) and diffusion models (DMs), have ushered in an era of generating highly realistic images. While this technological progress has captured significant interest, it has also raised concerns about the potential difficulty in distinguishing real images from their synthetic counterparts. This paper takes inspiration from the potent convergence capabilities between vision and language, coupled with the zero-shot nature of vision-language models (VLMs). We introduce an innovative method called Bi-LORA that leverages VLMs, combined with low-rank adaptation (LORA) tuning techniques, to enhance the precision of synthetic image detection for unseen model-generated images. The pivotal conceptual shift in our methodology revolves around reframing binary classification as an image captioning task, leveraging the distinctive capabilities of cutting-edge VLM, notably bootstrapping language image pre-training (BLIP2). Rigorous and comprehensive experiments are conducted to validate the effectiveness of our proposed approach, particularly in detecting unseen diffusion-generated images from unknown diffusion-based generative models during training, showcasing robustness to noise, and demonstrating generalization capabilities to GANs. The obtained results showcase an impressive average accuracy of 93.41% in synthetic image detection on unseen generation models. The code and models associated with this research can be publicly accessed at https://github.com/Mamadou-Keita/VLM-DETECT.
PDF
点此查看论文截图
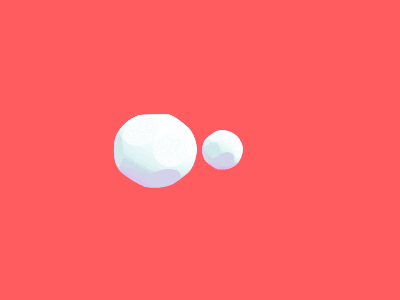
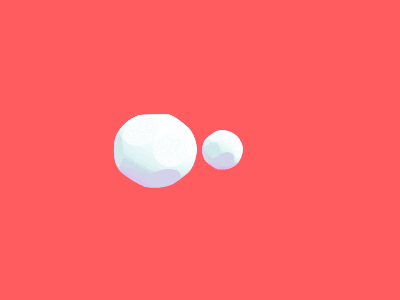
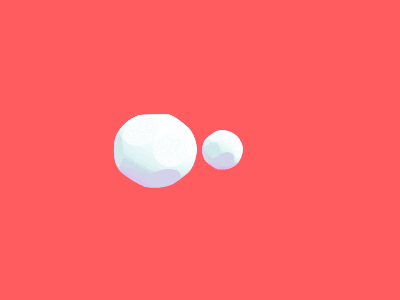
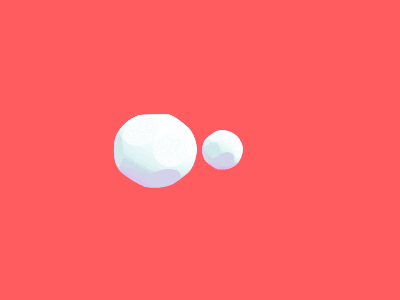
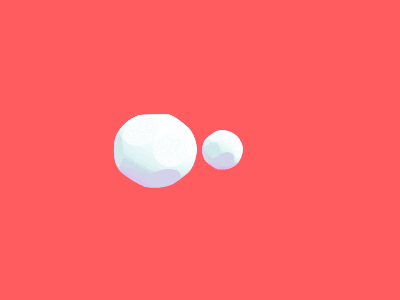
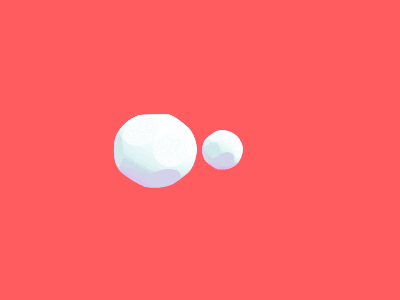
AUTODIFF: Autoregressive Diffusion Modeling for Structure-based Drug Design
Authors:Xinze Li, Penglei Wang, Tianfan Fu, Wenhao Gao, Chengtao Li, Leilei Shi, Junhong Liu
Structure-based drug design (SBDD), which aims to generate molecules that can bind tightly to the target protein, is an essential problem in drug discovery, and previous approaches have achieved initial success. However, most existing methods still suffer from invalid local structure or unrealistic conformation issues, which are mainly due to the poor leaning of bond angles or torsional angles. To alleviate these problems, we propose AUTODIFF, a diffusion-based fragment-wise autoregressive generation model. Specifically, we design a novel molecule assembly strategy named conformal motif that preserves the conformation of local structures of molecules first, then we encode the interaction of the protein-ligand complex with an SE(3)-equivariant convolutional network and generate molecules motif-by-motif with diffusion modeling. In addition, we also improve the evaluation framework of SBDD by constraining the molecular weights of the generated molecules in the same range, together with some new metrics, which make the evaluation more fair and practical. Extensive experiments on CrossDocked2020 demonstrate that our approach outperforms the existing models in generating realistic molecules with valid structures and conformations while maintaining high binding affinity.
PDF
点此查看论文截图
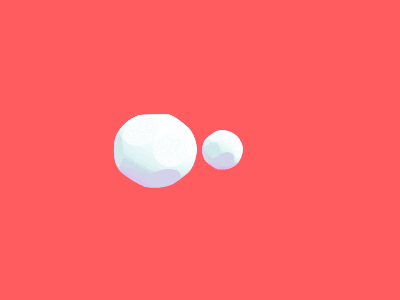
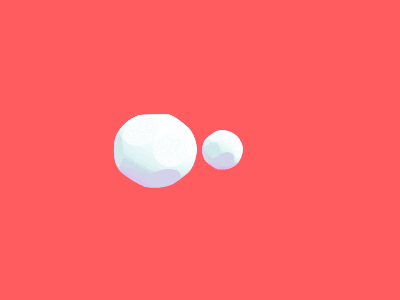
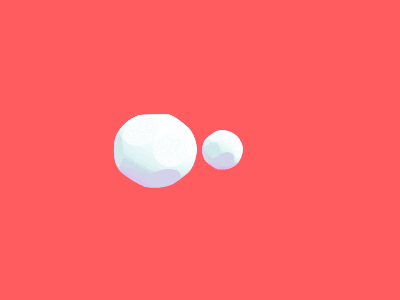
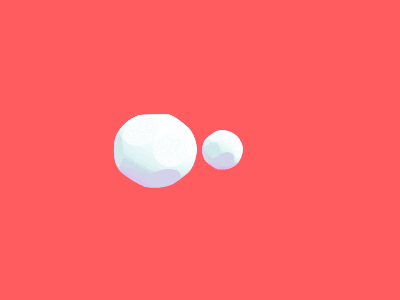