2023-11-25 更新
Label-Synchronous Neural Transducer for Adaptable Online E2E Speech Recognition
Authors:Keqi Deng, Philip C. Woodland
Although end-to-end (E2E) automatic speech recognition (ASR) has shown state-of-the-art recognition accuracy, it tends to be implicitly biased towards the training data distribution which can degrade generalisation. This paper proposes a label-synchronous neural transducer (LS-Transducer), which provides a natural approach to domain adaptation based on text-only data. The LS-Transducer extracts a label-level encoder representation before combining it with the prediction network output. Since blank tokens are no longer needed, the prediction network performs as a standard language model, which can be easily adapted using text-only data. An Auto-regressive Integrate-and-Fire (AIF) mechanism is proposed to generate the label-level encoder representation while retaining low latency operation that can be used for streaming. In addition, a streaming joint decoding method is designed to improve ASR accuracy while retaining synchronisation with AIF. Experiments show that compared to standard neural transducers, the proposed LS-Transducer gave a 12.9% relative WER reduction (WERR) for intra-domain LibriSpeech data, as well as 21.4% and 24.6% relative WERRs on cross-domain TED-LIUM 2 and AESRC2020 data with an adapted prediction network.
PDF This work has been submitted to the IEEE for possible publication. Copyright may be transferred without notice, after which this version may no longer be accessible
点此查看论文截图
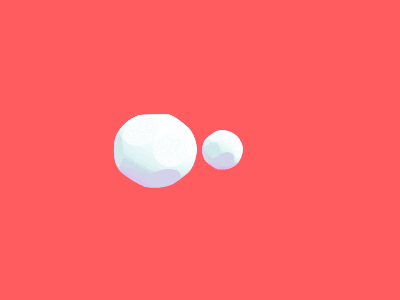
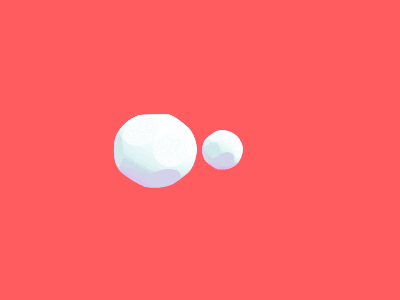
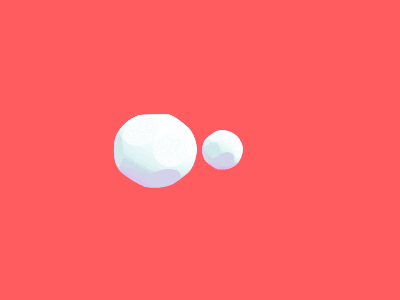
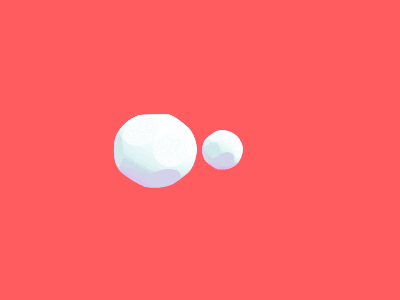
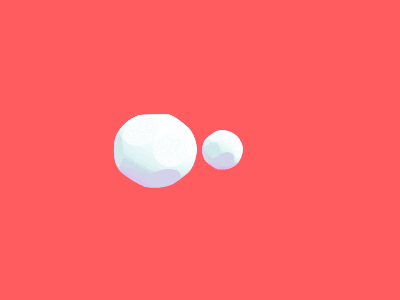
ML-LMCL: Mutual Learning and Large-Margin Contrastive Learning for Improving ASR Robustness in Spoken Language Understanding
Authors:Xuxin Cheng, Bowen Cao, Qichen Ye, Zhihong Zhu, Hongxiang Li, Yuexian Zou
Spoken language understanding (SLU) is a fundamental task in the task-oriented dialogue systems. However, the inevitable errors from automatic speech recognition (ASR) usually impair the understanding performance and lead to error propagation. Although there are some attempts to address this problem through contrastive learning, they (1) treat clean manual transcripts and ASR transcripts equally without discrimination in fine-tuning; (2) neglect the fact that the semantically similar pairs are still pushed away when applying contrastive learning; (3) suffer from the problem of Kullback-Leibler (KL) vanishing. In this paper, we propose Mutual Learning and Large-Margin Contrastive Learning (ML-LMCL), a novel framework for improving ASR robustness in SLU. Specifically, in fine-tuning, we apply mutual learning and train two SLU models on the manual transcripts and the ASR transcripts, respectively, aiming to iteratively share knowledge between these two models. We also introduce a distance polarization regularizer to avoid pushing away the intra-cluster pairs as much as possible. Moreover, we use a cyclical annealing schedule to mitigate KL vanishing issue. Experiments on three datasets show that ML-LMCL outperforms existing models and achieves new state-of-the-art performance.
PDF
点此查看论文截图
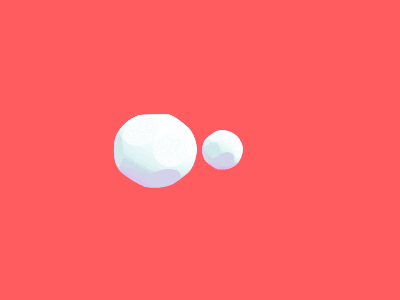
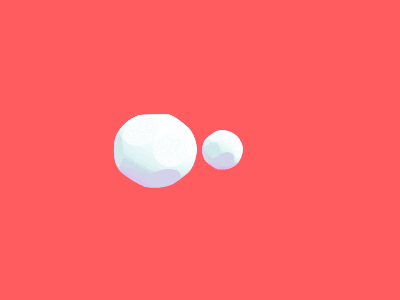
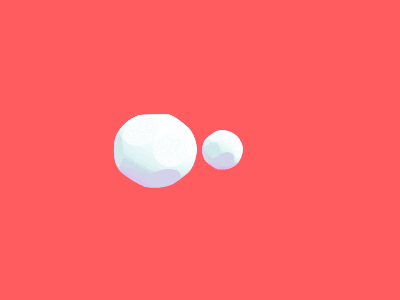
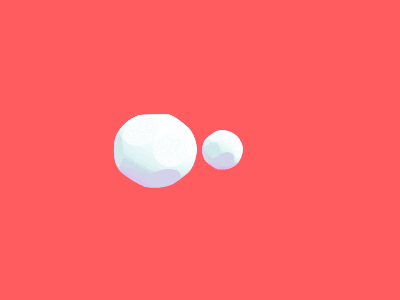
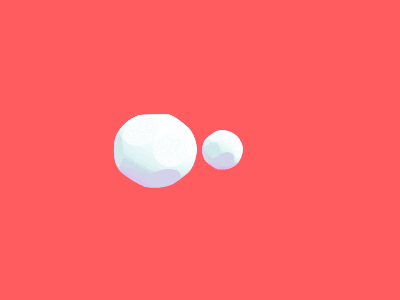
A recurrent connectionist model of melody perception : An exploration using TRACX2
Authors:Daniel Defays, Robert French, Barbara Tillmann
Are similar, or even identical, mechanisms used in the computational modeling of speech segmentation, serial image processing and music processing? We address this question by exploring how TRACX2, (French et al., 2011; French \& Cottrell, 2014; Mareschal \& French, 2017), a recognition-based, recursive connectionist autoencoder model of chunking and sequence segmentation, which has successfully simulated speech and serial-image processing, might be applied to elementary melody perception. The model, a three-layer autoencoder that recognizes ‘’chunks’’ of short sequences of intervals that have been frequently encountered on input, is trained on the tone intervals of melodically simple French children’s songs. It dynamically incorporates the internal representations of these chunks into new input. Its internal representations cluster in a manner that is consistent with ‘’human-recognizable’’ melodic categories. TRACX2 is sensitive to both contour and proximity information in the musical chunks that it encounters in its input. It shows the ‘’end-of-word’’ superiority effect demonstrated by Saffran et al. (1999) for short musical phrases. The overall findings suggest that the recursive autoassociative chunking mechanism, as implemented in TRACX2, may be a general segmentation and chunking mechanism, underlying not only word-and imagechunking, but also elementary melody processing.
PDF
点此查看论文截图
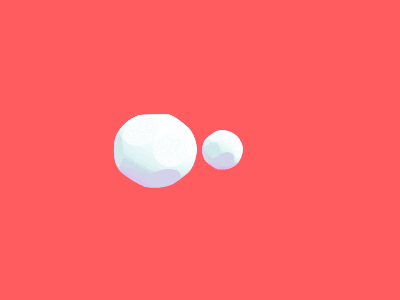
Analysis of Visual Features for Continuous Lipreading in Spanish
Authors:David Gimeno-Gómez, Carlos-D. Martínez-Hinarejos
During a conversation, our brain is responsible for combining information obtained from multiple senses in order to improve our ability to understand the message we are perceiving. Different studies have shown the importance of presenting visual information in these situations. Nevertheless, lipreading is a complex task whose objective is to interpret speech when audio is not available. By dispensing with a sense as crucial as hearing, it will be necessary to be aware of the challenge that this lack presents. In this paper, we propose an analysis of different speech visual features with the intention of identifying which of them is the best approach to capture the nature of lip movements for natural Spanish and, in this way, dealing with the automatic visual speech recognition task. In order to estimate our system, we present an audiovisual corpus compiled from a subset of the RTVE database, which has been used in the Albayz\’in evaluations. We employ a traditional system based on Hidden Markov Models with Gaussian Mixture Models. Results show that, although the task is difficult, in restricted conditions we obtain recognition results which determine that using eigenlips in combination with deep features is the best visual approach.
PDF Accepted in Proceedings of IberSpeech 2020 ( https://www.isca-speech.org/archive/iberspeech_2021/gimenogomez21_iberspeech.html )
点此查看论文截图
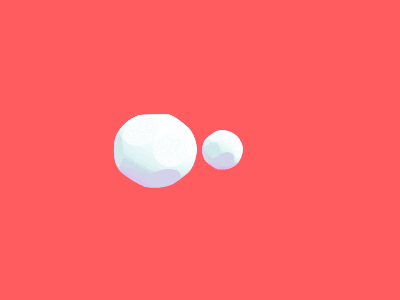
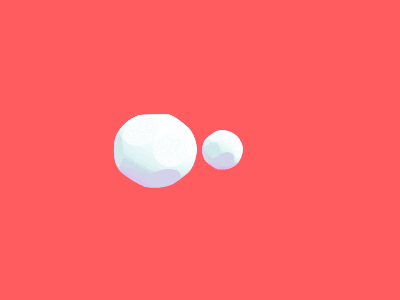
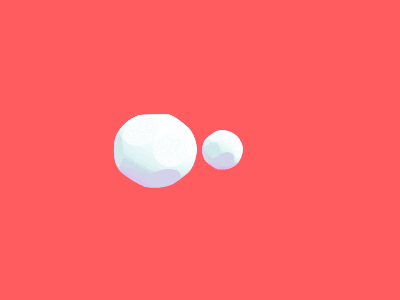
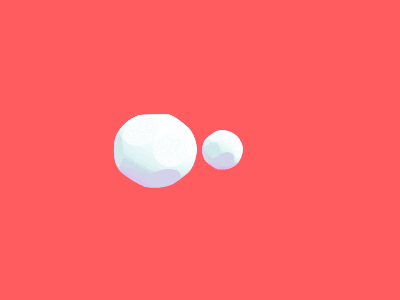
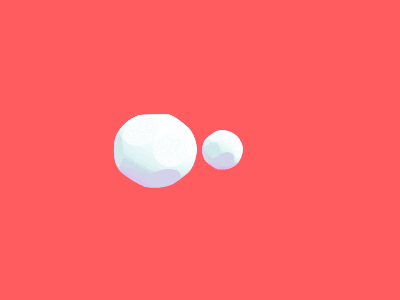
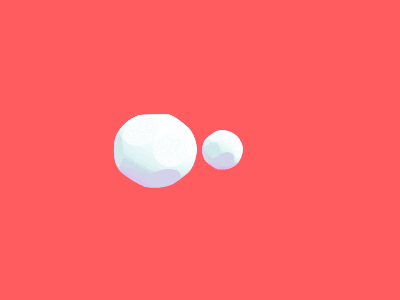
Speaker-Adapted End-to-End Visual Speech Recognition for Continuous Spanish
Authors:David Gimeno-Gómez, Carlos-D. Martínez-Hinarejos
Different studies have shown the importance of visual cues throughout the speech perception process. In fact, the development of audiovisual approaches has led to advances in the field of speech technologies. However, although noticeable results have recently been achieved, visual speech recognition remains an open research problem. It is a task in which, by dispensing with the auditory sense, challenges such as visual ambiguities and the complexity of modeling silence must be faced. Nonetheless, some of these challenges can be alleviated when the problem is approached from a speaker-dependent perspective. Thus, this paper studies, using the Spanish LIP-RTVE database, how the estimation of specialized end-to-end systems for a specific person could affect the quality of speech recognition. First, different adaptation strategies based on the fine-tuning technique were proposed. Then, a pre-trained CTC/Attention architecture was used as a baseline throughout our experiments. Our findings showed that a two-step fine-tuning process, where the VSR system is first adapted to the task domain, provided significant improvements when the speaker adaptation was addressed. Furthermore, results comparable to the current state of the art were reached even when only a limited amount of data was available.
PDF Accepted in Proceedings of IberSpeech 2022 ( https://www.isca-speech.org/archive/iberspeech_2022/gimenogomez22_iberspeech.html )
点此查看论文截图
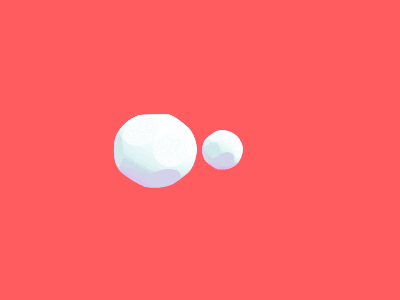
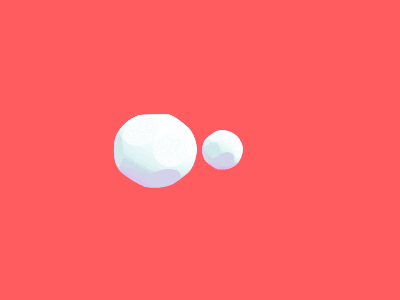
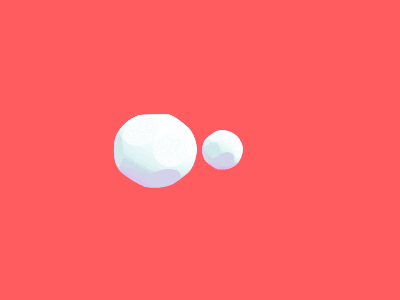
Investigating Weight-Perturbed Deep Neural Networks With Application in Iris Presentation Attack Detection
Authors:Renu Sharma, Redwan Sony, Arun Ross
Deep neural networks (DNNs) exhibit superior performance in various machine learning tasks, e.g., image classification, speech recognition, biometric recognition, object detection, etc. However, it is essential to analyze their sensitivity to parameter perturbations before deploying them in real-world applications. In this work, we assess the sensitivity of DNNs against perturbations to their weight and bias parameters. The sensitivity analysis involves three DNN architectures (VGG, ResNet, and DenseNet), three types of parameter perturbations (Gaussian noise, weight zeroing, and weight scaling), and two settings (entire network and layer-wise). We perform experiments in the context of iris presentation attack detection and evaluate on two publicly available datasets: LivDet-Iris-2017 and LivDet-Iris-2020. Based on the sensitivity analysis, we propose improved models simply by perturbing parameters of the network without undergoing training. We further combine these perturbed models at the score-level and at the parameter-level to improve the performance over the original model. The ensemble at the parameter-level shows an average improvement of 43.58% on the LivDet-Iris-2017 dataset and 9.25% on the LivDet-Iris-2020 dataset. The source code is available at https://github.com/redwankarimsony/WeightPerturbation-MSU.
PDF
点此查看论文截图
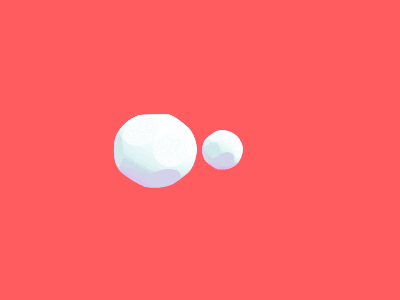
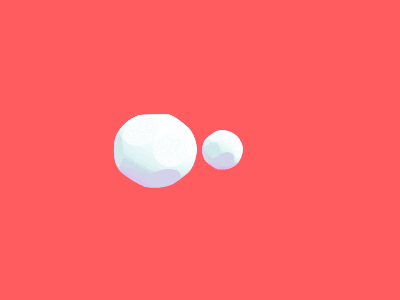
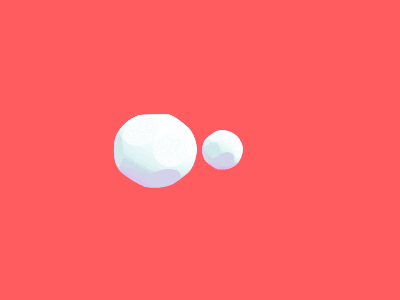
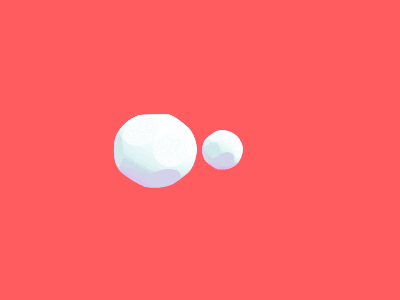
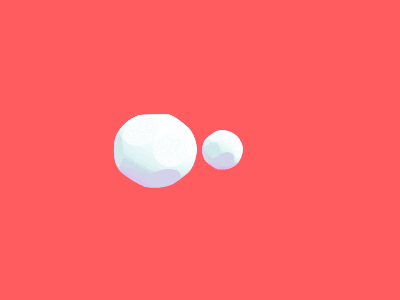
Sparsity-Driven EEG Channel Selection for Brain-Assisted Speech Enhancement
Authors:Jie Zhang, Qing-Tian Xu, Zhen-Hua Ling
Speech enhancement is widely used as a front-end to improve the speech quality in many audio systems, while it is still hard to extract the target speech in multi-talker conditions without prior information on the speaker identity. It was shown by auditory attention decoding that the attended speaker can be revealed by the electroencephalogram (EEG) of the listener implicitly. In this work, we therefore propose a novel end-to-end brain-assisted speech enhancement network (BASEN), which incorporates the listeners’ EEG signals and adopts a temporal convolutional network together with a convolutional multi-layer cross attention module to fuse EEG-audio features. Considering that an EEG cap with sparse channels exhibits multiple benefits and in practice many electrodes might contribute marginally, we further propose two channel selection methods, called residual Gumbel selection and convolutional regularization selection. They are dedicated to tackling the issues of training instability and duplicated channel selections, respectively. Experimental results on a public dataset show the superiority of the proposed baseline BASEN over existing approaches. The proposed channel selection methods can significantly reduce the amount of informative EEG channels with a negligible impact on the performance.
PDF arXiv admin note: text overlap with arXiv:2305.09994
点此查看论文截图
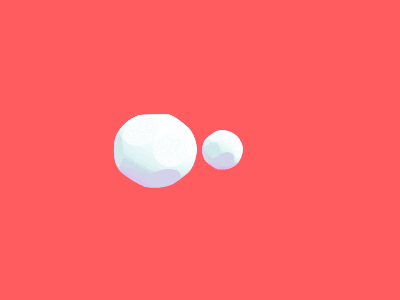
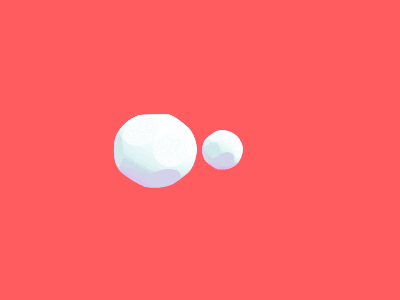
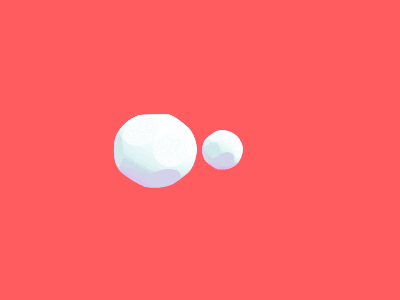
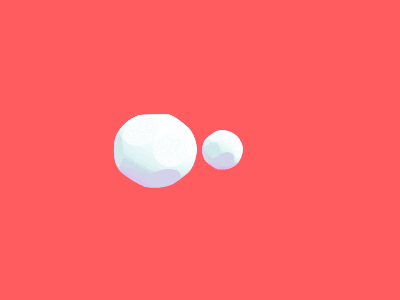