2023-10-05 更新
Accurate and lightweight dehazing via multi-receptive-field non-local network and novel contrastive regularization
Authors:Zewei He, Zixuan Chen, Ziqian Lu, Xuecheng Sun, Zhe-Ming Lu
Recently, deep learning-based methods have dominated image dehazing domain. Although very competitive dehazing performance has been achieved with sophisticated models, effective solutions for extracting useful features are still under-explored. In addition, non-local network, which has made a breakthrough in many vision tasks, has not been appropriately applied to image dehazing. Thus, a multi-receptive-field non-local network (MRFNLN) consisting of the multi-stream feature attention block (MSFAB) and cross non-local block (CNLB) is presented in this paper. We start with extracting richer features for dehazing. Specifically, we design a multi-stream feature extraction (MSFE) sub-block, which contains three parallel convolutions with different receptive fields (i.e., $1\times 1$, $3\times 3$, $5\times 5$) for extracting multi-scale features. Following MSFE, we employ an attention sub-block to make the model adaptively focus on important channels/regions. The MSFE and attention sub-blocks constitute our MSFAB. Then, we design a cross non-local block (CNLB), which can capture long-range dependencies beyond the query. Instead of the same input source of query branch, the key and value branches are enhanced by fusing more preceding features. CNLB is computation-friendly by leveraging a spatial pyramid down-sampling (SPDS) strategy to reduce the computation and memory consumption without sacrificing the performance. Last but not least, a novel detail-focused contrastive regularization (DFCR) is presented by emphasizing the low-level details and ignoring the high-level semantic information in the representation space. Comprehensive experimental results demonstrate that the proposed MRFNLN model outperforms recent state-of-the-art dehazing methods with less than 1.5 Million parameters.
PDF submitted to IEEE TCYB for possible publication
点此查看论文截图
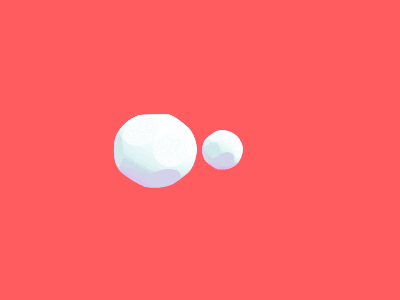
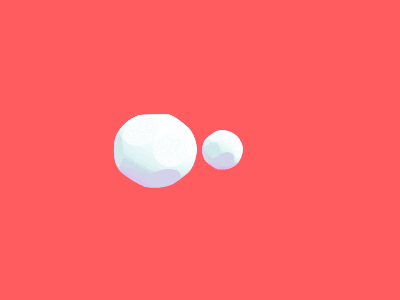
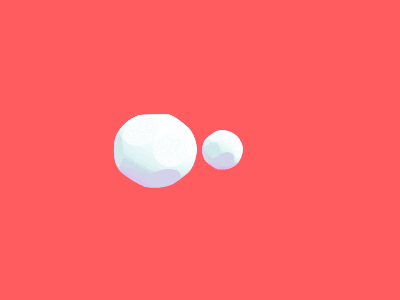
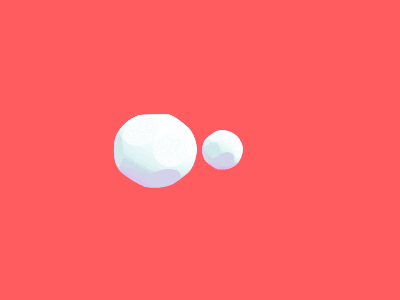
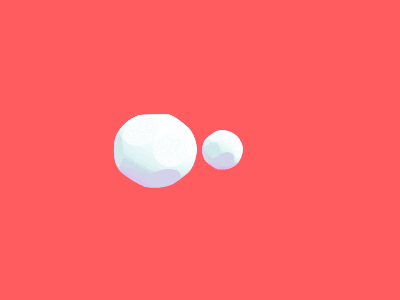
Understanding Masked Autoencoders From a Local Contrastive Perspective
Authors:Xiaoyu Yue, Lei Bai, Meng Wei, Jiangmiao Pang, Xihui Liu, Luping Zhou, Wanli Ouyang
Masked AutoEncoder(MAE) has revolutionized the field of self-supervised learning with its simple yet effective masking and reconstruction strategies. However, despite achieving state-of-the-art performance across various downstream vision tasks, the underlying mechanisms that drive MAE’s efficacy are less well-explored compared to the canonical contrastive learning paradigm. In this paper, we explore a new perspective to explain what truly contributes to the “rich hidden representations inside the MAE”. Firstly, concerning MAE’s generative pretraining pathway, with a unique encoder-decoder architecture to reconstruct images from aggressive masking, we conduct an in-depth analysis of the decoder’s behaviors. We empirically find that MAE’s decoder mainly learns local features with a limited receptive field, adhering to the well-known Locality Principle. Building upon this locality assumption, we propose a theoretical framework that reformulates the reconstruction-based MAE into a local region-level contrastive learning form for improved understanding. Furthermore, to substantiate the local contrastive nature of MAE, we introduce a Siamese architecture that combines the essence of MAE and contrastive learning without masking and explicit decoder, which sheds light on a unified and more flexible self-supervised learning framework.
PDF
点此查看论文截图
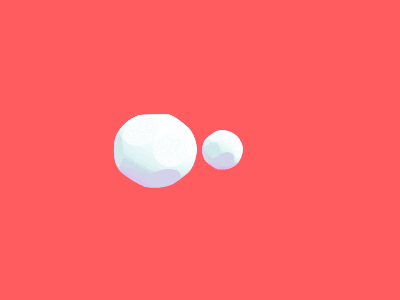
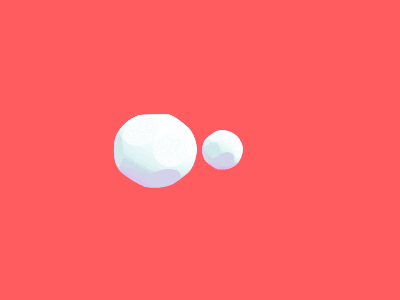
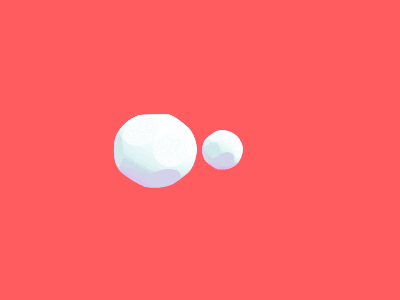
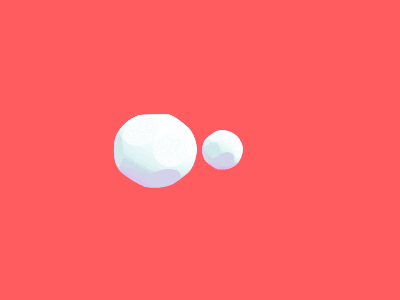
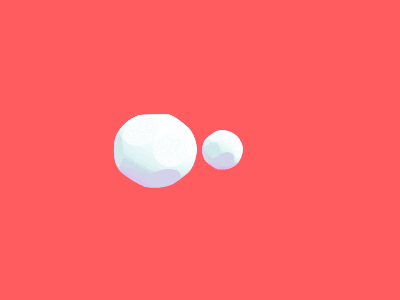
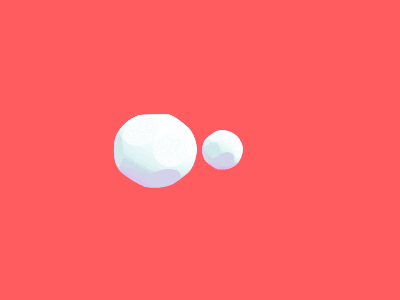