2023-10-05 更新
BiSinger: Bilingual Singing Voice Synthesis
Authors:Huali Zhou, Yueqian Lin, Yao Shi, Peng Sun, Ming Li
Although Singing Voice Synthesis (SVS) has made great strides with Text-to-Speech (TTS) techniques, multilingual singing voice modeling remains relatively unexplored. This paper presents BiSinger, a bilingual pop SVS system for English and Chinese Mandarin. Current systems require separate models per language and cannot accurately represent both Chinese and English, hindering code-switch SVS. To address this gap, we design a shared representation between Chinese and English singing voices, achieved by using the CMU dictionary with mapping rules. We fuse monolingual singing datasets with open-source singing voice conversion techniques to generate bilingual singing voices while also exploring the potential use of bilingual speech data. Experiments affirm that our language-independent representation and incorporation of related datasets enable a single model with enhanced performance in English and code-switch SVS while maintaining Chinese song performance. Audio samples are available at https://bisinger-svs.github.io.
PDF Accepted by ASRU2023
点此查看论文截图
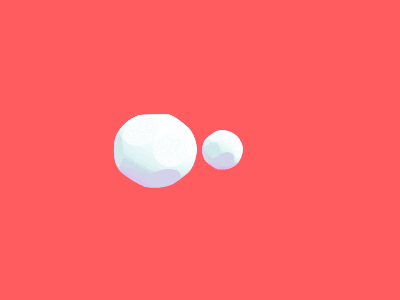
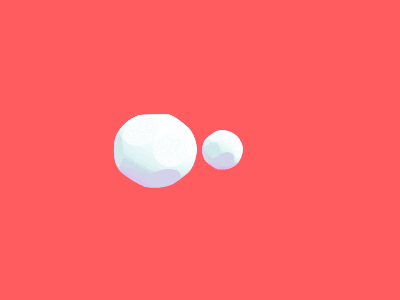
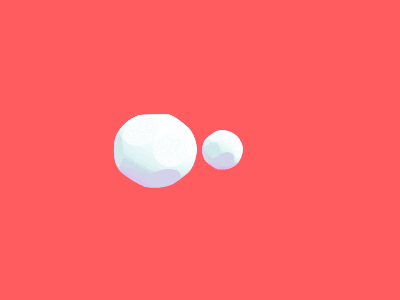
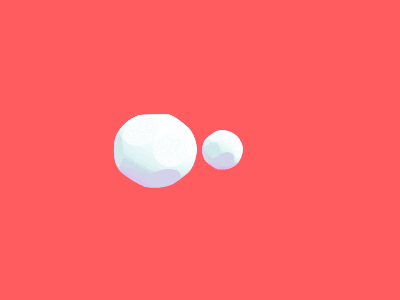
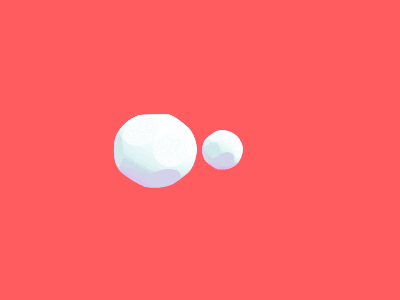
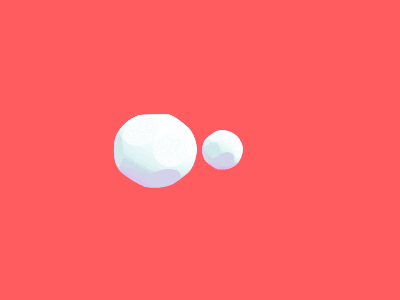
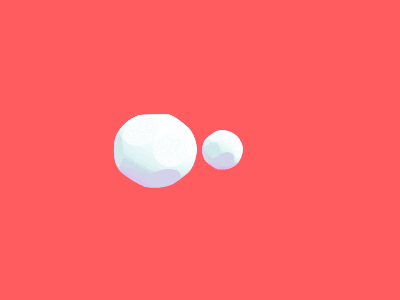
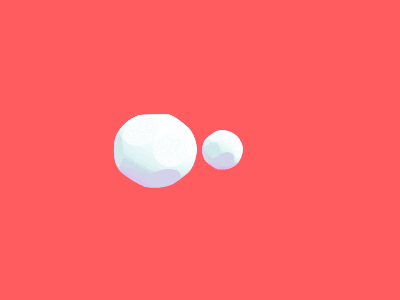
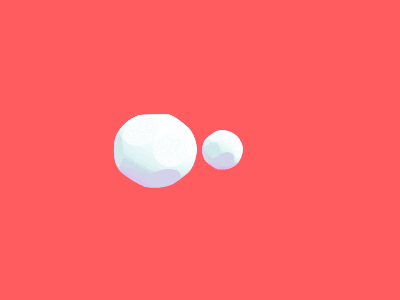
Unsupervised Pre-Training for Vietnamese Automatic Speech Recognition in the HYKIST Project
Authors:Khai Le-Duc
In today’s interconnected globe, moving abroad is more and more prevalent, whether it’s for employment, refugee resettlement, or other causes. Language difficulties between natives and immigrants present a common issue on a daily basis, especially in medical domain. This can make it difficult for patients and doctors to communicate during anamnesis or in the emergency room, which compromises patient care. The goal of the HYKIST Project is to develop a speech translation system to support patient-doctor communication with ASR and MT. ASR systems have recently displayed astounding performance on particular tasks for which enough quantities of training data are available, such as LibriSpeech. Building a good model is still difficult due to a variety of speaking styles, acoustic and recording settings, and a lack of in-domain training data. In this thesis, we describe our efforts to construct ASR systems for a conversational telephone speech recognition task in the medical domain for Vietnamese language to assist emergency room contact between doctors and patients across linguistic barriers. In order to enhance the system’s performance, we investigate various training schedules and data combining strategies. We also examine how best to make use of the little data that is available. The use of publicly accessible models like XLSR-53 is compared to the use of customized pre-trained models, and both supervised and unsupervised approaches are utilized using wav2vec 2.0 as architecture.
PDF Bachelor Thesis
点此查看论文截图
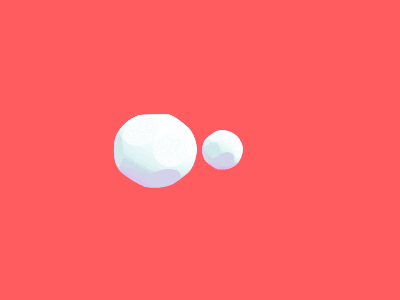
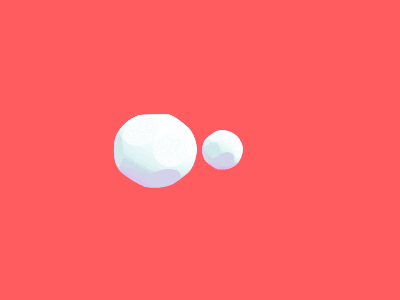
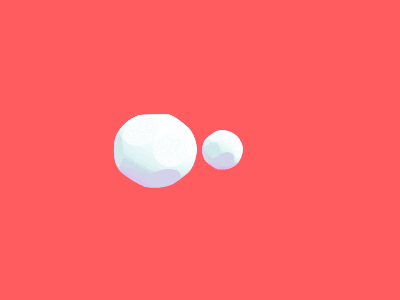
Meeting Recognition with Continuous Speech Separation and Transcription-Supported Diarization
Authors:Thilo von Neumann, Christoph Boeddeker, Tobias Cord-Landwehr, Marc Delcroix, Reinhold Haeb-Umbach
We propose a modular pipeline for the single-channel separation, recognition, and diarization of meeting-style recordings and evaluate it on the Libri-CSS dataset. Using a Continuous Speech Separation (CSS) system with a TF-GridNet separation architecture, followed by a speaker-agnostic speech recognizer, we achieve state-of-the-art recognition performance in terms of Optimal Reference Combination Word Error Rate (ORC WER). Then, a d-vector-based diarization module is employed to extract speaker embeddings from the enhanced signals and to assign the CSS outputs to the correct speaker. Here, we propose a syntactically informed diarization using sentence- and word-level boundaries of the ASR module to support speaker turn detection. This results in a state-of-the-art Concatenated minimum-Permutation Word Error Rate (cpWER) for the full meeting recognition pipeline.
PDF Submitted to ICASSP 2024
点此查看论文截图
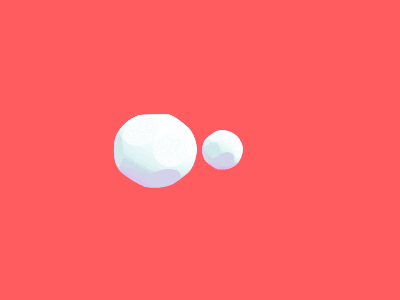
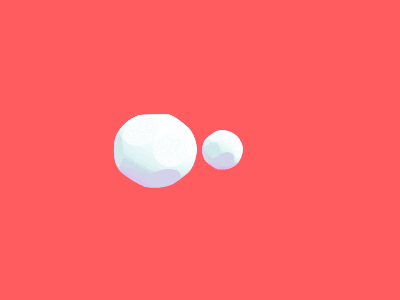
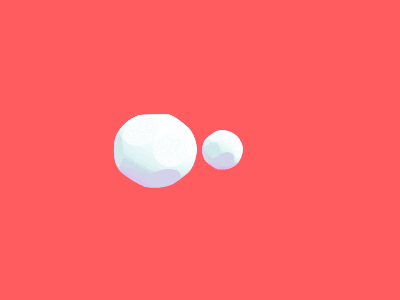
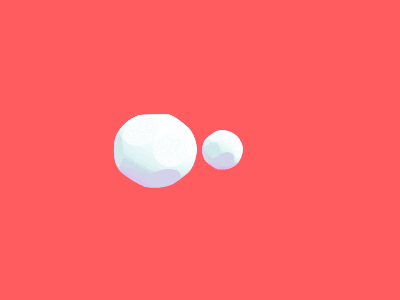
Low-Resource Self-Supervised Learning with SSL-Enhanced TTS
Authors:Po-chun Hsu, Ali Elkahky, Wei-Ning Hsu, Yossi Adi, Tu Anh Nguyen, Jade Copet, Emmanuel Dupoux, Hung-yi Lee, Abdelrahman Mohamed
Self-supervised learning (SSL) techniques have achieved remarkable results in various speech processing tasks. Nonetheless, a significant challenge remains in reducing the reliance on vast amounts of speech data for pre-training. This paper proposes to address this challenge by leveraging synthetic speech to augment a low-resource pre-training corpus. We construct a high-quality text-to-speech (TTS) system with limited resources using SSL features and generate a large synthetic corpus for pre-training. Experimental results demonstrate that our proposed approach effectively reduces the demand for speech data by 90\% with only slight performance degradation. To the best of our knowledge, this is the first work aiming to enhance low-resource self-supervised learning in speech processing.
PDF Preprint
点此查看论文截图
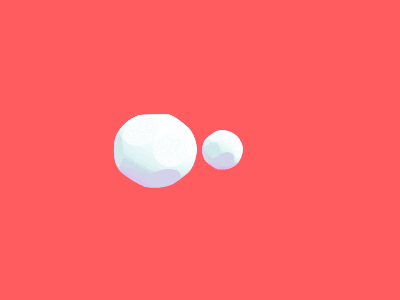
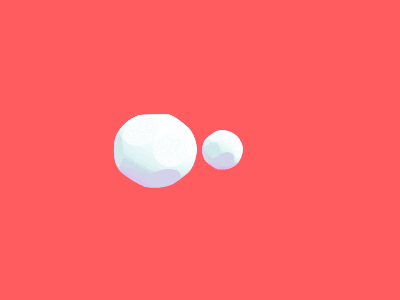
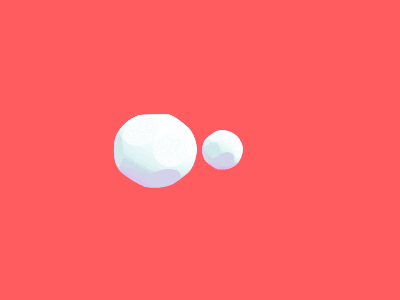
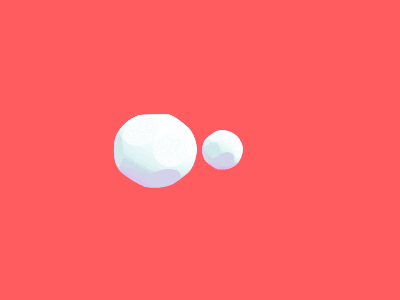
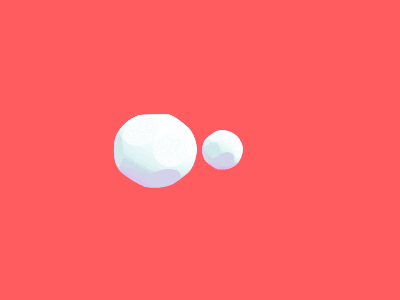
Toward Universal Speech Enhancement for Diverse Input Conditions
Authors:Wangyou Zhang, Kohei Saijo, Zhong-Qiu Wang, Shinji Watanabe, Yanmin Qian
The past decade has witnessed substantial growth of data-driven speech enhancement (SE) techniques thanks to deep learning. While existing approaches have shown impressive performance in some common datasets, most of them are designed only for a single condition (e.g., single-channel, multi-channel, or a fixed sampling frequency) or only consider a single task (e.g., denoising or dereverberation). Currently, there is no universal SE approach that can effectively handle diverse input conditions with a single model. In this paper, we make the first attempt to investigate this line of research. First, we devise a single SE model that is independent of microphone channels, signal lengths, and sampling frequencies. Second, we design a universal SE benchmark by combining existing public corpora with multiple conditions. Our experiments on a wide range of datasets show that the proposed single model can successfully handle diverse conditions with strong performance.
PDF 6 pages, 3 figures, 5 tables, accepted by ASRU 2023
点此查看论文截图
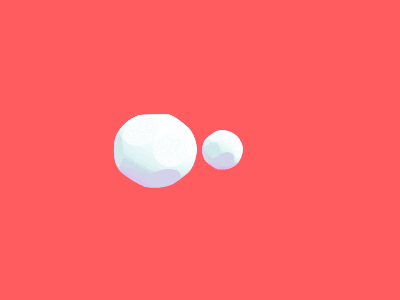
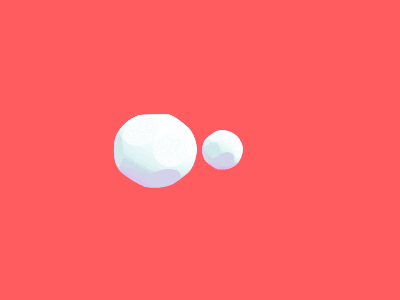
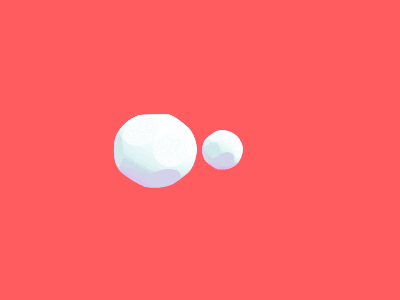
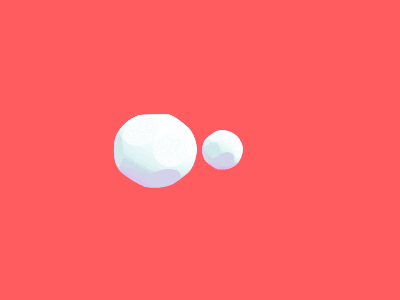
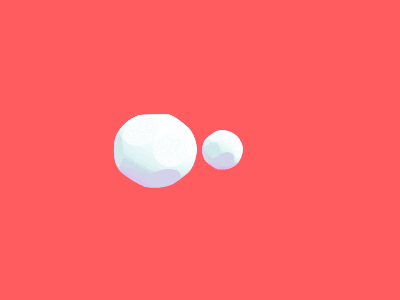
AV-CPL: Continuous Pseudo-Labeling for Audio-Visual Speech Recognition
Authors:Andrew Rouditchenko, Ronan Collobert, Tatiana Likhomanenko
Audio-visual speech contains synchronized audio and visual information that provides cross-modal supervision to learn representations for both automatic speech recognition (ASR) and visual speech recognition (VSR). We introduce continuous pseudo-labeling for audio-visual speech recognition (AV-CPL), a semi-supervised method to train an audio-visual speech recognition (AVSR) model on a combination of labeled and unlabeled videos with continuously regenerated pseudo-labels. Our models are trained for speech recognition from audio-visual inputs and can perform speech recognition using both audio and visual modalities, or only one modality. Our method uses the same audio-visual model for both supervised training and pseudo-label generation, mitigating the need for external speech recognition models to generate pseudo-labels. AV-CPL obtains significant improvements in VSR performance on the LRS3 dataset while maintaining practical ASR and AVSR performance. Finally, using visual-only speech data, our method is able to leverage unlabeled visual speech to improve VSR.
PDF Under review
点此查看论文截图
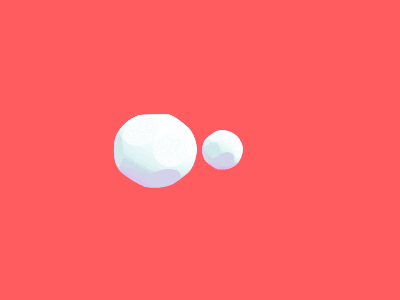
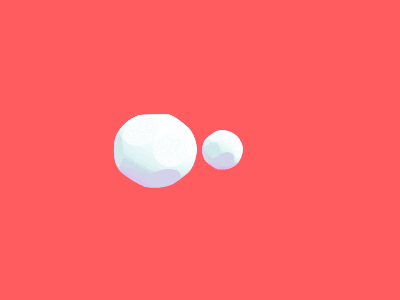
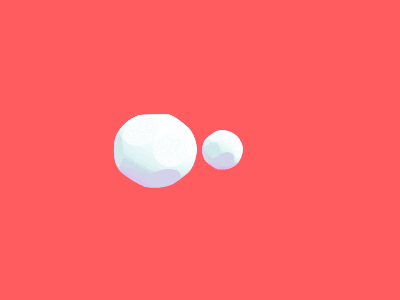
AfriSpeech-200: Pan-African Accented Speech Dataset for Clinical and General Domain ASR
Authors:Tobi Olatunji, Tejumade Afonja, Aditya Yadavalli, Chris Chinenye Emezue, Sahib Singh, Bonaventure F. P. Dossou, Joanne Osuchukwu, Salomey Osei, Atnafu Lambebo Tonja, Naome Etori, Clinton Mbataku
Africa has a very low doctor-to-patient ratio. At very busy clinics, doctors could see 30+ patients per day — a heavy patient burden compared with developed countries — but productivity tools such as clinical automatic speech recognition (ASR) are lacking for these overworked clinicians. However, clinical ASR is mature, even ubiquitous, in developed nations, and clinician-reported performance of commercial clinical ASR systems is generally satisfactory. Furthermore, the recent performance of general domain ASR is approaching human accuracy. However, several gaps exist. Several publications have highlighted racial bias with speech-to-text algorithms and performance on minority accents lags significantly. To our knowledge, there is no publicly available research or benchmark on accented African clinical ASR, and speech data is non-existent for the majority of African accents. We release AfriSpeech, 200hrs of Pan-African English speech, 67,577 clips from 2,463 unique speakers across 120 indigenous accents from 13 countries for clinical and general domain ASR, a benchmark test set, with publicly available pre-trained models with SOTA performance on the AfriSpeech benchmark.
PDF Accepted to TACL 2023. This is a pre-MIT Press publication version
点此查看论文截图
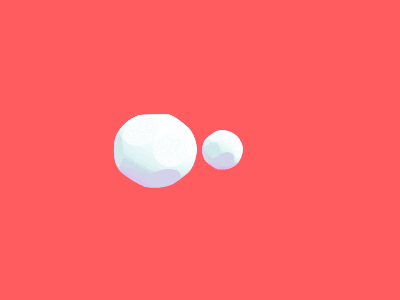
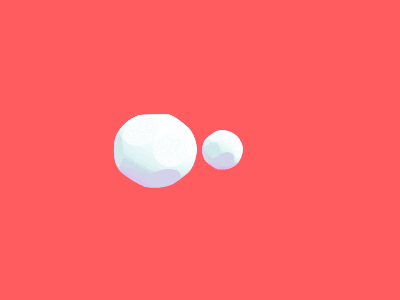
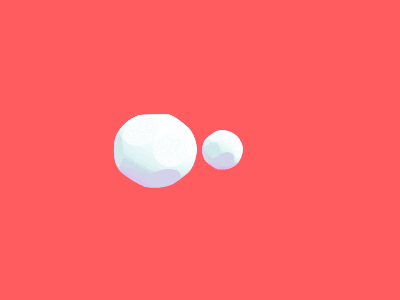
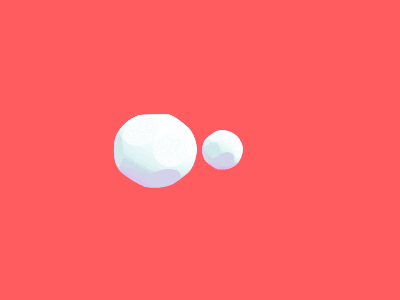
DiffPoseTalk: Speech-Driven Stylistic 3D Facial Animation and Head Pose Generation via Diffusion Models
Authors:Zhiyao Sun, Tian Lv, Sheng Ye, Matthieu Gaetan Lin, Jenny Sheng, Yu-Hui Wen, Minjing Yu, Yong-jin Liu
The generation of stylistic 3D facial animations driven by speech poses a significant challenge as it requires learning a many-to-many mapping between speech, style, and the corresponding natural facial motion. However, existing methods either employ a deterministic model for speech-to-motion mapping or encode the style using a one-hot encoding scheme. Notably, the one-hot encoding approach fails to capture the complexity of the style and thus limits generalization ability. In this paper, we propose DiffPoseTalk, a generative framework based on the diffusion model combined with a style encoder that extracts style embeddings from short reference videos. During inference, we employ classifier-free guidance to guide the generation process based on the speech and style. We extend this to include the generation of head poses, thereby enhancing user perception. Additionally, we address the shortage of scanned 3D talking face data by training our model on reconstructed 3DMM parameters from a high-quality, in-the-wild audio-visual dataset. Our extensive experiments and user study demonstrate that our approach outperforms state-of-the-art methods. The code and dataset will be made publicly available.
PDF Project page: https://raineggplant.github.io/DiffPoseTalk/
点此查看论文截图
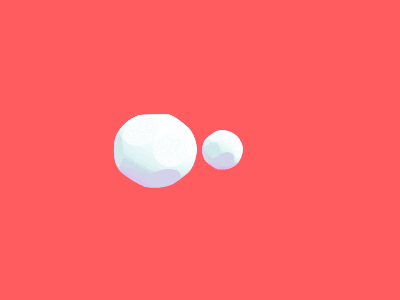
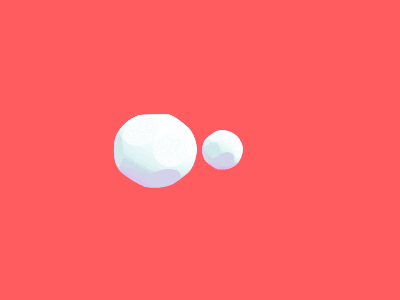
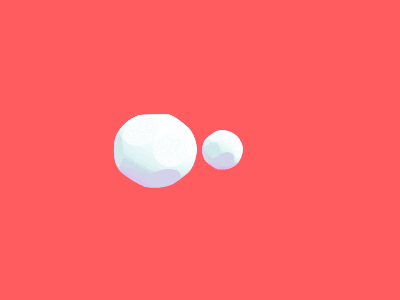
Harnessing Pre-Trained Sentence Transformers for Offensive Language Detection in Indian Languages
Authors:Ananya Joshi, Raviraj Joshi
In our increasingly interconnected digital world, social media platforms have emerged as powerful channels for the dissemination of hate speech and offensive content. This work delves into the domain of hate speech detection, placing specific emphasis on three low-resource Indian languages: Bengali, Assamese, and Gujarati. The challenge is framed as a text classification task, aimed at discerning whether a tweet contains offensive or non-offensive content. Leveraging the HASOC 2023 datasets, we fine-tuned pre-trained BERT and SBERT models to evaluate their effectiveness in identifying hate speech. Our findings underscore the superiority of monolingual sentence-BERT models, particularly in the Bengali language, where we achieved the highest ranking. However, the performance in Assamese and Gujarati languages signifies ongoing opportunities for enhancement. Our goal is to foster inclusive online spaces by countering hate speech proliferation.
PDF HASOC at FIRE 2023
点此查看论文截图
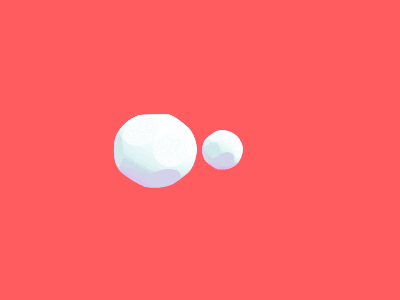
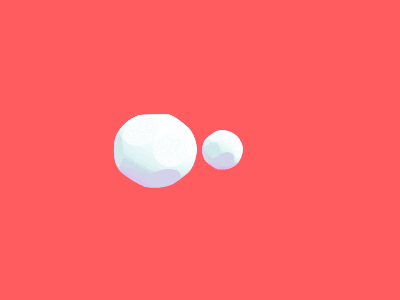
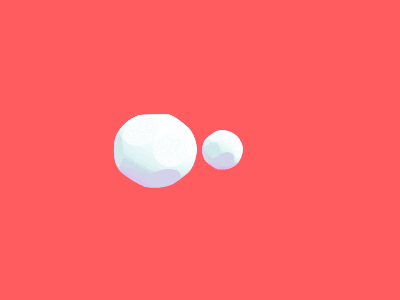
Unsupervised Speech Recognition with N-Skipgram and Positional Unigram Matching
Authors:Liming Wang, Mark Hasegawa-Johnson, Chang D. Yoo
Training unsupervised speech recognition systems presents challenges due to GAN-associated instability, misalignment between speech and text, and significant memory demands. To tackle these challenges, we introduce a novel ASR system, ESPUM. This system harnesses the power of lower-order N-skipgrams (up to N=3) combined with positional unigram statistics gathered from a small batch of samples. Evaluated on the TIMIT benchmark, our model showcases competitive performance in ASR and phoneme segmentation tasks. Access our publicly available code at https://github.com/lwang114/GraphUnsupASR.
PDF
点此查看论文截图
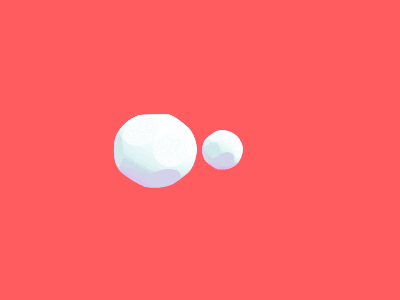
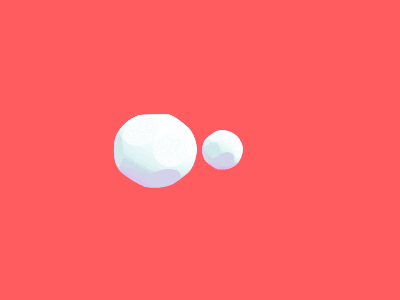
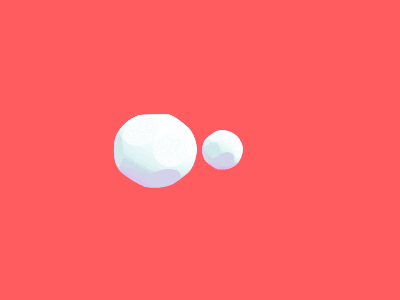
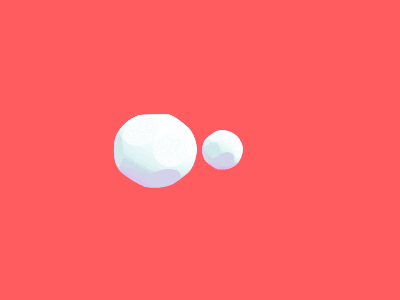