2023-08-26 更新
Target Speech Extraction with Conditional Diffusion Model
Authors:Naoyuki Kamo, Marc Delcroix, Tomohiro Nakatani
Diffusion model-based speech enhancement has received increased attention since it can generate very natural enhanced signals and generalizes well to unseen conditions. Diffusion models have been explored for several sub-tasks of speech enhancement, such as speech denoising, dereverberation, and source separation. In this paper, we investigate their use for target speech extraction (TSE), which consists of estimating the clean speech signal of a target speaker in a mixture of multi-talkers. TSE is realized by conditioning the extraction process on a clue identifying the target speaker. We show we can realize TSE using a conditional diffusion model conditioned on the clue. Besides, we introduce ensemble inference to reduce potential extraction errors caused by the diffusion process. In experiments on Libri2mix corpus, we show that the proposed diffusion model-based TSE combined with ensemble inference outperforms a comparable TSE system trained discriminatively.
PDF 5 pages, 4 figures, Interspeech2023
点此查看论文截图
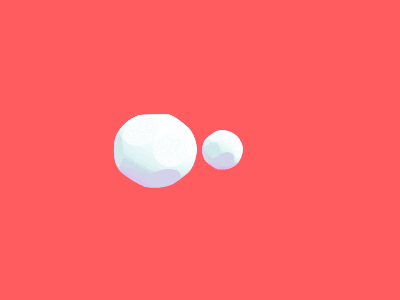
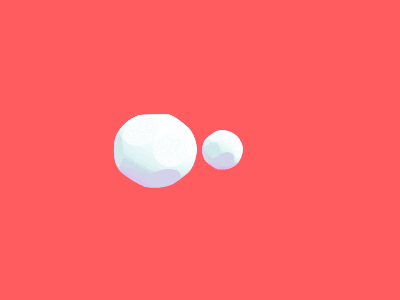
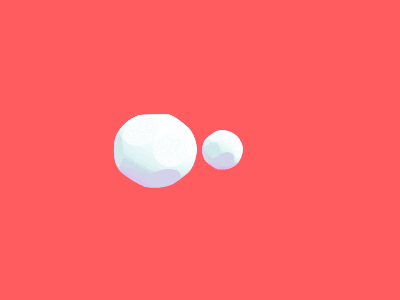
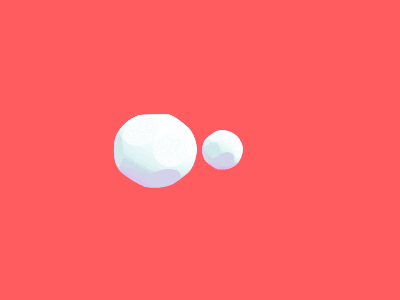
Separate Anything You Describe
Authors:Xubo Liu, Qiuqiang Kong, Yan Zhao, Haohe Liu, Yi Yuan, Yuzhuo Liu, Rui Xia, Yuxuan Wang, Mark D. Plumbley, Wenwu Wang
Language-queried audio source separation (LASS) is a new paradigm for computational auditory scene analysis (CASA). LASS aims to separate a target sound from an audio mixture given a natural language query, which provides a natural and scalable interface for digital audio applications. Recent works on LASS, despite attaining promising separation performance on specific sources (e.g., musical instruments, limited classes of audio events), are unable to separate audio concepts in the open domain. In this work, we introduce AudioSep, a foundation model for open-domain audio source separation with natural language queries. We train AudioSep on large-scale multimodal datasets and extensively evaluate its capabilities on numerous tasks including audio event separation, musical instrument separation, and speech enhancement. AudioSep demonstrates strong separation performance and impressive zero-shot generalization ability using audio captions or text labels as queries, substantially outperforming previous audio-queried and language-queried sound separation models. For reproducibility of this work, we will release the source code, evaluation benchmark and pre-trained model at: https://github.com/Audio-AGI/AudioSep.
PDF Project Page: https://audio-agi.github.io/Separate-Anything-You-Describe; Code: https://github.com/Audio-AGI/AudioSep
点此查看论文截图
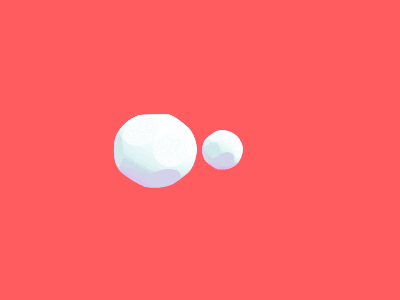
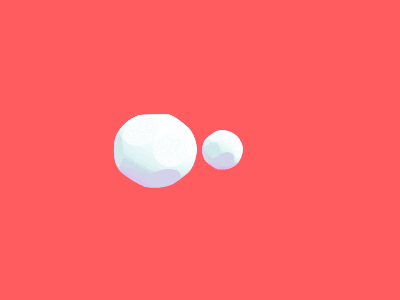
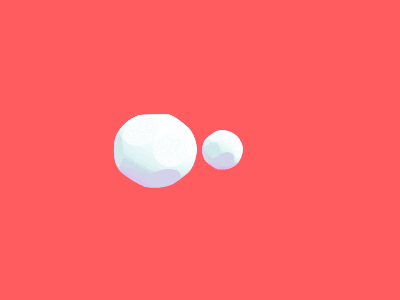
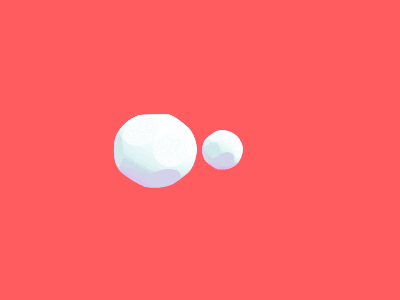
SpeechX: Neural Codec Language Model as a Versatile Speech Transformer
Authors:Xiaofei Wang, Manthan Thakker, Zhuo Chen, Naoyuki Kanda, Sefik Emre Eskimez, Sanyuan Chen, Min Tang, Shujie Liu, Jinyu Li, Takuya Yoshioka
Recent advancements in generative speech models based on audio-text prompts have enabled remarkable innovations like high-quality zero-shot text-to-speech. However, existing models still face limitations in handling diverse audio-text speech generation tasks involving transforming input speech and processing audio captured in adverse acoustic conditions. This paper introduces SpeechX, a versatile speech generation model capable of zero-shot TTS and various speech transformation tasks, dealing with both clean and noisy signals. SpeechX combines neural codec language modeling with multi-task learning using task-dependent prompting, enabling unified and extensible modeling and providing a consistent way for leveraging textual input in speech enhancement and transformation tasks. Experimental results show SpeechX’s efficacy in various tasks, including zero-shot TTS, noise suppression, target speaker extraction, speech removal, and speech editing with or without background noise, achieving comparable or superior performance to specialized models across tasks. See https://aka.ms/speechx for demo samples.
PDF See https://aka.ms/speechx for demo samples
点此查看论文截图
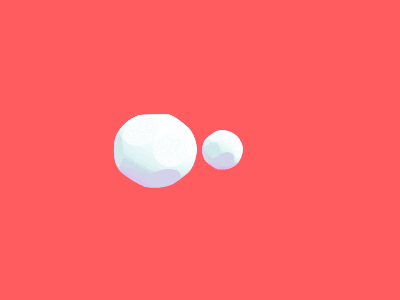
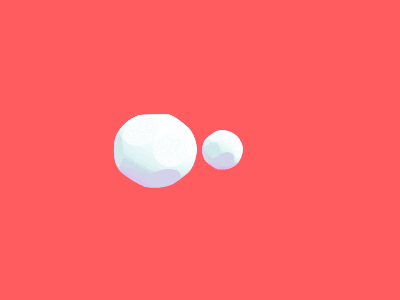
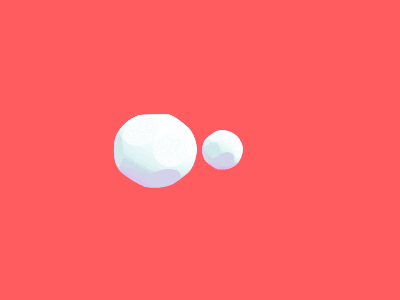
Integrating Emotion Recognition with Speech Recognition and Speaker Diarisation for Conversations
Authors:Wen Wu, Chao Zhang, Philip C. Woodland
Although automatic emotion recognition (AER) has recently drawn significant research interest, most current AER studies use manually segmented utterances, which are usually unavailable for dialogue systems. This paper proposes integrating AER with automatic speech recognition (ASR) and speaker diarisation (SD) in a jointly-trained system. Distinct output layers are built for four sub-tasks including AER, ASR, voice activity detection and speaker classification based on a shared encoder. Taking the audio of a conversation as input, the integrated system finds all speech segments and transcribes the corresponding emotion classes, word sequences, and speaker identities. Two metrics are proposed to evaluate AER performance with automatic segmentation based on time-weighted emotion and speaker classification errors. Results on the IEMOCAP dataset show that the proposed system consistently outperforms two baselines with separately trained single-task systems on AER, ASR and SD.
PDF Interspeech 2023
点此查看论文截图
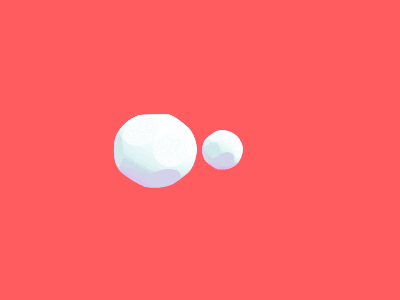
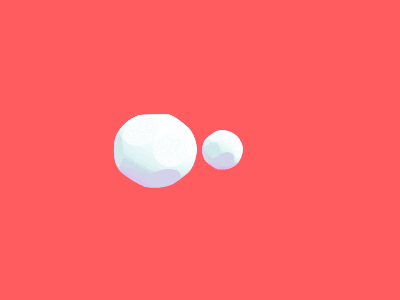
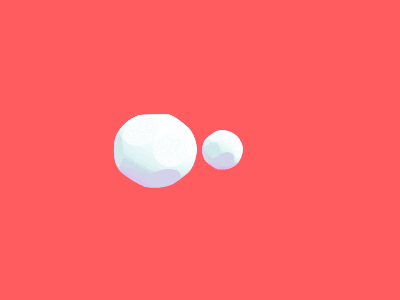
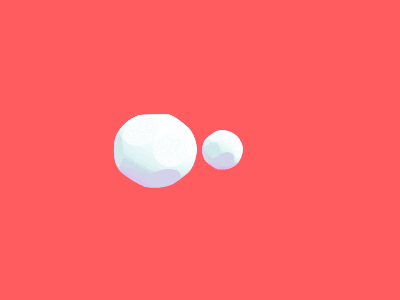
Evaluating Picture Description Speech for Dementia Detection using Image-text Alignment
Authors:Youxiang Zhu, Nana Lin, Xiaohui Liang, John A. Batsis, Robert M. Roth, Brian MacWhinney
Using picture description speech for dementia detection has been studied for 30 years. Despite the long history, previous models focus on identifying the differences in speech patterns between healthy subjects and patients with dementia but do not utilize the picture information directly. In this paper, we propose the first dementia detection models that take both the picture and the description texts as inputs and incorporate knowledge from large pre-trained image-text alignment models. We observe the difference between dementia and healthy samples in terms of the text’s relevance to the picture and the focused area of the picture. We thus consider such a difference could be used to enhance dementia detection accuracy. Specifically, we use the text’s relevance to the picture to rank and filter the sentences of the samples. We also identified focused areas of the picture as topics and categorized the sentences according to the focused areas. We propose three advanced models that pre-processed the samples based on their relevance to the picture, sub-image, and focused areas. The evaluation results show that our advanced models, with knowledge of the picture and large image-text alignment models, achieve state-of-the-art performance with the best detection accuracy at 83.44%, which is higher than the text-only baseline model at 79.91%. Lastly, we visualize the sample and picture results to explain the advantages of our models.
PDF
点此查看论文截图
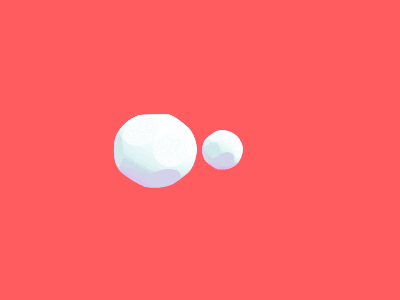
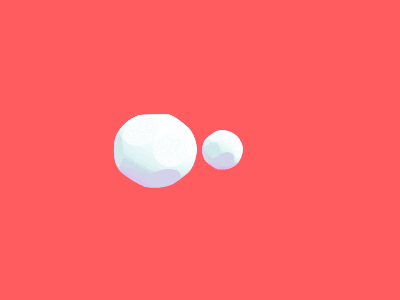
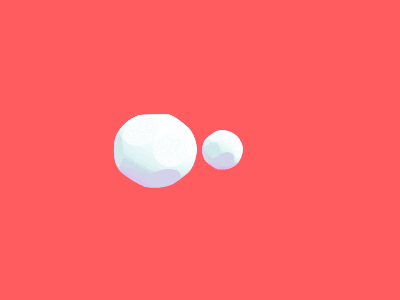
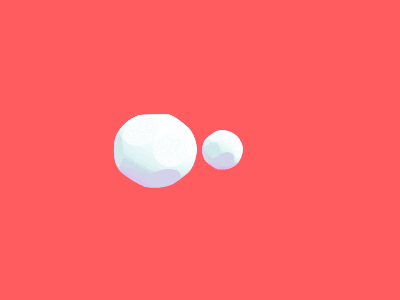
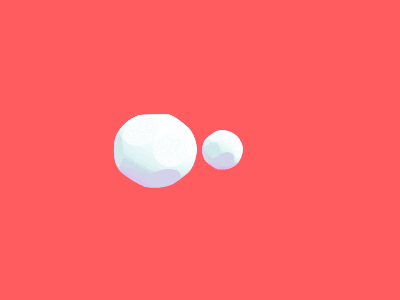
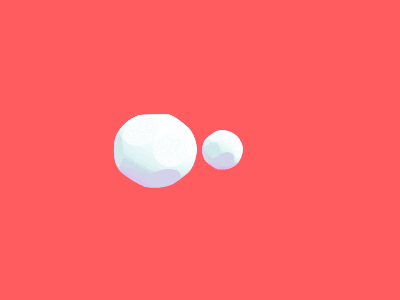
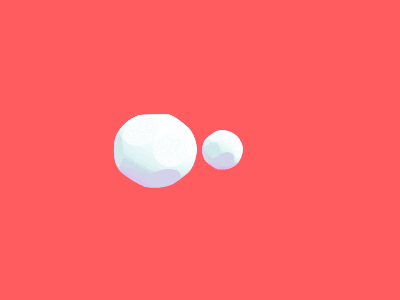
Decoding Emotions: A comprehensive Multilingual Study of Speech Models for Speech Emotion Recognition
Authors:Anant Singh, Akshat Gupta
Recent advancements in transformer-based speech representation models have greatly transformed speech processing. However, there has been limited research conducted on evaluating these models for speech emotion recognition (SER) across multiple languages and examining their internal representations. This article addresses these gaps by presenting a comprehensive benchmark for SER with eight speech representation models and six different languages. We conducted probing experiments to gain insights into inner workings of these models for SER. We find that using features from a single optimal layer of a speech model reduces the error rate by 32\% on average across seven datasets when compared to systems where features from all layers of speech models are used. We also achieve state-of-the-art results for German and Persian languages. Our probing results indicate that the middle layers of speech models capture the most important emotional information for speech emotion recognition.
PDF
点此查看论文截图
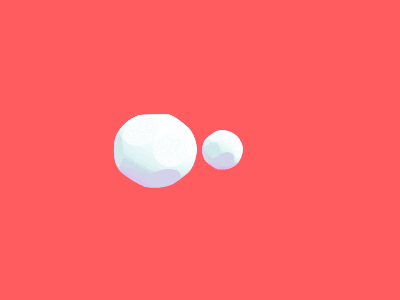
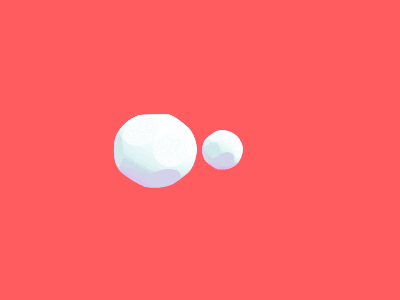
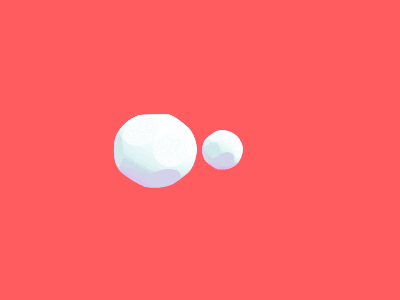
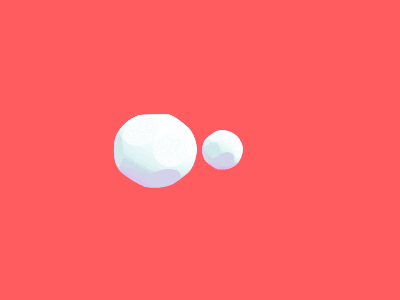
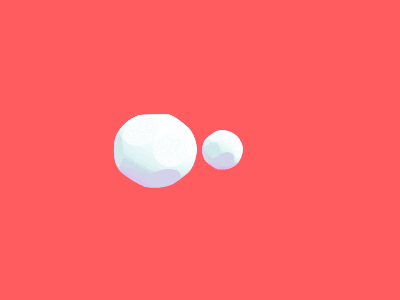
A Survey on Deep Multi-modal Learning for Body Language Recognition and Generation
Authors:Li Liu, Lufei Gao, Wentao Lei, Fengji Ma, Xiaotian Lin, Jinting Wang
Body language (BL) refers to the non-verbal communication expressed through physical movements, gestures, facial expressions, and postures. It is a form of communication that conveys information, emotions, attitudes, and intentions without the use of spoken or written words. It plays a crucial role in interpersonal interactions and can complement or even override verbal communication. Deep multi-modal learning techniques have shown promise in understanding and analyzing these diverse aspects of BL. The survey emphasizes their applications to BL generation and recognition. Several common BLs are considered i.e., Sign Language (SL), Cued Speech (CS), Co-speech (CoS), and Talking Head (TH), and we have conducted an analysis and established the connections among these four BL for the first time. Their generation and recognition often involve multi-modal approaches. Benchmark datasets for BL research are well collected and organized, along with the evaluation of SOTA methods on these datasets. The survey highlights challenges such as limited labeled data, multi-modal learning, and the need for domain adaptation to generalize models to unseen speakers or languages. Future research directions are presented, including exploring self-supervised learning techniques, integrating contextual information from other modalities, and exploiting large-scale pre-trained multi-modal models. In summary, this survey paper provides a comprehensive understanding of deep multi-modal learning for various BL generations and recognitions for the first time. By analyzing advancements, challenges, and future directions, it serves as a valuable resource for researchers and practitioners in advancing this field. n addition, we maintain a continuously updated paper list for deep multi-modal learning for BL recognition and generation: https://github.com/wentaoL86/awesome-body-language.
PDF
点此查看论文截图
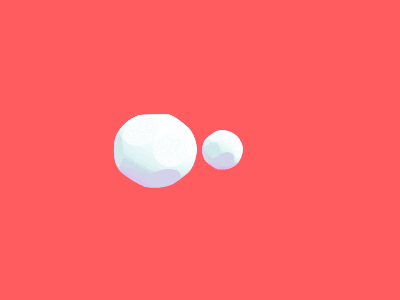
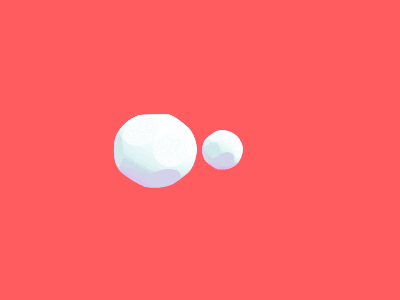
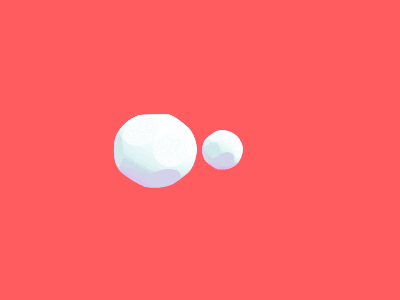
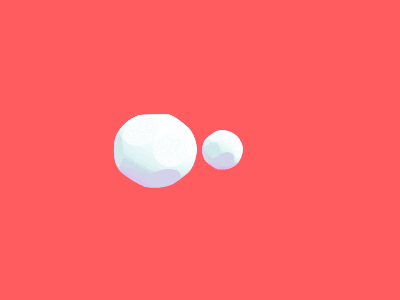
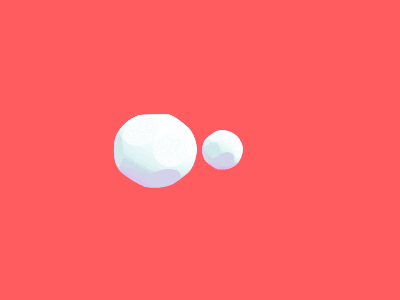
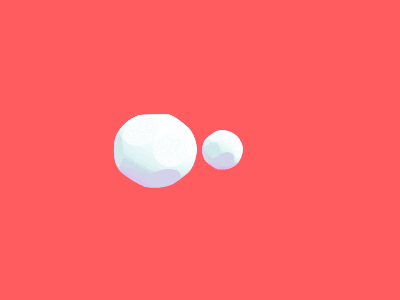
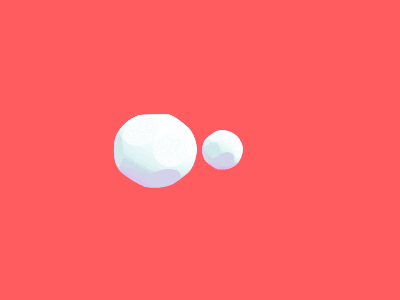
Explicit Estimation of Magnitude and Phase Spectra in Parallel for High-Quality Speech Enhancement
Authors:Ye-Xin Lu, Yang Ai, Zhen-Hua Ling
Phase information has a significant impact on speech perceptual quality and intelligibility. However, existing speech enhancement methods encounter limitations in explicit phase estimation due to the non-structural nature and wrapping characteristics of the phase, leading to a bottleneck in enhanced speech quality. To overcome the above issue, in this paper, we proposed MP-SENet, a novel Speech Enhancement Network which explicitly enhances Magnitude and Phase spectra in parallel. The proposed MP-SENet adopts a codec architecture in which the encoder and decoder are bridged by time-frequency Transformers along both time and frequency dimensions. The encoder aims to encode time-frequency representations derived from the input distorted magnitude and phase spectra. The decoder comprises dual-stream magnitude and phase decoders, directly enhancing magnitude and wrapped phase spectra by incorporating a magnitude estimation architecture and a phase parallel estimation architecture, respectively. To train the MP-SENet model effectively, we define multi-level loss functions, including mean square error and perceptual metric loss of magnitude spectra, anti-wrapping loss of phase spectra, as well as mean square error and consistency loss of short-time complex spectra. Experimental results demonstrate that our proposed MP-SENet excels in high-quality speech enhancement across multiple tasks, including speech denoising, dereverberation, and bandwidth extension. Compared to existing phase-aware speech enhancement methods, it successfully avoids the bidirectional compensation effect between the magnitude and phase, leading to a better harmonic restoration. Notably, for the speech denoising task, the MP-SENet yields a state-of-the-art performance with a PESQ of 3.60 on the public VoiceBank+DEMAND dataset.
PDF Submmited to IEEE Transactions on Audio, Speech and Language Processing
点此查看论文截图
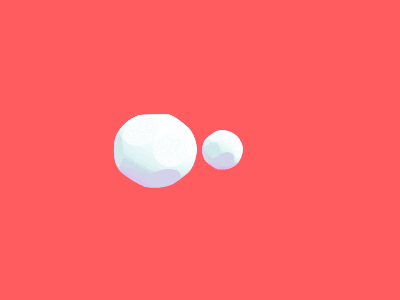
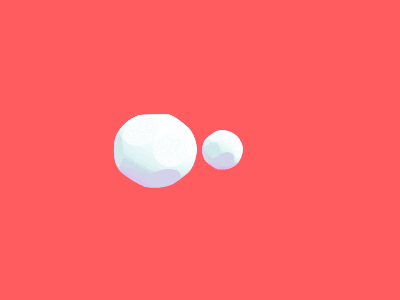
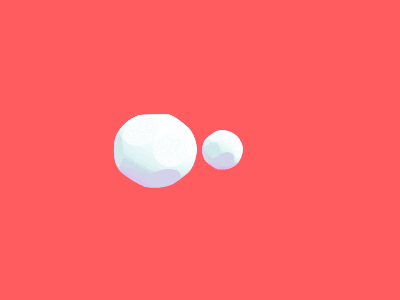
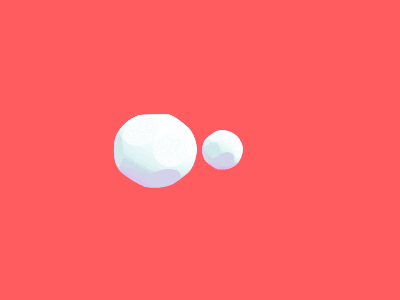
Bayes Risk Transducer: Transducer with Controllable Alignment Prediction
Authors:Jinchuan Tian, Jianwei Yu, Hangting Chen, Brian Yan, Chao Weng, Dong Yu, Shinji Watanabe
Automatic speech recognition (ASR) based on transducers is widely used. In training, a transducer maximizes the summed posteriors of all paths. The path with the highest posterior is commonly defined as the predicted alignment between the speech and the transcription. While the vanilla transducer does not have a prior preference for any of the valid paths, this work intends to enforce the preferred paths and achieve controllable alignment prediction. Specifically, this work proposes Bayes Risk Transducer (BRT), which uses a Bayes risk function to set lower risk values to the preferred paths so that the predicted alignment is more likely to satisfy specific desired properties. We further demonstrate that these predicted alignments with intentionally designed properties can provide practical advantages over the vanilla transducer. Experimentally, the proposed BRT saves inference cost by up to 46% for non-streaming ASR and reduces overall system latency by 41% for streaming ASR.
PDF
点此查看论文截图
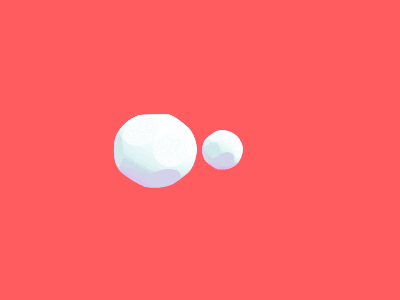
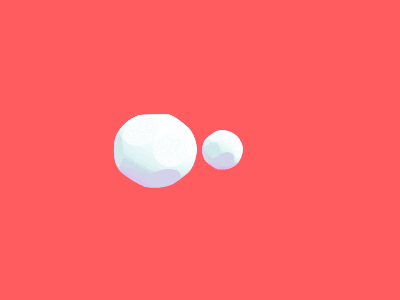
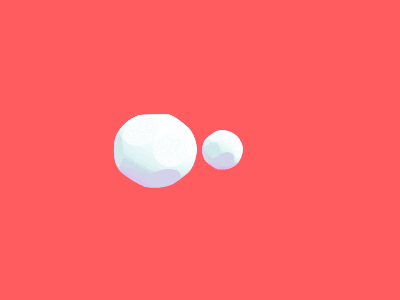
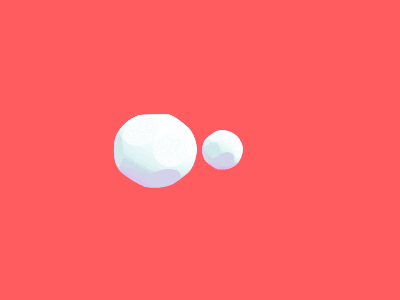
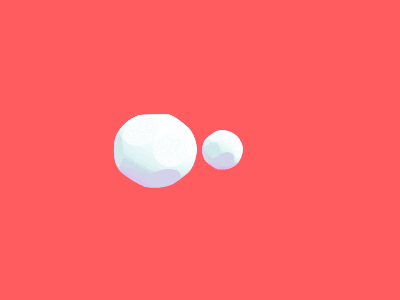
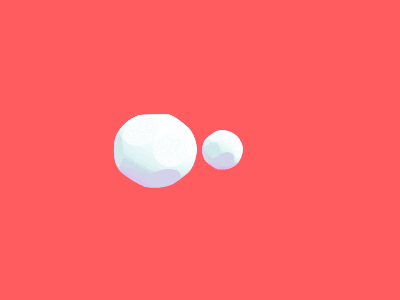
Improving Continuous Sign Language Recognition with Cross-Lingual Signs
Authors:Fangyun Wei, Yutong Chen
This work dedicates to continuous sign language recognition (CSLR), which is a weakly supervised task dealing with the recognition of continuous signs from videos, without any prior knowledge about the temporal boundaries between consecutive signs. Data scarcity heavily impedes the progress of CSLR. Existing approaches typically train CSLR models on a monolingual corpus, which is orders of magnitude smaller than that of speech recognition. In this work, we explore the feasibility of utilizing multilingual sign language corpora to facilitate monolingual CSLR. Our work is built upon the observation of cross-lingual signs, which originate from different sign languages but have similar visual signals (e.g., hand shape and motion). The underlying idea of our approach is to identify the cross-lingual signs in one sign language and properly leverage them as auxiliary training data to improve the recognition capability of another. To achieve the goal, we first build two sign language dictionaries containing isolated signs that appear in two datasets. Then we identify the sign-to-sign mappings between two sign languages via a well-optimized isolated sign language recognition model. At last, we train a CSLR model on the combination of the target data with original labels and the auxiliary data with mapped labels. Experimentally, our approach achieves state-of-the-art performance on two widely-used CSLR datasets: Phoenix-2014 and Phoenix-2014T.
PDF Accepted by ICCV 2023
点此查看论文截图
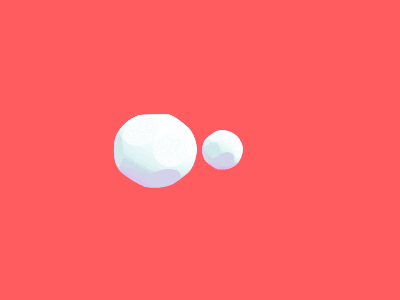
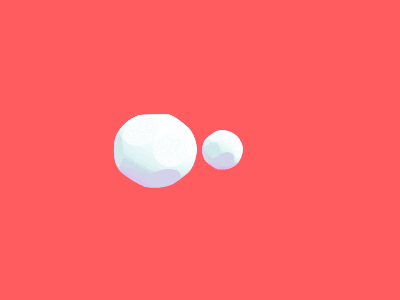
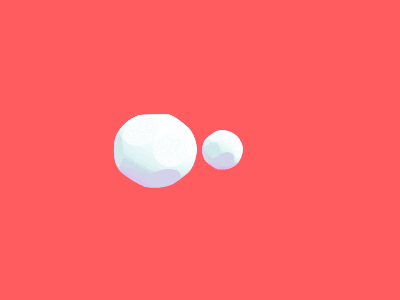
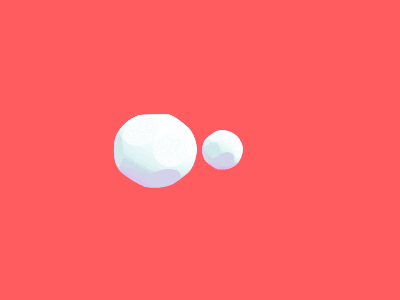
SeamlessM4T-Massively Multilingual & Multimodal Machine Translation
Authors:Seamless Communication, Loïc Barrault, Yu-An Chung, Mariano Cora Meglioli, David Dale, Ning Dong, Paul-Ambroise Duquenne, Hady Elsahar, Hongyu Gong, Kevin Heffernan, John Hoffman, Christopher Klaiber, Pengwei Li, Daniel Licht, Jean Maillard, Alice Rakotoarison, Kaushik Ram Sadagopan, Guillaume Wenzek, Ethan Ye, Bapi Akula, Peng-Jen Chen, Naji El Hachem, Brian Ellis, Gabriel Mejia Gonzalez, Justin Haaheim, Prangthip Hansanti, Russ Howes, Bernie Huang, Min-Jae Hwang, Hirofumi Inaguma, Somya Jain, Elahe Kalbassi, Amanda Kallet, Ilia Kulikov, Janice Lam, Daniel Li, Xutai Ma, Ruslan Mavlyutov, Benjamin Peloquin, Mohamed Ramadan, Abinesh Ramakrishnan, Anna Sun, Kevin Tran, Tuan Tran, Igor Tufanov, Vish Vogeti, Carleigh Wood, Yilin Yang, Bokai Yu, Pierre Andrews, Can Balioglu, Marta R. Costa-jussà, Onur Celebi, Maha Elbayad, Cynthia Gao, Francisco Guzmán, Justine Kao, Ann Lee, Alexandre Mourachko, Juan Pino, Sravya Popuri, Christophe Ropers, Safiyyah Saleem, Holger Schwenk, Paden Tomasello, Changhan Wang, Jeff Wang, Skyler Wang
What does it take to create the Babel Fish, a tool that can help individuals translate speech between any two languages? While recent breakthroughs in text-based models have pushed machine translation coverage beyond 200 languages, unified speech-to-speech translation models have yet to achieve similar strides. More specifically, conventional speech-to-speech translation systems rely on cascaded systems that perform translation progressively, putting high-performing unified systems out of reach. To address these gaps, we introduce SeamlessM4T, a single model that supports speech-to-speech translation, speech-to-text translation, text-to-speech translation, text-to-text translation, and automatic speech recognition for up to 100 languages. To build this, we used 1 million hours of open speech audio data to learn self-supervised speech representations with w2v-BERT 2.0. Subsequently, we created a multimodal corpus of automatically aligned speech translations. Filtered and combined with human-labeled and pseudo-labeled data, we developed the first multilingual system capable of translating from and into English for both speech and text. On FLEURS, SeamlessM4T sets a new standard for translations into multiple target languages, achieving an improvement of 20% BLEU over the previous SOTA in direct speech-to-text translation. Compared to strong cascaded models, SeamlessM4T improves the quality of into-English translation by 1.3 BLEU points in speech-to-text and by 2.6 ASR-BLEU points in speech-to-speech. Tested for robustness, our system performs better against background noises and speaker variations in speech-to-text tasks compared to the current SOTA model. Critically, we evaluated SeamlessM4T on gender bias and added toxicity to assess translation safety. Finally, all contributions in this work are open-sourced and accessible at https://github.com/facebookresearch/seamless_communication
PDF
点此查看论文截图
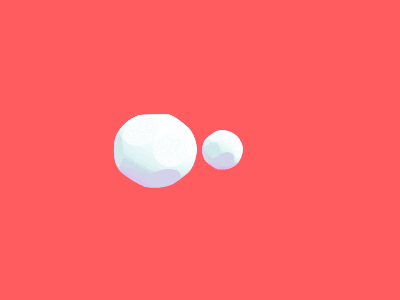
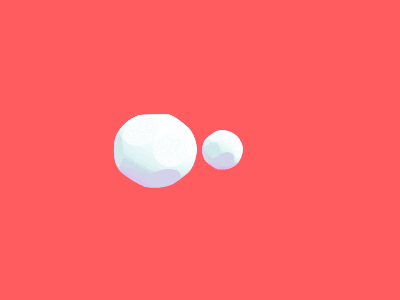
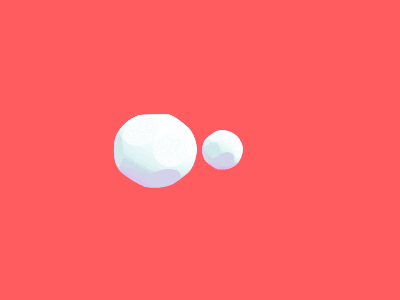
KinSPEAK: Improving speech recognition for Kinyarwanda via semi-supervised learning methods
Authors:Antoine Nzeyimana
Despite recent availability of large transcribed Kinyarwanda speech data, achieving robust speech recognition for Kinyarwanda is still challenging. In this work, we show that using self-supervised pre-training, following a simple curriculum schedule during fine-tuning and using semi-supervised learning to leverage large unlabelled speech data significantly improve speech recognition performance for Kinyarwanda. Our approach focuses on using public domain data only. A new studio-quality speech dataset is collected from a public website, then used to train a clean baseline model. The clean baseline model is then used to rank examples from a more diverse and noisy public dataset, defining a simple curriculum training schedule. Finally, we apply semi-supervised learning to label and learn from large unlabelled data in four successive generations. Our final model achieves 3.2% word error rate (WER) on the new dataset and 15.9% WER on Mozilla Common Voice benchmark, which is state-of-the-art to the best of our knowledge. Our experiments also indicate that using syllabic rather than character-based tokenization results in better speech recognition performance for Kinyarwanda.
PDF 9 pages, 2 figures, 5 tables
点此查看论文截图
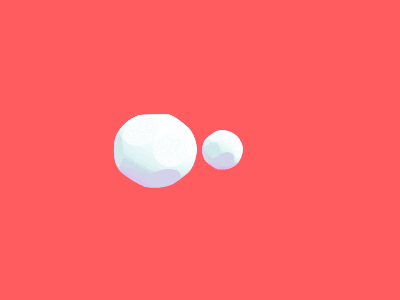
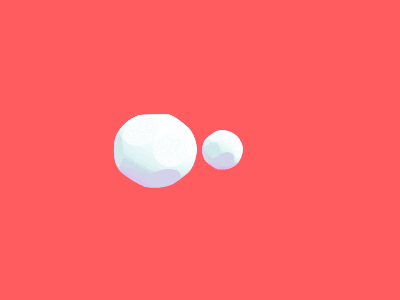
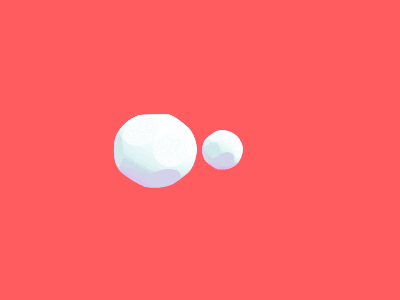
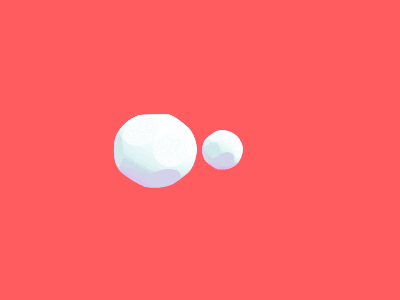
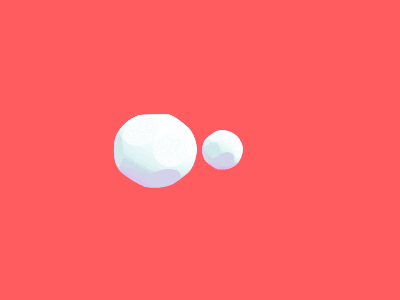
AdVerb: Visually Guided Audio Dereverberation
Authors:Sanjoy Chowdhury, Sreyan Ghosh, Subhrajyoti Dasgupta, Anton Ratnarajah, Utkarsh Tyagi, Dinesh Manocha
We present AdVerb, a novel audio-visual dereverberation framework that uses visual cues in addition to the reverberant sound to estimate clean audio. Although audio-only dereverberation is a well-studied problem, our approach incorporates the complementary visual modality to perform audio dereverberation. Given an image of the environment where the reverberated sound signal has been recorded, AdVerb employs a novel geometry-aware cross-modal transformer architecture that captures scene geometry and audio-visual cross-modal relationship to generate a complex ideal ratio mask, which, when applied to the reverberant audio predicts the clean sound. The effectiveness of our method is demonstrated through extensive quantitative and qualitative evaluations. Our approach significantly outperforms traditional audio-only and audio-visual baselines on three downstream tasks: speech enhancement, speech recognition, and speaker verification, with relative improvements in the range of 18% - 82% on the LibriSpeech test-clean set. We also achieve highly satisfactory RT60 error scores on the AVSpeech dataset.
PDF Accepted at ICCV 2023. For project page, see https://gamma.umd.edu/researchdirections/speech/adverb
点此查看论文截图
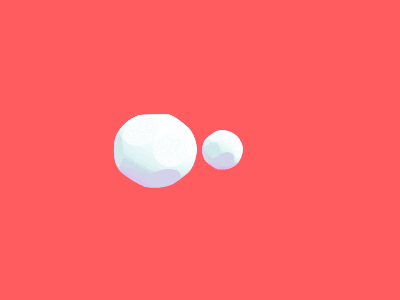
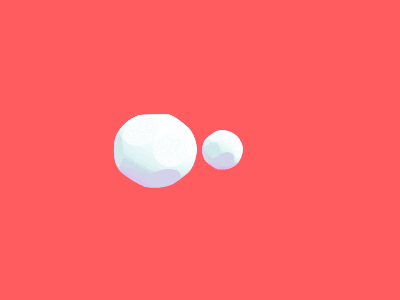
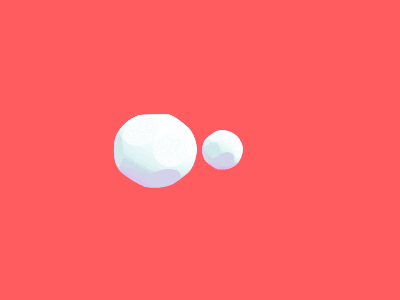
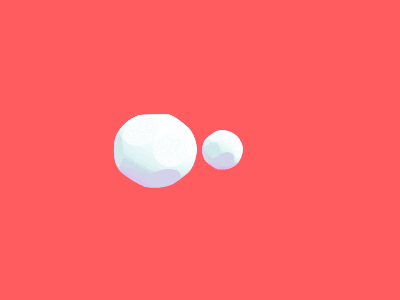
A Small and Fast BERT for Chinese Medical Punctuation Restoration
Authors:Tongtao Ling, Chen Liao, Zhipeng Yu, Lei Chen, Shilei Huang, Yi Liu
In clinical dictation, utterances after automatic speech recognition (ASR) without explicit punctuation marks may lead to the misunderstanding of dictated reports. To give a precise and understandable clinical report with ASR, automatic punctuation restoration is required. Considering a practical scenario, we propose a fast and light pre-trained model for Chinese medical punctuation restoration based on ‘pretraining and fine-tuning’ paradigm. In this work, we distill pre-trained models by incorporating supervised contrastive learning and a novel auxiliary pre-training task (Punctuation Mark Prediction) to make it well-suited for punctuation restoration. Our experiments on various distilled models reveal that our model can achieve 95% performance while 10% model size relative to state-of-the-art Chinese RoBERTa.
PDF 5 pages, 2 figures
点此查看论文截图
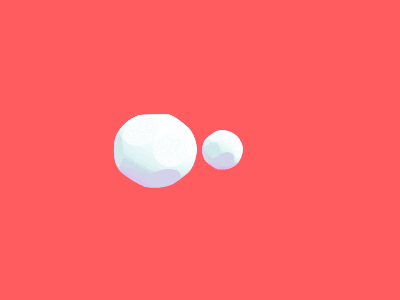
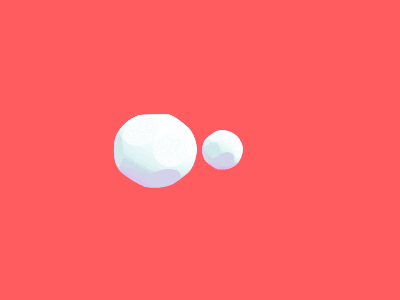
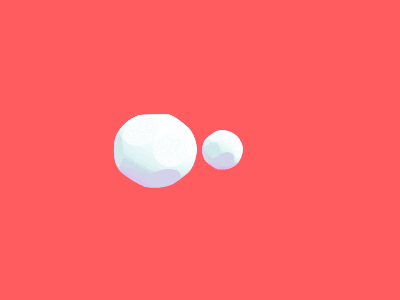
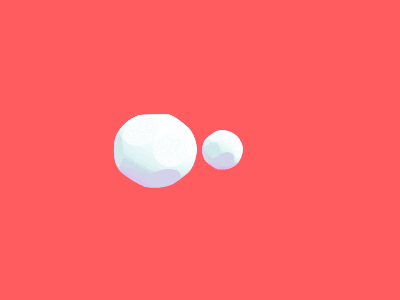
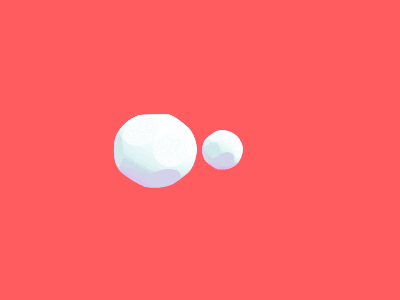
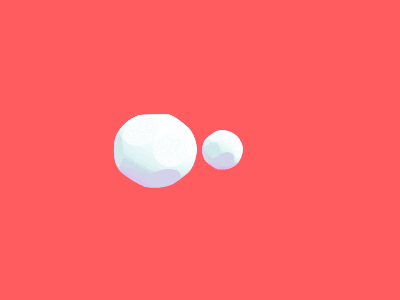
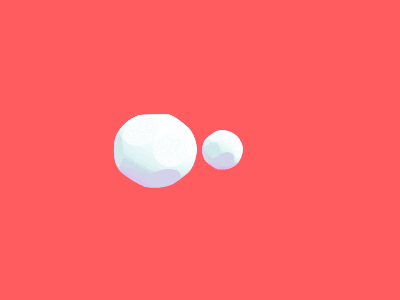
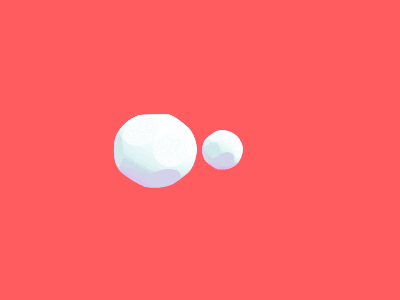
Advancing Hungarian Text Processing with HuSpaCy: Efficient and Accurate NLP Pipelines
Authors:György Orosz, Gergő Szabó, Péter Berkecz, Zsolt Szántó, Richárd Farkas
This paper presents a set of industrial-grade text processing models for Hungarian that achieve near state-of-the-art performance while balancing resource efficiency and accuracy. Models have been implemented in the spaCy framework, extending the HuSpaCy toolkit with several improvements to its architecture. Compared to existing NLP tools for Hungarian, all of our pipelines feature all basic text processing steps including tokenization, sentence-boundary detection, part-of-speech tagging, morphological feature tagging, lemmatization, dependency parsing and named entity recognition with high accuracy and throughput. We thoroughly evaluated the proposed enhancements, compared the pipelines with state-of-the-art tools and demonstrated the competitive performance of the new models in all text preprocessing steps. All experiments are reproducible and the pipelines are freely available under a permissive license.
PDF Submitted to TSD 2023 Conference
点此查看论文截图
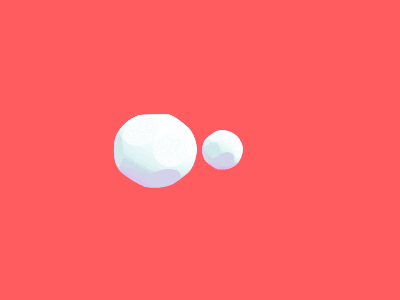
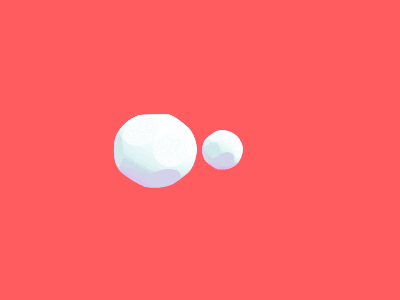
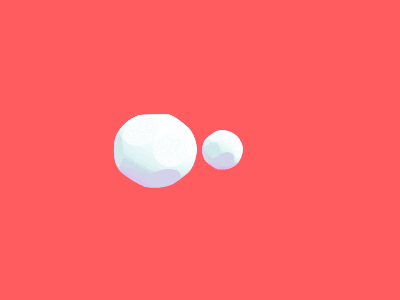