2023-05-25 更新
Use of Speech Impairment Severity for Dysarthric Speech Recognition
Authors:Mengzhe Geng, Zengrui Jin, Tianzi Wang, Shujie Hu, Jiajun Deng, Mingyu Cui, Guinan Li, Jianwei Yu, Xurong Xie, Xunying Liu
A key challenge in dysarthric speech recognition is the speaker-level diversity attributed to both speaker-identity associated factors such as gender, and speech impairment severity. Most prior researches on addressing this issue focused on using speaker-identity only. To this end, this paper proposes a novel set of techniques to use both severity and speaker-identity in dysarthric speech recognition: a) multitask training incorporating severity prediction error; b) speaker-severity aware auxiliary feature adaptation; and c) structured LHUC transforms separately conditioned on speaker-identity and severity. Experiments conducted on UASpeech suggest incorporating additional speech impairment severity into state-of-the-art hybrid DNN, E2E Conformer and pre-trained Wav2vec 2.0 ASR systems produced statistically significant WER reductions up to 4.78% (14.03% relative). Using the best system the lowest published WER of 17.82% (51.25% on very low intelligibility) was obtained on UASpeech.
PDF Accepted to INTERSPEECH2023
点此查看论文截图
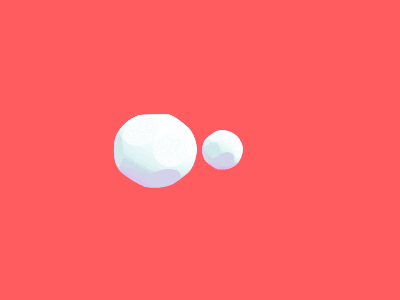
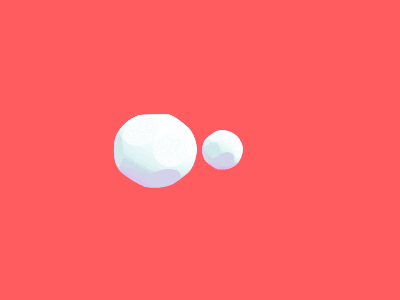
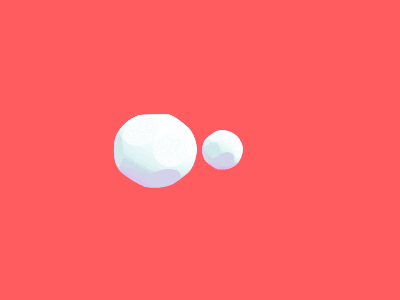
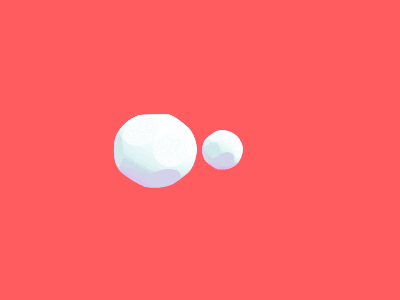
Diffusion-Based Mel-Spectrogram Enhancement for Personalized Speech Synthesis with Found Data
Authors:Yusheng Tian, Wei Liu, Tan Lee
Creating synthetic voices with found data is challenging, as real-world recordings often contain various types of audio degradation. One way to address this problem is to pre-enhance the speech with an enhancement model and then use the enhanced data for text-to-speech (TTS) model training. Ideally, the enhancement model should be able to tackle multiple types of audio degradation simultaneously. This paper investigates the use of conditional diffusion models for generalized speech enhancement. The enhancement is performed on the log Mel-spectrogram domain to align with the TTS training objective. Text information is introduced as an additional condition to improve the model robustness. Experiments on real-world recordings demonstrate that the synthetic voice built on data enhanced by the proposed model produces higher-quality synthetic speech, compared to those trained on data enhanced by strong baselines. Audio samples are available at \url{https://dmse4tts.github.io/}.
PDF
点此查看论文截图
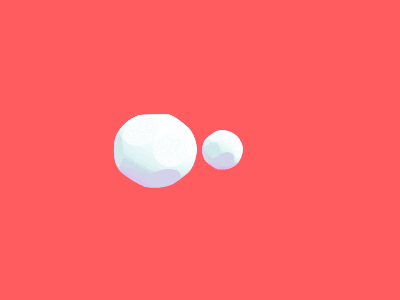
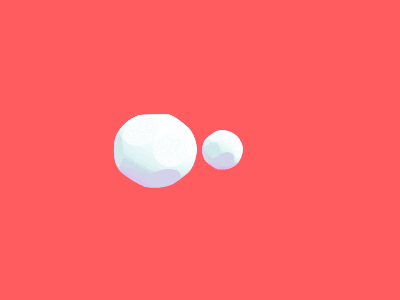
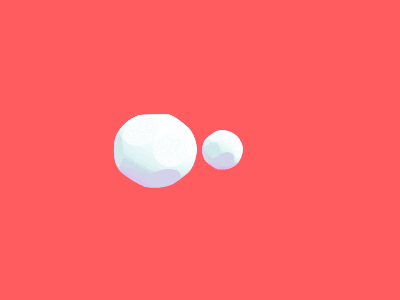
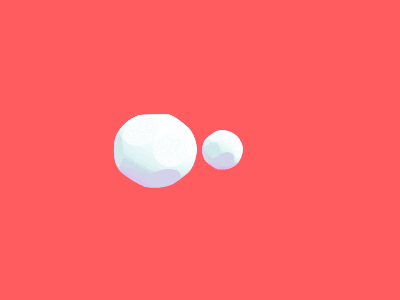
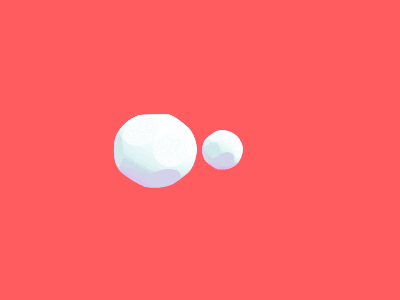
Federated learning for secure development of AI models for Parkinson’s disease detection using speech from different languages
Authors:Soroosh Tayebi Arasteh, Cristian David Rios-Urrego, Elmar Noeth, Andreas Maier, Seung Hee Yang, Jan Rusz, Juan Rafael Orozco-Arroyave
Parkinson’s disease (PD) is a neurological disorder impacting a person’s speech. Among automatic PD assessment methods, deep learning models have gained particular interest. Recently, the community has explored cross-pathology and cross-language models which can improve diagnostic accuracy even further. However, strict patient data privacy regulations largely prevent institutions from sharing patient speech data with each other. In this paper, we employ federated learning (FL) for PD detection using speech signals from 3 real-world language corpora of German, Spanish, and Czech, each from a separate institution. Our results indicate that the FL model outperforms all the local models in terms of diagnostic accuracy, while not performing very differently from the model based on centrally combined training sets, with the advantage of not requiring any data sharing among collaborators. This will simplify inter-institutional collaborations, resulting in enhancement of patient outcomes.
PDF Accepted for INTERSPEECH 2023
点此查看论文截图
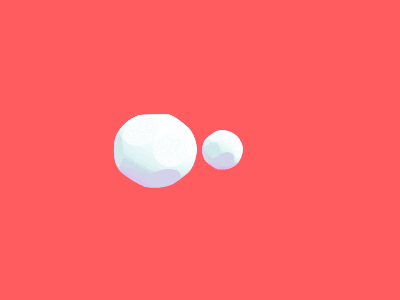
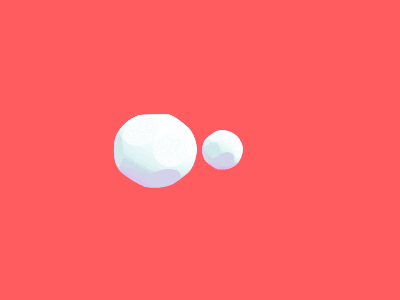
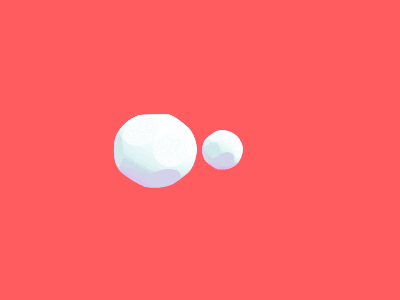
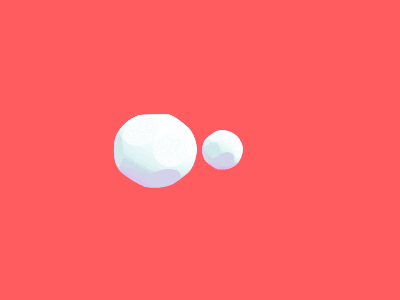
DualVC: Dual-mode Voice Conversion using Intra-model Knowledge Distillation and Hybrid Predictive Coding
Authors:Ziqian Ning, Yuepeng Jiang, Pengcheng Zhu, Jixun Yao, Shuai Wang, Lei Xie, Mengxiao Bi
Voice conversion is an increasingly popular technology, and the growing number of real-time applications requires models with streaming conversion capabilities. Unlike typical (non-streaming) voice conversion, which can leverage the entire utterance as full context, streaming voice conversion faces significant challenges due to the missing future information, resulting in degraded intelligibility, speaker similarity, and sound quality. To address this challenge, we propose DualVC, a dual-mode neural voice conversion approach that supports both streaming and non-streaming modes using jointly trained separate network parameters. Furthermore, we propose intra-model knowledge distillation and hybrid predictive coding (HPC) to enhance the performance of streaming conversion. Additionally, we incorporate data augmentation to train a noise-robust autoregressive decoder, improving the model’s performance on long-form speech conversion. Experimental results demonstrate that the proposed model outperforms the baseline models in the context of streaming voice conversion, while maintaining comparable performance to the non-streaming topline system that leverages the complete context, albeit with a latency of only 252.8 ms.
PDF
点此查看论文截图
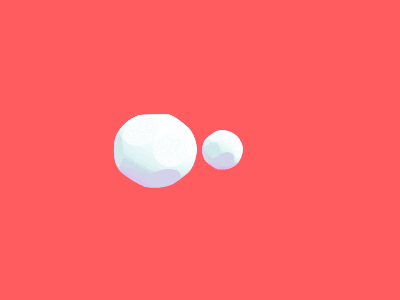
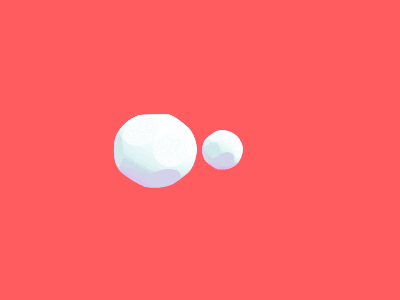
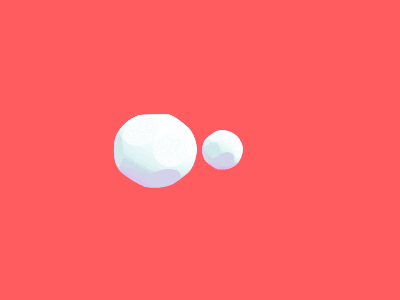
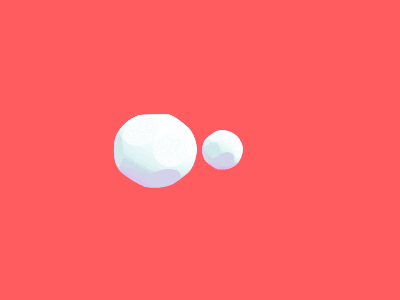
CASA-ASR: Context-Aware Speaker-Attributed ASR
Authors:Mohan Shi, Zhihao Du, Qian Chen, Fan Yu, Yangze Li, Shiliang Zhang, Jie Zhang, Li-Rong Dai
Recently, speaker-attributed automatic speech recognition (SA-ASR) has attracted a wide attention, which aims at answering the question ``who spoke what’’. Different from modular systems, end-to-end (E2E) SA-ASR minimizes the speaker-dependent recognition errors directly and shows a promising applicability. In this paper, we propose a context-aware SA-ASR (CASA-ASR) model by enhancing the contextual modeling ability of E2E SA-ASR. Specifically, in CASA-ASR, a contextual text encoder is involved to aggregate the semantic information of the whole utterance, and a context-dependent scorer is employed to model the speaker discriminability by contrasting with speakers in the context. In addition, a two-pass decoding strategy is further proposed to fully leverage the contextual modeling ability resulting in a better recognition performance. Experimental results on AliMeeting corpus show that the proposed CASA-ASR model outperforms the original E2E SA-ASR system with a relative improvement of 11.76% in terms of speaker-dependent character error rate.
PDF Accepted by Interspeech2023
点此查看论文截图
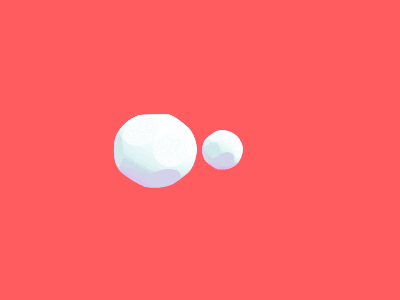
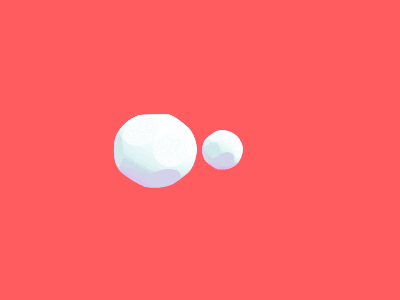
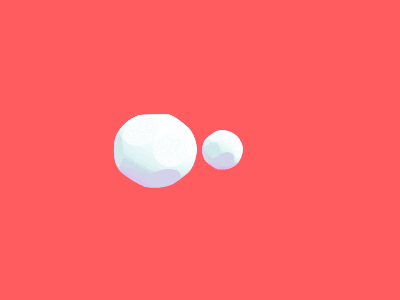
On the Efficacy and Noise-Robustness of Jointly Learned Speech Emotion and Automatic Speech Recognition
Authors:Lokesh Bansal, S. Pavankumar Dubagunta, Malolan Chetlur, Pushpak Jagtap, Aravind Ganapathiraju
New-age conversational agent systems perform both speech emotion recognition (SER) and automatic speech recognition (ASR) using two separate and often independent approaches for real-world application in noisy environments. In this paper, we investigate a joint ASR-SER multitask learning approach in a low-resource setting and show that improvements are observed not only in SER, but also in ASR. We also investigate the robustness of such jointly trained models to the presence of background noise, babble, and music. Experimental results on the IEMOCAP dataset show that joint learning can improve ASR word error rate (WER) and SER classification accuracy by 10.7% and 2.3% respectively in clean scenarios. In noisy scenarios, results on data augmented with MUSAN show that the joint approach outperforms the independent ASR and SER approaches across many noisy conditions. Overall, the joint ASR-SER approach yielded more noise-resistant models than the independent ASR and SER approaches.
PDF accepted to be part of INTERSPEECH 2023
点此查看论文截图
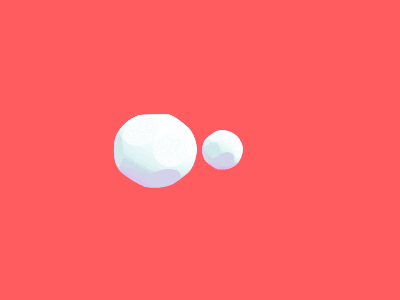
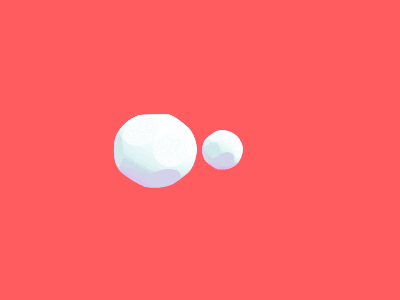
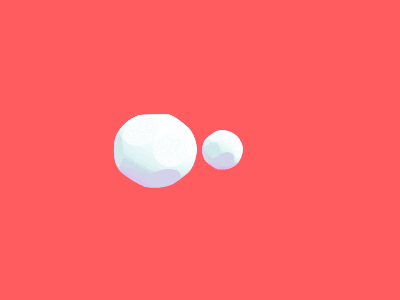
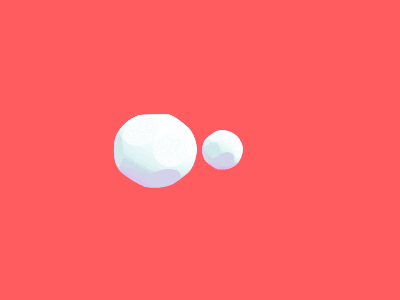
Duplex Diffusion Models Improve Speech-to-Speech Translation
Authors:Xianchao Wu
Speech-to-speech translation is a typical sequence-to-sequence learning task that naturally has two directions. How to effectively leverage bidirectional supervision signals to produce high-fidelity audio for both directions? Existing approaches either train two separate models or a multitask-learned model with low efficiency and inferior performance. In this paper, we propose a duplex diffusion model that applies diffusion probabilistic models to both sides of a reversible duplex Conformer, so that either end can simultaneously input and output a distinct language’s speech. Our model enables reversible speech translation by simply flipping the input and output ends. Experiments show that our model achieves the first success of reversible speech translation with significant improvements of ASR-BLEU scores compared with a list of state-of-the-art baselines.
PDF 11 pages, 3 figures. Accepted by ACL 2023 findings
点此查看论文截图
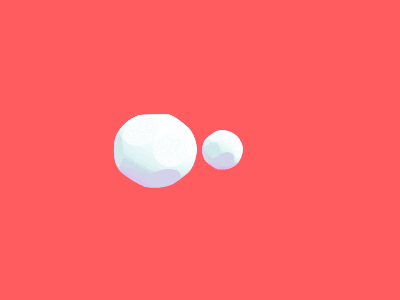
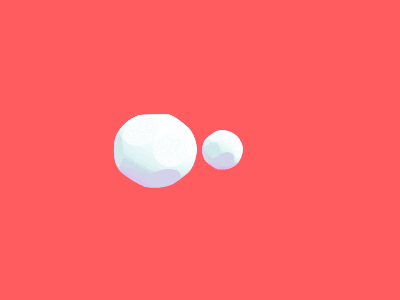
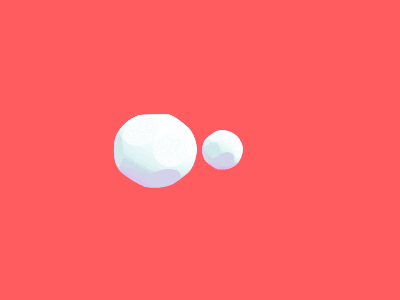
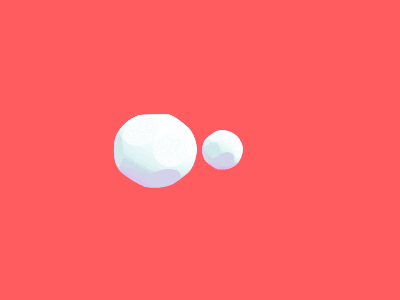
ViT-TTS: Visual Text-to-Speech with Scalable Diffusion Transformer
Authors:Huadai Liu, Rongjie Huang, Xuan Lin, Wenqiang Xu, Maozong Zheng, Hong Chen, Jinzheng He, Zhou Zhao
Text-to-speech(TTS) has undergone remarkable improvements in performance, particularly with the advent of Denoising Diffusion Probabilistic Models (DDPMs). However, the perceived quality of audio depends not solely on its content, pitch, rhythm, and energy, but also on the physical environment. In this work, we propose ViT-TTS, the first visual TTS model with scalable diffusion transformers. ViT-TTS complement the phoneme sequence with the visual information to generate high-perceived audio, opening up new avenues for practical applications of AR and VR to allow a more immersive and realistic audio experience. To mitigate the data scarcity in learning visual acoustic information, we 1) introduce a self-supervised learning framework to enhance both the visual-text encoder and denoiser decoder; 2) leverage the diffusion transformer scalable in terms of parameters and capacity to learn visual scene information. Experimental results demonstrate that ViT-TTS achieves new state-of-the-art results, outperforming cascaded systems and other baselines regardless of the visibility of the scene. With low-resource data (1h, 2h, 5h), ViT-TTS achieves comparative results with rich-resource baselines.~\footnote{Audio samples are available at \url{https://ViT-TTS.github.io/.}}
PDF
点此查看论文截图
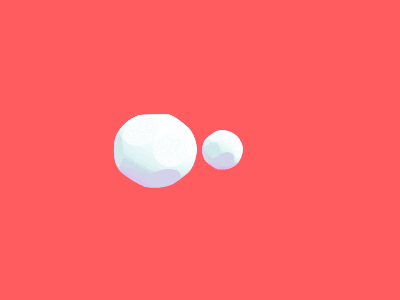
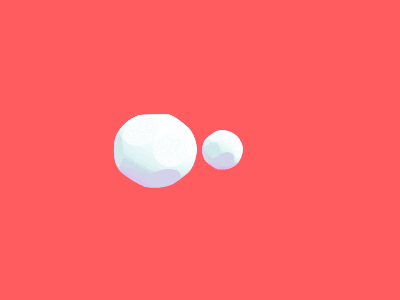
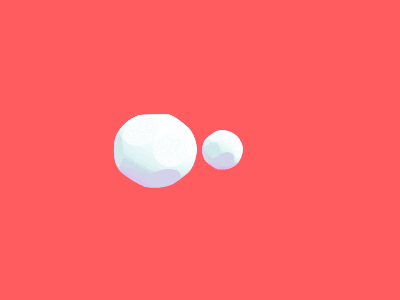
MP-SENet: A Speech Enhancement Model with Parallel Denoising of Magnitude and Phase Spectra
Authors:Ye-Xin Lu, Yang Ai, Zhen-Hua Ling
This paper proposes MP-SENet, a novel Speech Enhancement Network which directly denoises Magnitude and Phase spectra in parallel. The proposed MP-SENet adopts a codec architecture in which the encoder and decoder are bridged by convolution-augmented transformers. The encoder aims to encode time-frequency representations from the input noisy magnitude and phase spectra. The decoder is composed of parallel magnitude mask decoder and phase decoder, directly recovering clean magnitude spectra and clean-wrapped phase spectra by incorporating learnable sigmoid activation and parallel phase estimation architecture, respectively. Multi-level losses defined on magnitude spectra, phase spectra, short-time complex spectra, and time-domain waveforms are used to train the MP-SENet model jointly. Experimental results show that our proposed MP-SENet achieves a PESQ of 3.50 on the public VoiceBank+DEMAND dataset and outperforms existing advanced speech enhancement methods.
PDF Accepted by Interspeech 2023
点此查看论文截图
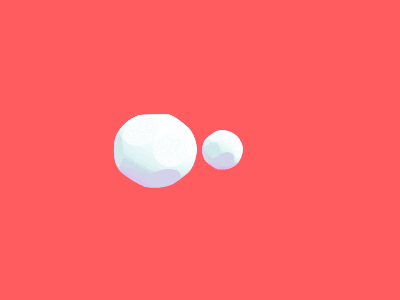
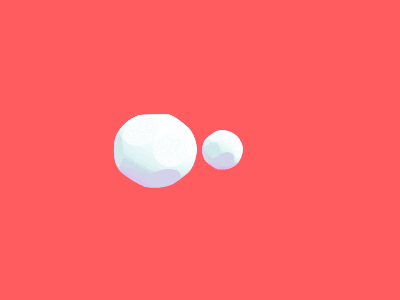
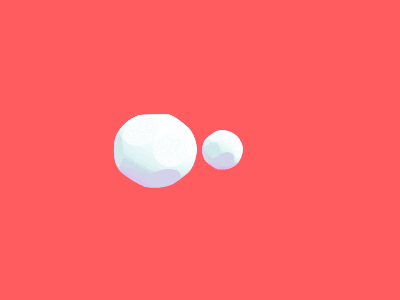
Parts of Speech-Grounded Subspaces in Vision-Language Models
Authors:James Oldfield, Christos Tzelepis, Yannis Panagakis, Mihalis A. Nicolaou, Ioannis Patras
Latent image representations arising from vision-language models have proved immensely useful for a variety of downstream tasks. However, their utility is limited by their entanglement with respect to different visual attributes. For instance, recent work has shown that CLIP image representations are often biased toward specific visual properties (such as objects or actions) in an unpredictable manner. In this paper, we propose to separate representations of the different visual modalities in CLIP’s joint vision-language space by leveraging the association between parts of speech and specific visual modes of variation (e.g. nouns relate to objects, adjectives describe appearance). This is achieved by formulating an appropriate component analysis model that learns subspaces capturing variability corresponding to a specific part of speech, while jointly minimising variability to the rest. Such a subspace yields disentangled representations of the different visual properties of an image or text in closed form while respecting the underlying geometry of the manifold on which the representations lie. What’s more, we show the proposed model additionally facilitates learning subspaces corresponding to specific visual appearances (e.g. artists’ painting styles), which enables the selective removal of entire visual themes from CLIP-based text-to-image synthesis. We validate the model both qualitatively, by visualising the subspace projections with a text-to-image model and by preventing the imitation of artists’ styles, and quantitatively, through class invariance metrics and improvements to baseline zero-shot classification. Our code is available at: https://github.com/james-oldfield/PoS-subspaces.
PDF
点此查看论文截图
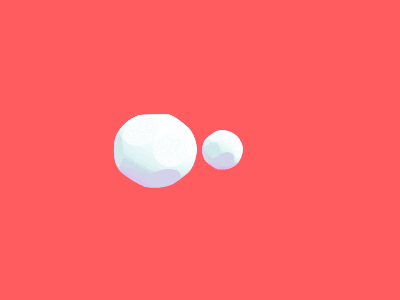
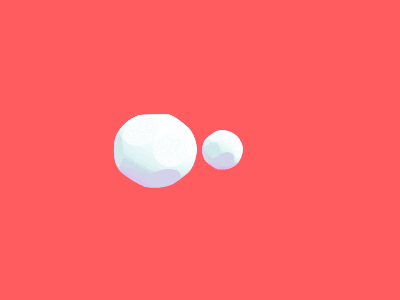
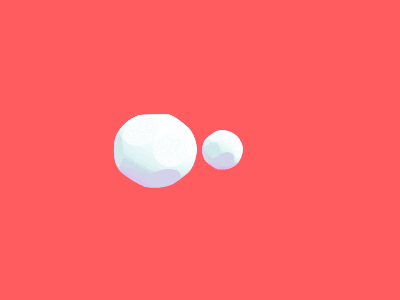
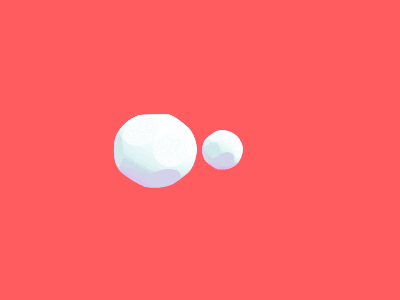
Masked Modeling Duo for Speech: Specializing General-Purpose Audio Representation to Speech using Denoising Distillation
Authors:Daisuke Niizumi, Daiki Takeuchi, Yasunori Ohishi, Noboru Harada, Kunio Kashino
Self-supervised learning general-purpose audio representations have demonstrated high performance in a variety of tasks. Although they can be optimized for application by fine-tuning, even higher performance can be expected if they can be specialized to pre-train for an application. This paper explores the challenges and solutions in specializing general-purpose audio representations for a specific application using speech, a highly demanding field, as an example. We enhance Masked Modeling Duo (M2D), a general-purpose model, to close the performance gap with state-of-the-art (SOTA) speech models. To do so, we propose a new task, denoising distillation, to learn from fine-grained clustered features, and M2D for Speech (M2D-S), which jointly learns the denoising distillation task and M2D masked prediction task. Experimental results show that M2D-S performs comparably to or outperforms SOTA speech models on the SUPERB benchmark, demonstrating that M2D can specialize in a demanding field. Our code is available at: https://github.com/nttcslab/m2d/tree/master/speech
PDF Interspeech 2023; 5 pages, 2 figures, 6 tables, Code: https://github.com/nttcslab/m2d/tree/master/speech
点此查看论文截图
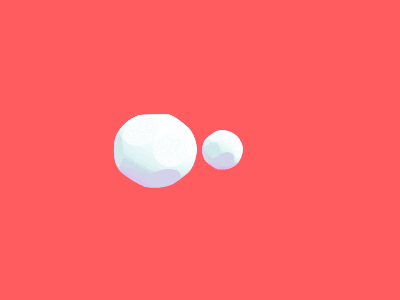
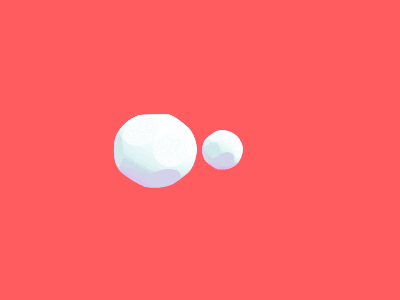
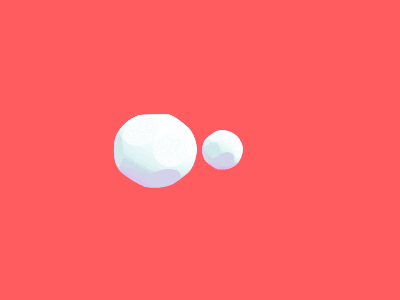
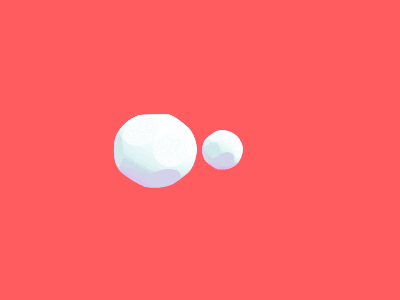
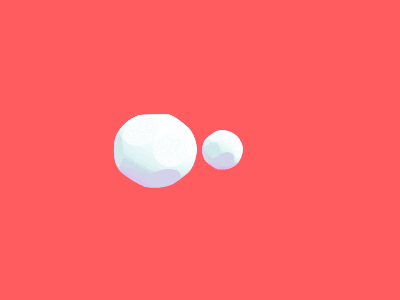
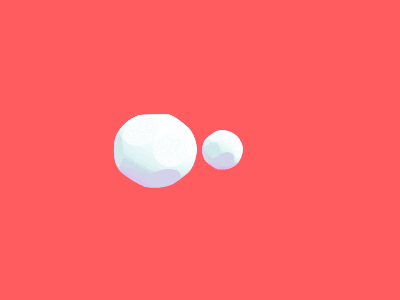
On the Transferability of Whisper-based Representations for “In-the-Wild” Cross-Task Downstream Speech Applications
Authors:Vamsikrishna Chemudupati, Marzieh Tahaei, Heitor Guimaraes, Arthur Pimentel, Anderson Avila, Mehdi Rezagholizadeh, Boxing Chen, Tiago Falk
Large self-supervised pre-trained speech models have achieved remarkable success across various speech-processing tasks. The self-supervised training of these models leads to universal speech representations that can be used for different downstream tasks, ranging from automatic speech recognition (ASR) to speaker identification. Recently, Whisper, a transformer-based model was proposed and trained on large amount of weakly supervised data for ASR; it outperformed several state-of-the-art self-supervised models. Given the superiority of Whisper for ASR, in this paper we explore the transferability of the representation for four other speech tasks in SUPERB benchmark. Moreover, we explore the robustness of Whisper representation for ``in the wild’’ tasks where speech is corrupted by environment noise and room reverberation. Experimental results show Whisper achieves promising results across tasks and environmental conditions, thus showing potential for cross-task real-world deployment.
PDF
点此查看论文截图
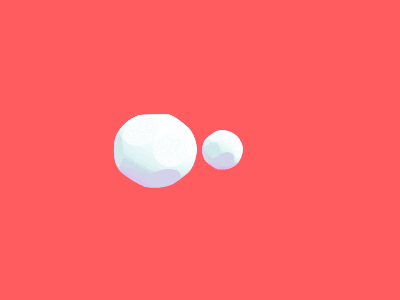
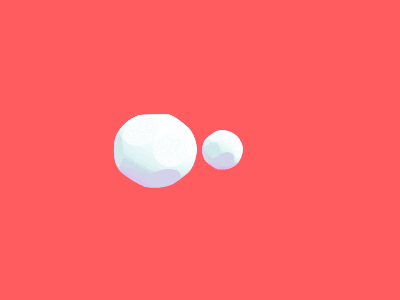
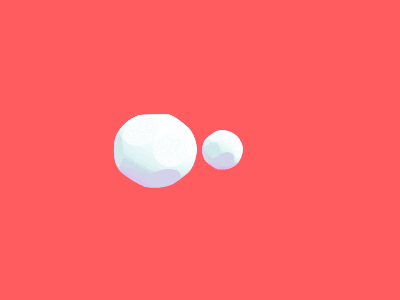
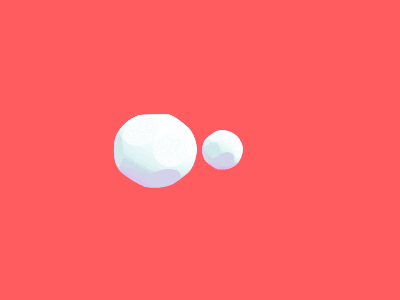
Evaluating OpenAI’s Whisper ASR for Punctuation Prediction and Topic Modeling of life histories of the Museum of the Person
Authors:Lucas Rafael Stefanel Gris, Ricardo Marcacini, Arnaldo Candido Junior, Edresson Casanova, Anderson Soares, Sandra Maria Aluísio
Automatic speech recognition (ASR) systems play a key role in applications involving human-machine interactions. Despite their importance, ASR models for the Portuguese language proposed in the last decade have limitations in relation to the correct identification of punctuation marks in automatic transcriptions, which hinder the use of transcriptions by other systems, models, and even by humans. However, recently Whisper ASR was proposed by OpenAI, a general-purpose speech recognition model that has generated great expectations in dealing with such limitations. This chapter presents the first study on the performance of Whisper for punctuation prediction in the Portuguese language. We present an experimental evaluation considering both theoretical aspects involving pausing points (comma) and complete ideas (exclamation, question, and fullstop), as well as practical aspects involving transcript-based topic modeling - an application dependent on punctuation marks for promising performance. We analyzed experimental results from videos of Museum of the Person, a virtual museum that aims to tell and preserve people’s life histories, thus discussing the pros and cons of Whisper in a real-world scenario. Although our experiments indicate that Whisper achieves state-of-the-art results, we conclude that some punctuation marks require improvements, such as exclamation, semicolon and colon.
PDF
点此查看论文截图
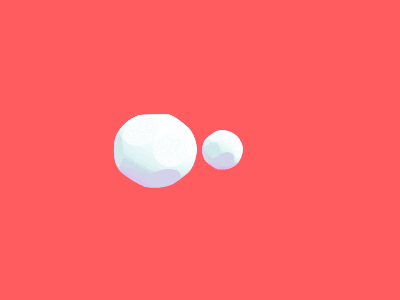
Incorporating Ultrasound Tongue Images for Audio-Visual Speech Enhancement through Knowledge Distillation
Authors:Rui-Chen Zheng, Yang Ai, Zhen-Hua Ling
Audio-visual speech enhancement (AV-SE) aims to enhance degraded speech along with extra visual information such as lip videos, and has been shown to be more effective than audio-only speech enhancement. This paper proposes further incorporating ultrasound tongue images to improve lip-based AV-SE systems’ performance. Knowledge distillation is employed at the training stage to address the challenge of acquiring ultrasound tongue images during inference, enabling an audio-lip speech enhancement student model to learn from a pre-trained audio-lip-tongue speech enhancement teacher model. Experimental results demonstrate significant improvements in the quality and intelligibility of the speech enhanced by the proposed method compared to the traditional audio-lip speech enhancement baselines. Further analysis using phone error rates (PER) of automatic speech recognition (ASR) shows that palatal and velar consonants benefit most from the introduction of ultrasound tongue images.
PDF To be published in InterSpeech 2023
点此查看论文截图
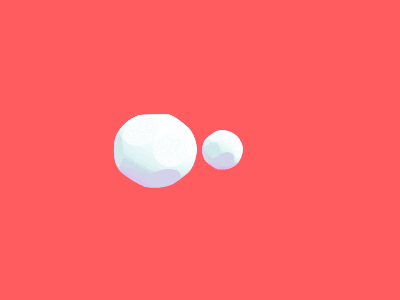
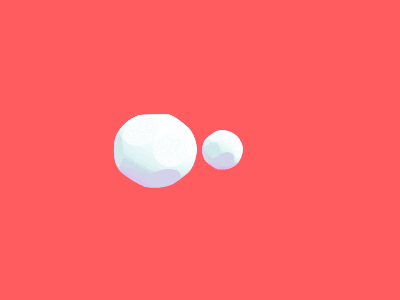
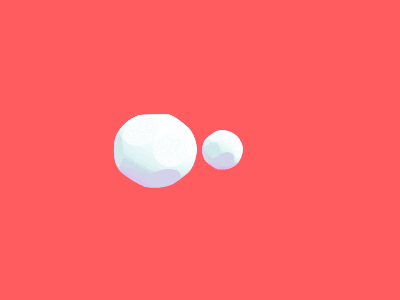
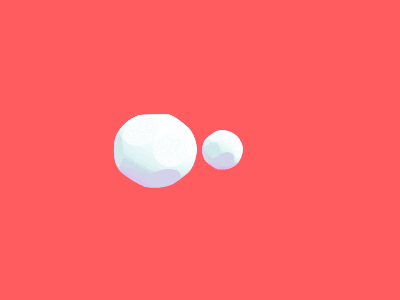