2023-05-10 更新
Hybrid Transducer and Attention based Encoder-Decoder Modeling for Speech-to-Text Tasks
Authors:Yun Tang, Anna Y. Sun, Hirofumi Inaguma, Xinyue Chen, Ning Dong, Xutai Ma, Paden D. Tomasello, Juan Pino
Transducer and Attention based Encoder-Decoder (AED) are two widely used frameworks for speech-to-text tasks. They are designed for different purposes and each has its own benefits and drawbacks for speech-to-text tasks. In order to leverage strengths of both modeling methods, we propose a solution by combining Transducer and Attention based Encoder-Decoder (TAED) for speech-to-text tasks. The new method leverages AED’s strength in non-monotonic sequence to sequence learning while retaining Transducer’s streaming property. In the proposed framework, Transducer and AED share the same speech encoder. The predictor in Transducer is replaced by the decoder in the AED model, and the outputs of the decoder are conditioned on the speech inputs instead of outputs from an unconditioned language model. The proposed solution ensures that the model is optimized by covering all possible read/write scenarios and creates a matched environment for streaming applications. We evaluate the proposed approach on the \textsc{MuST-C} dataset and the findings demonstrate that TAED performs significantly better than Transducer for offline automatic speech recognition (ASR) and speech-to-text translation (ST) tasks. In the streaming case, TAED outperforms Transducer in the ASR task and one ST direction while comparable results are achieved in another translation direction.
PDF ACL 2023 main conference
点此查看论文截图
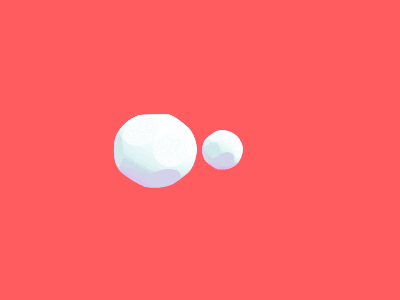
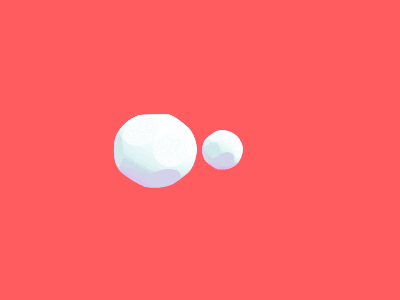
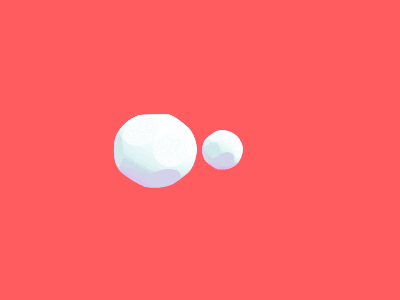
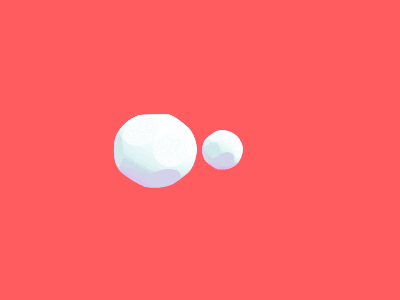
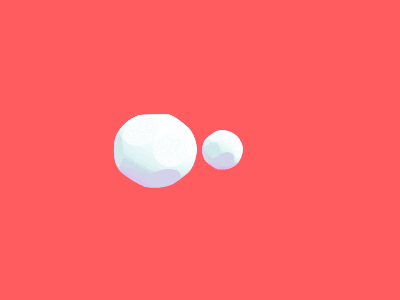
Multi-Temporal Lip-Audio Memory for Visual Speech Recognition
Authors:Jeong Hun Yeo, Minsu Kim, Yong Man Ro
Visual Speech Recognition (VSR) is a task to predict a sentence or word from lip movements. Some works have been recently presented which use audio signals to supplement visual information. However, existing methods utilize only limited information such as phoneme-level features and soft labels of Automatic Speech Recognition (ASR) networks. In this paper, we present a Multi-Temporal Lip-Audio Memory (MTLAM) that makes the best use of audio signals to complement insufficient information of lip movements. The proposed method is mainly composed of two parts: 1) MTLAM saves multi-temporal audio features produced from short- and long-term audio signals, and the MTLAM memorizes a visual-to-audio mapping to load stored multi-temporal audio features from visual features at the inference phase. 2) We design an audio temporal model to produce multi-temporal audio features capturing the context of neighboring words. In addition, to construct effective visual-to-audio mapping, the audio temporal models can generate audio features time-aligned with visual features. Through extensive experiments, we validate the effectiveness of the MTLAM achieving state-of-the-art performances on two public VSR datasets.
PDF Presented at ICASSP 2023
点此查看论文截图
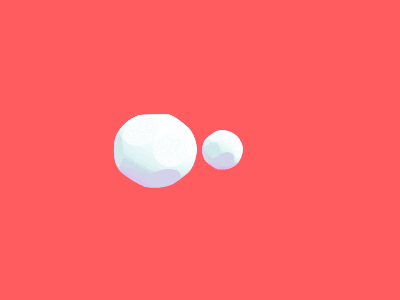
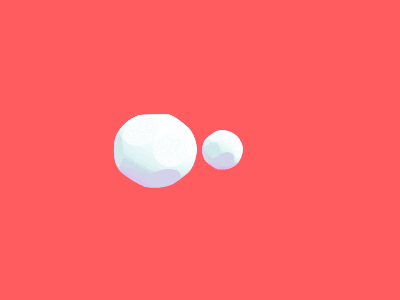
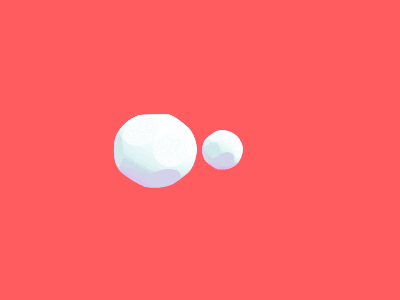
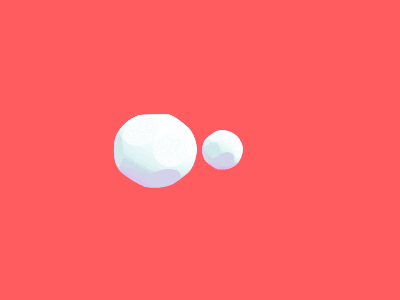
Fast Conformer with Linearly Scalable Attention for Efficient Speech Recognition
Authors:Dima Rekesh, Samuel Kriman, Somshubra Majumdar, Vahid Noroozi, He Juang, Oleksii Hrinchuk, Ankur Kumar, Boris Ginsburg
Conformer-based models have become the most dominant end- to-end architecture for speech processing tasks. In this work, we propose a carefully redesigned Conformer with a new down- sampling schema. The proposed model, named Fast Con- former, is 2.8x faster than original Conformer, while preserv- ing state-of-the-art accuracy on Automatic Speech Recognition benchmarks. Also we replace the original Conformer global attention with limited context attention post-training to enable transcription of an hour-long audio. We further improve long- form speech transcription by adding a global token. Fast Con- former combined with a Transformer decoder also outperforms the original Conformer in accuracy and in speed for Speech Translation and Spoken Language Understanding.
PDF
点此查看论文截图
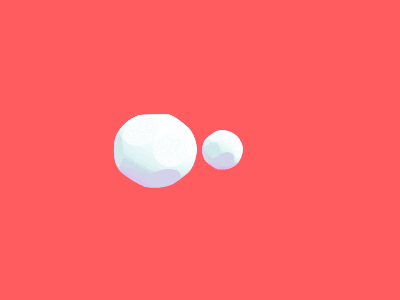
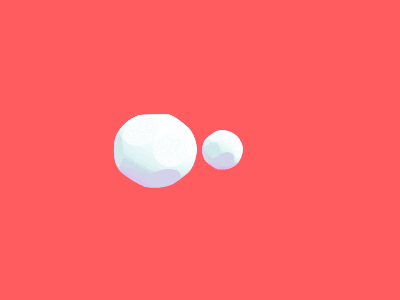
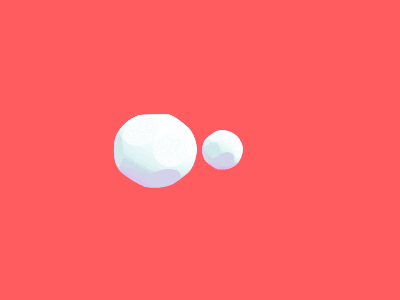
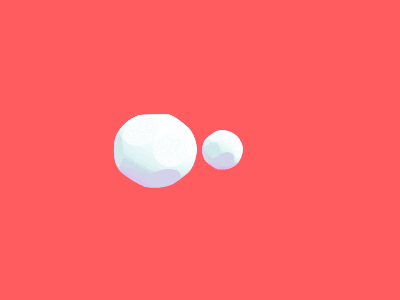
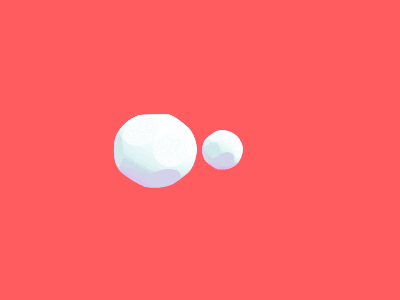
Who Needs Decoders? Efficient Estimation of Sequence-level Attributes
Authors:Yassir Fathullah, Puria Radmard, Adian Liusie, Mark J. F. Gales
State-of-the-art sequence-to-sequence models often require autoregressive decoding, which can be highly expensive. However, for some downstream tasks such as out-of-distribution (OOD) detection and resource allocation, the actual decoding output is not needed just a scalar attribute of this sequence. In these scenarios, where for example knowing the quality of a system’s output to predict poor performance prevails over knowing the output itself, is it possible to bypass the autoregressive decoding? We propose Non-Autoregressive Proxy (NAP) models that can efficiently predict general scalar-valued sequence-level attributes. Importantly, NAPs predict these metrics directly from the encodings, avoiding the expensive autoregressive decoding stage. We consider two sequence-to-sequence task: Machine Translation (MT); and Automatic Speech Recognition (ASR). In OOD for MT, NAPs outperform a deep ensemble while being significantly faster. NAPs are also shown to be able to predict performance metrics such as BERTScore (MT) or word error rate (ASR). For downstream tasks, such as data filtering and resource optimization, NAPs generate performance predictions that outperform predictive uncertainty while being highly inference efficient.
PDF
点此查看论文截图
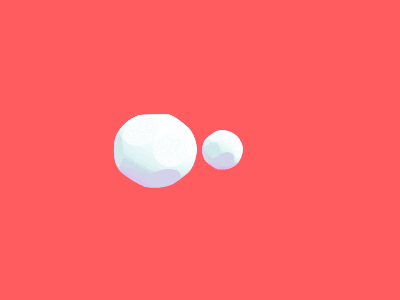
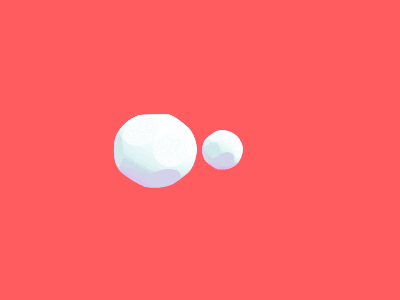
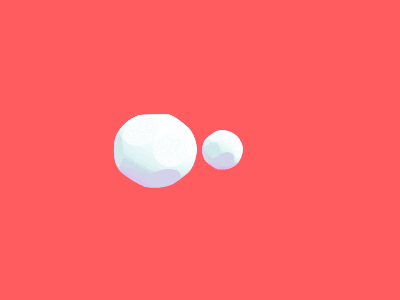
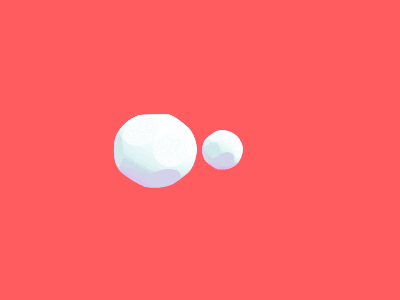
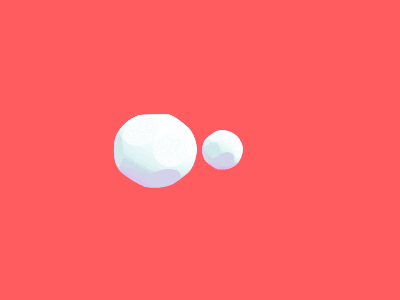
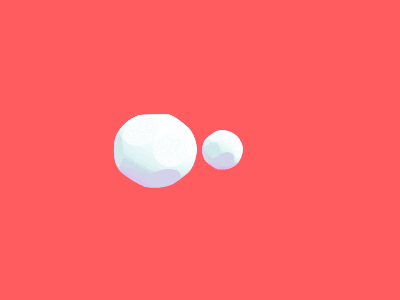
Exploration of Language Dependency for Japanese Self-Supervised Speech Representation Models
Authors:Takanori Ashihara, Takafumi Moriya, Kohei Matsuura, Tomohiro Tanaka
Self-supervised learning (SSL) has been dramatically successful not only in monolingual but also in cross-lingual settings. However, since the two settings have been studied individually in general, there has been little research focusing on how effective a cross-lingual model is in comparison with a monolingual model. In this paper, we investigate this fundamental question empirically with Japanese automatic speech recognition (ASR) tasks. First, we begin by comparing the ASR performance of cross-lingual and monolingual models for two different language tasks while keeping the acoustic domain as identical as possible. Then, we examine how much unlabeled data collected in Japanese is needed to achieve performance comparable to a cross-lingual model pre-trained with tens of thousands of hours of English and/or multilingual data. Finally, we extensively investigate the effectiveness of SSL in Japanese and demonstrate state-of-the-art performance on multiple ASR tasks. Since there is no comprehensive SSL study for Japanese, we hope this study will guide Japanese SSL research.
PDF Accepted at ICASSP 2023
点此查看论文截图
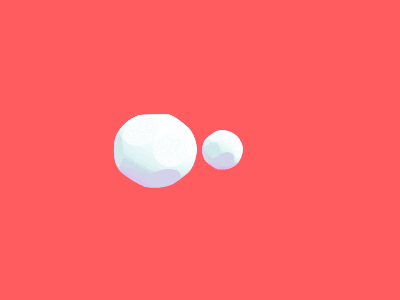
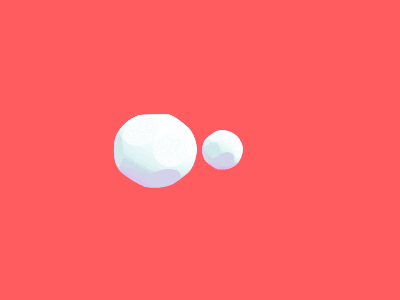
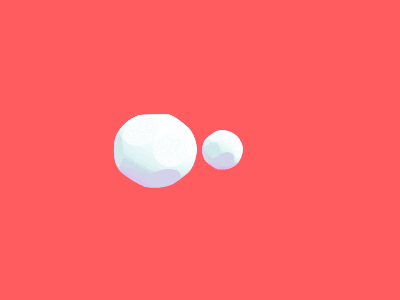
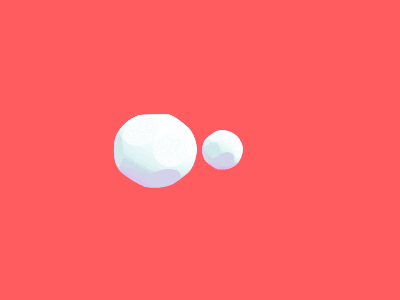
Robust Acoustic and Semantic Contextual Biasing in Neural Transducers for Speech Recognition
Authors:Xuandi Fu, Kanthashree Mysore Sathyendra, Ankur Gandhe, Jing Liu, Grant P. Strimel, Ross McGowan, Athanasios Mouchtaris
Attention-based contextual biasing approaches have shown significant improvements in the recognition of generic and/or personal rare-words in End-to-End Automatic Speech Recognition (E2E ASR) systems like neural transducers. These approaches employ cross-attention to bias the model towards specific contextual entities injected as bias-phrases to the model. Prior approaches typically relied on subword encoders for encoding the bias phrases. However, subword tokenizations are coarse and fail to capture granular pronunciation information which is crucial for biasing based on acoustic similarity. In this work, we propose to use lightweight character representations to encode fine-grained pronunciation features to improve contextual biasing guided by acoustic similarity between the audio and the contextual entities (termed acoustic biasing). We further integrate pretrained neural language model (NLM) based encoders to encode the utterance’s semantic context along with contextual entities to perform biasing informed by the utterance’s semantic context (termed semantic biasing). Experiments using a Conformer Transducer model on the Librispeech dataset show a 4.62% - 9.26% relative WER improvement on different biasing list sizes over the baseline contextual model when incorporating our proposed acoustic and semantic biasing approach. On a large-scale in-house dataset, we observe 7.91% relative WER improvement compared to our baseline model. On tail utterances, the improvements are even more pronounced with 36.80% and 23.40% relative WER improvements on Librispeech rare words and an in-house testset respectively.
PDF Accepted at ICASSP 2023
点此查看论文截图
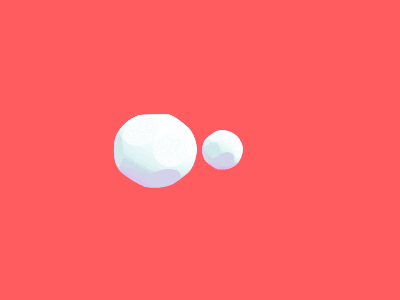
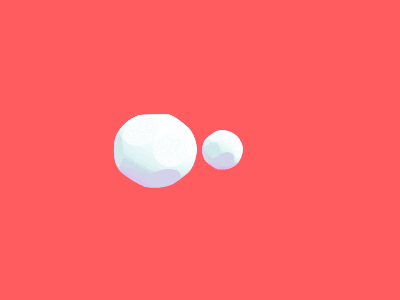
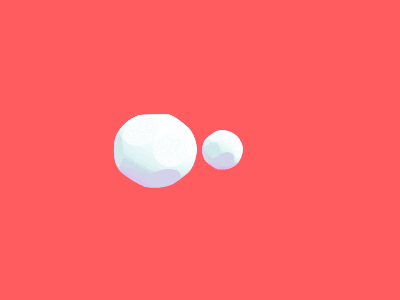
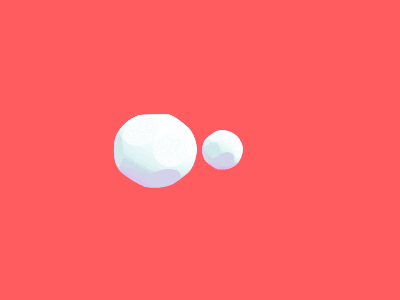