2023-03-22 更新
Deep Composite Face Image Attacks: Generation, Vulnerability and Detection
Authors:Jag Mohan Singh, Raghavendra Ramachandra
Face manipulation attacks have drawn the attention of biometric researchers because of their vulnerability to Face Recognition Systems (FRS). This paper proposes a novel scheme to generate Composite Face Image Attacks (CFIA) based on facial attributes using Generative Adversarial Networks (GANs). Given the face images corresponding to two unique data subjects, the proposed CFIA method will independently generate the segmented facial attributes, then blend them using transparent masks to generate the CFIA samples. We generate $526$ unique CFIA combinations of facial attributes for each pair of contributory data subjects. Extensive experiments are carried out on our newly generated CFIA dataset consisting of 1000 unique identities with 2000 bona fide samples and 526000 CFIA samples, thus resulting in an overall 528000 face image samples. We present a sequence of experiments to benchmark the attack potential of CFIA samples using four different automatic FRS. We introduced a new metric named Generalized Morphing Attack Potential (G-MAP) to benchmark the vulnerability of generated attacks on FRS effectively. Additional experiments are performed on the representative subset of the CFIA dataset to benchmark both perceptual quality and human observer response. Finally, the CFIA detection performance is benchmarked using three different single image based face Morphing Attack Detection (MAD) algorithms. The source code of the proposed method together with CFIA dataset will be made publicly available: \url{https://github.com/jagmohaniiit/LatentCompositionCode}
PDF The submitted paper is accepted in IEEE Access 2023
点此查看论文截图
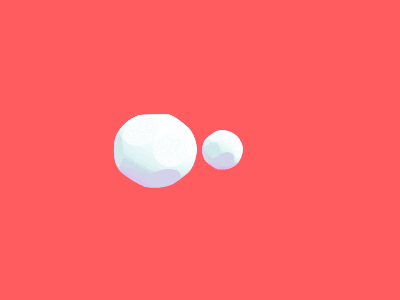
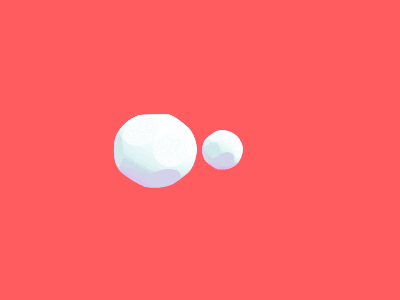
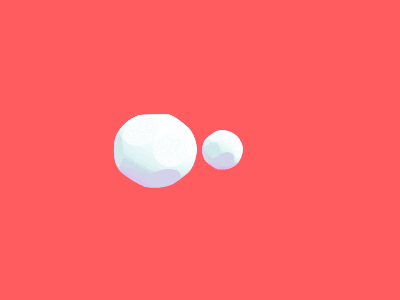
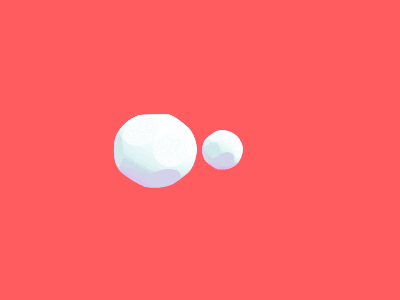
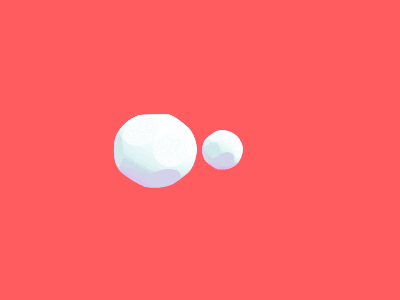
Explain To Me: Salience-Based Explainability for Synthetic Face Detection Models
Authors:Colton Crum, Patrick Tinsley, Aidan Boyd, Jacob Piland, Christopher Sweet, Timothy Kelley, Kevin Bowyer, Adam Czajka
The performance of convolutional neural networks has continued to improve over the last decade. At the same time, as model complexity grows, it becomes increasingly more difficult to explain model decisions. Such explanations may be of critical importance for reliable operation of human-machine pairing setups, or for model selection when the “best” model among many equally-accurate models must be established. Saliency maps represent one popular way of explaining model decisions by highlighting image regions models deem important when making a prediction. However, examining salience maps at scale is not practical. In this paper, we propose five novel methods of leveraging model salience to explain a model behavior at scale. These methods ask: (a) what is the average entropy for a model’s salience maps, (b) how does model salience change when fed out-of-set samples, (c) how closely does model salience follow geometrical transformations, (d) what is the stability of model salience across independent training runs, and (e) how does model salience react to salience-guided image degradations. To assess the proposed measures on a concrete and topical problem, we conducted a series of experiments for the task of synthetic face detection with two types of models: those trained traditionally with cross-entropy loss, and those guided by human salience when training to increase model generalizability. These two types of models are characterized by different, interpretable properties of their salience maps, which allows for the evaluation of the correctness of the proposed measures. We offer source codes for each measure along with this paper.
PDF 13 pages, 10 figures
点此查看论文截图
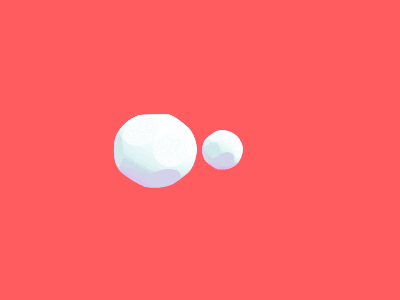
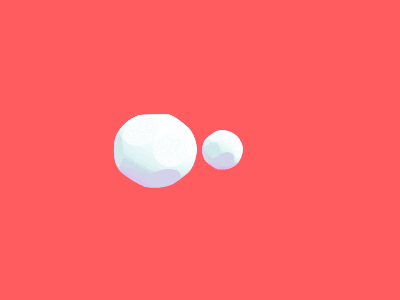
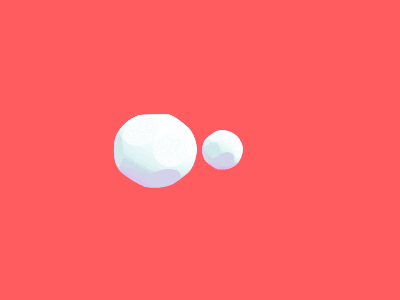
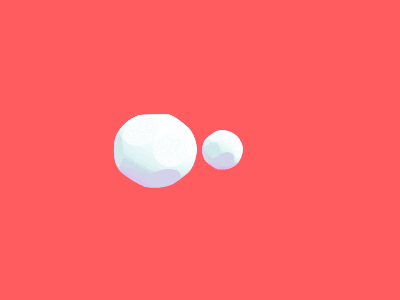
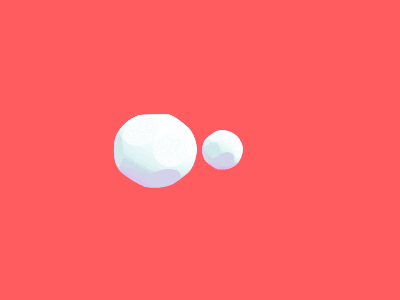