2023-03-17 更新
Mimic3D: Thriving 3D-Aware GANs via 3D-to-2D Imitation
Authors:Xingyu Chen, Yu Deng, Baoyuan Wang
Generating images with both photorealism and multiview 3D consistency is crucial for 3D-aware GANs, yet existing methods struggle to achieve them simultaneously. Improving the photorealism via CNN-based 2D super-resolution can break the strict 3D consistency, while keeping the 3D consistency by learning high-resolution 3D representations for direct rendering often compromises image quality. In this paper, we propose a novel learning strategy, namely 3D-to-2D imitation, which enables a 3D-aware GAN to generate high-quality images while maintaining their strict 3D consistency, by letting the images synthesized by the generator’s 3D rendering branch to mimic those generated by its 2D super-resolution branch. We also introduce 3D-aware convolutions into the generator for better 3D representation learning, which further improves the image generation quality. With the above strategies, our method reaches FID scores of 5.4 and 4.3 on FFHQ and AFHQ-v2 Cats, respectively, at 512x512 resolution, largely outperforming existing 3D-aware GANs using direct 3D rendering and coming very close to the previous state-of-the-art method that leverages 2D super-resolution. Project website: https://seanchenxy.github.io/Mimic3DWeb.
PDF
点此查看论文截图
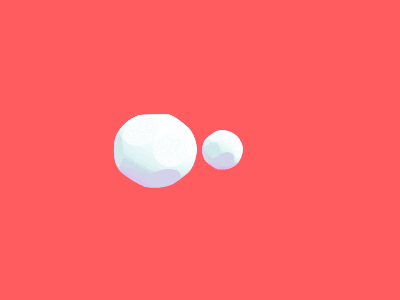
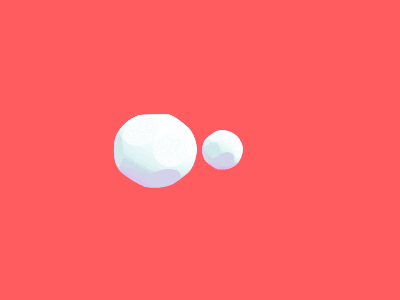
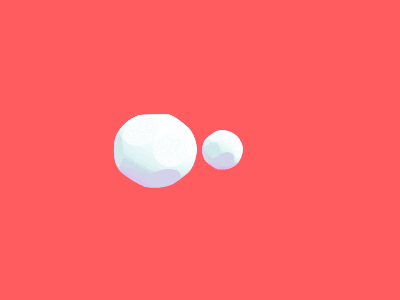
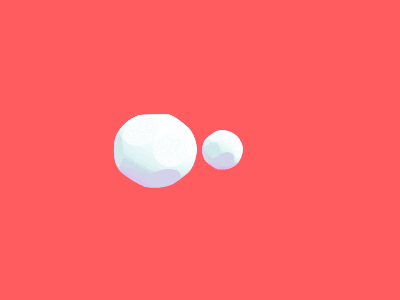
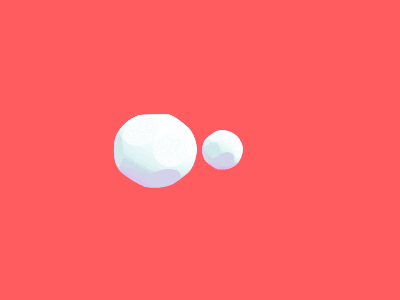
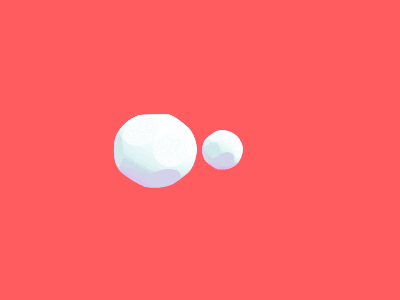
Text-to-ECG: 12-Lead Electrocardiogram Synthesis conditioned on Clinical Text Reports
Authors:Hyunseung Chung, Jiho Kim, Joon-myoung Kwon, Ki-Hyun Jeon, Min Sung Lee, Edward Choi
Electrocardiogram (ECG) synthesis is the area of research focused on generating realistic synthetic ECG signals for medical use without concerns over annotation costs or clinical data privacy restrictions. Traditional ECG generation models consider a single ECG lead and utilize GAN-based generative models. These models can only generate single lead samples and require separate training for each diagnosis class. The diagnosis classes of ECGs are insufficient to capture the intricate differences between ECGs depending on various features (e.g. patient demographic details, co-existing diagnosis classes, etc.). To alleviate these challenges, we present a text-to-ECG task, in which textual inputs are used to produce ECG outputs. Then we propose Auto-TTE, an autoregressive generative model conditioned on clinical text reports to synthesize 12-lead ECGs, for the first time to our knowledge. We compare the performance of our model with other representative models in text-to-speech and text-to-image. Experimental results show the superiority of our model in various quantitative evaluations and qualitative analysis. Finally, we conduct a user study with three board-certified cardiologists to confirm the fidelity and semantic alignment of generated samples. our code will be available at https://github.com/TClife/text_to_ecg
PDF Accepted to ICASSP 2023 (5 pages, 3 figures, 4 tables)
点此查看论文截图
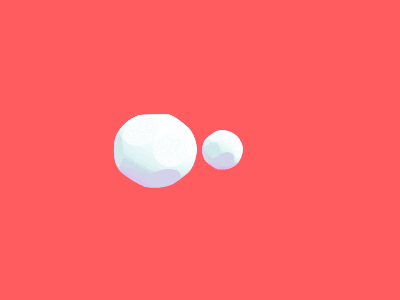
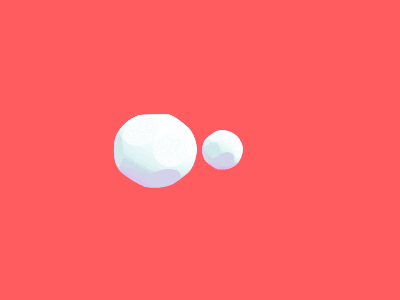
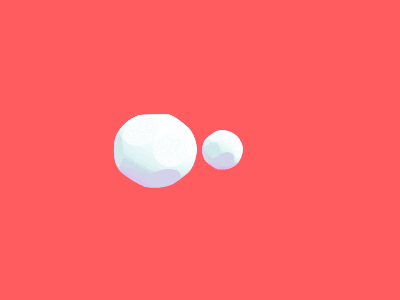
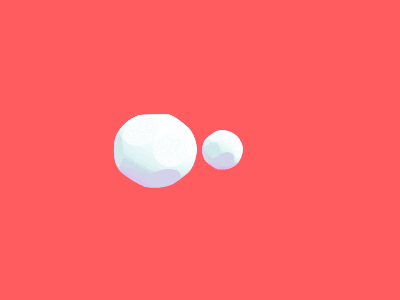
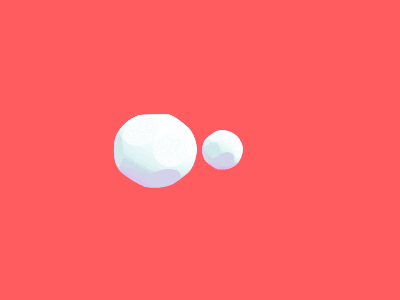