2022-12-08 更新
Cyclically Disentangled Feature Translation for Face Anti-spoofing
Authors:Haixiao Yue, Keyao Wang, Guosheng Zhang, Haocheng Feng, Junyu Han, Errui Ding, Jingdong Wang
Current domain adaptation methods for face anti-spoofing leverage labeled source domain data and unlabeled target domain data to obtain a promising generalizable decision boundary. However, it is usually difficult for these methods to achieve a perfect domain-invariant liveness feature disentanglement, which may degrade the final classification performance by domain differences in illumination, face category, spoof type, etc. In this work, we tackle cross-scenario face anti-spoofing by proposing a novel domain adaptation method called cyclically disentangled feature translation network (CDFTN). Specifically, CDFTN generates pseudo-labeled samples that possess: 1) source domain-invariant liveness features and 2) target domain-specific content features, which are disentangled through domain adversarial training. A robust classifier is trained based on the synthetic pseudo-labeled images under the supervision of source domain labels. We further extend CDFTN for multi-target domain adaptation by leveraging data from more unlabeled target domains. Extensive experiments on several public datasets demonstrate that our proposed approach significantly outperforms the state of the art.
PDF Accepted by AAAI2023
点此查看论文截图
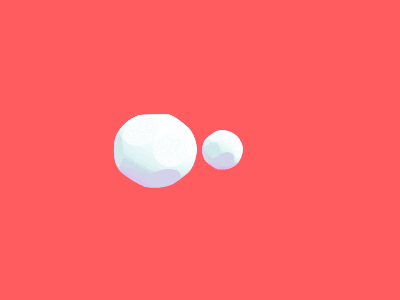
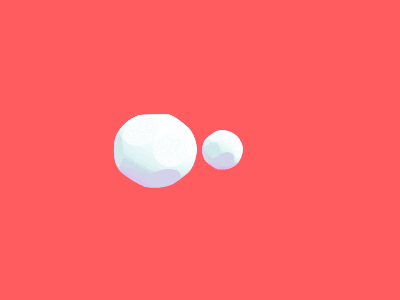
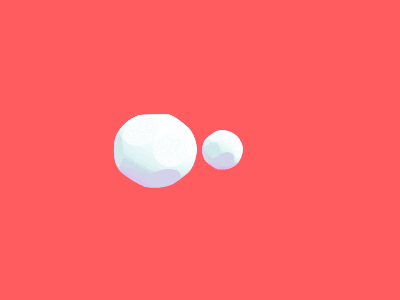
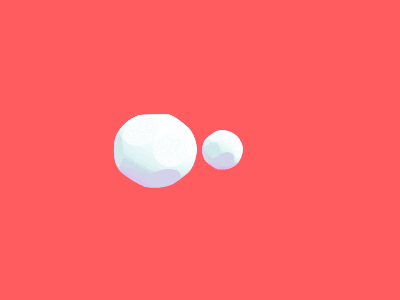
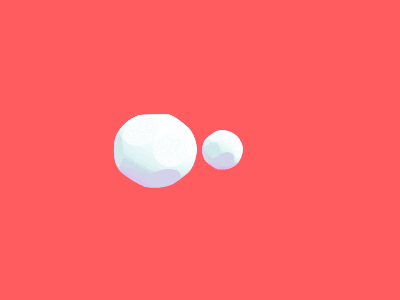
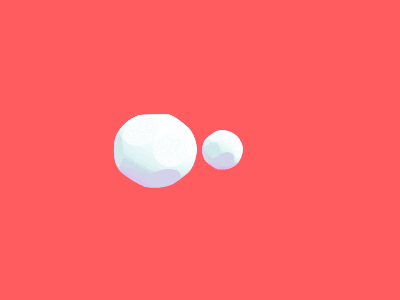
Voice Spoofing Countermeasures: Taxonomy, State-of-the-art, experimental analysis of generalizability, open challenges, and the way forward
Authors:Awais Khan, Khalid Mahmood Malik, James Ryan, Mikul Saravanan
Malicious actors may seek to use different voice-spoofing attacks to fool ASV systems and even use them for spreading misinformation. Various countermeasures have been proposed to detect these spoofing attacks. Due to the extensive work done on spoofing detection in automated speaker verification (ASV) systems in the last 6-7 years, there is a need to classify the research and perform qualitative and quantitative comparisons on state-of-the-art countermeasures. Additionally, no existing survey paper has reviewed integrated solutions to voice spoofing evaluation and speaker verification, adversarial/antiforensics attacks on spoofing countermeasures, and ASV itself, or unified solutions to detect multiple attacks using a single model. Further, no work has been done to provide an apples-to-apples comparison of published countermeasures in order to assess their generalizability by evaluating them across corpora. In this work, we conduct a review of the literature on spoofing detection using hand-crafted features, deep learning, end-to-end, and universal spoofing countermeasure solutions to detect speech synthesis (SS), voice conversion (VC), and replay attacks. Additionally, we also review integrated solutions to voice spoofing evaluation and speaker verification, adversarial and anti-forensics attacks on voice countermeasures, and ASV. The limitations and challenges of the existing spoofing countermeasures are also presented. We report the performance of these countermeasures on several datasets and evaluate them across corpora. For the experiments, we employ the ASVspoof2019 and VSDC datasets along with GMM, SVM, CNN, and CNN-GRU classifiers. (For reproduceability of the results, the code of the test bed can be found in our GitHub Repository.
PDF
点此查看论文截图
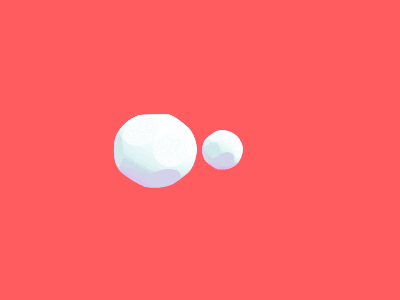
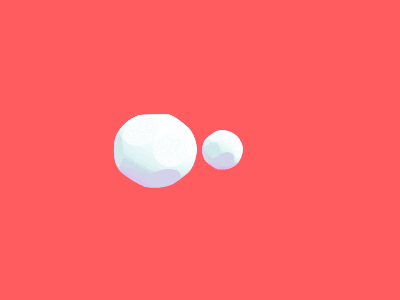
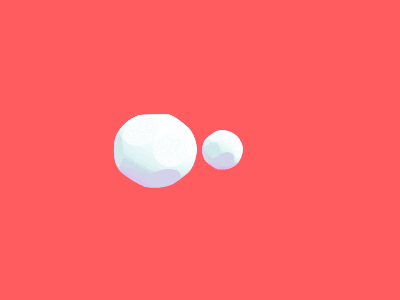
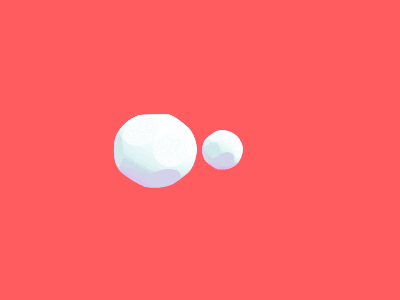
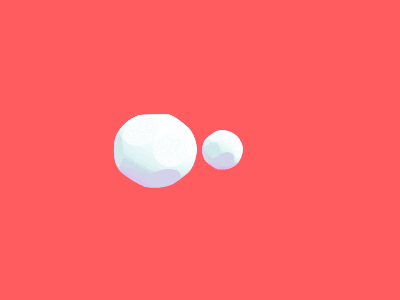
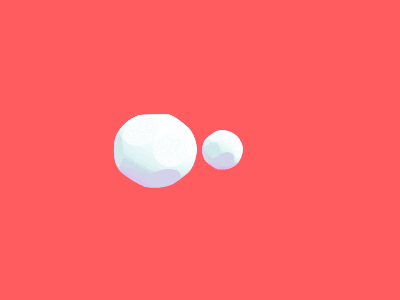
SAMO: Speaker Attractor Multi-Center One-Class Learning for Voice Anti-Spoofing
Authors:Siwen Ding, You Zhang, Zhiyao Duan
Voice anti-spoofing systems are crucial auxiliaries for automatic speaker verification (ASV) systems. A major challenge is caused by unseen attacks empowered by advanced speech synthesis technologies. Our previous research on one-class learning has improved the generalization ability to unseen attacks by compacting the bona fide speech in the embedding space. However, such compactness lacks consideration of the diversity of speakers. In this work, we propose speaker attractor multi-center one-class learning (SAMO), which clusters bona fide speech around a number of speaker attractors and pushes away spoofing attacks from all the attractors in a high-dimensional embedding space. For training, we propose an algorithm for the co-optimization of bona fide speech clustering and bona fide/spoof classification. For inference, we propose strategies to enable anti-spoofing for speakers without enrollment. Our proposed system outperforms existing state-of-the-art single systems with a relative improvement of 38% on equal error rate (EER) on the ASVspoof2019 LA evaluation set.
PDF
点此查看论文截图
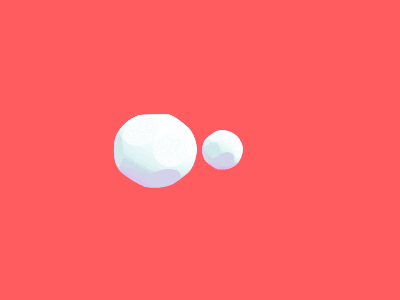
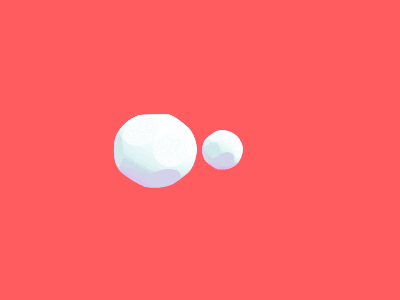
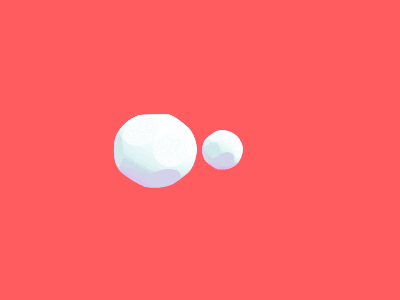
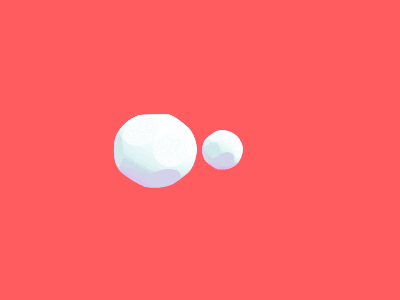
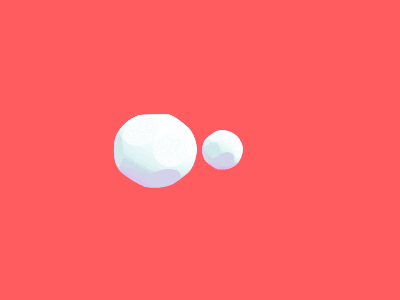
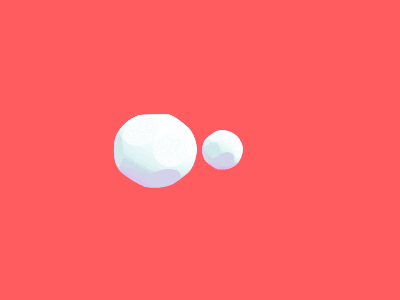
Robust Face Anti-Spoofing with Dual Probabilistic Modeling
Authors:Yuanhan Zhang, Yichao Wu, Zhenfei Yin, Jing Shao, Ziwei Liu
The field of face anti-spoofing (FAS) has witnessed great progress with the surge of deep learning. Due to its data-driven nature, existing FAS methods are sensitive to the noise in the dataset, which will hurdle the learning process. However, very few works consider noise modeling in FAS. In this work, we attempt to fill this gap by automatically addressing the noise problem from both label and data perspectives in a probabilistic manner. Specifically, we propose a unified framework called Dual Probabilistic Modeling (DPM), with two dedicated modules, DPM-LQ (Label Quality aware learning) and DPM-DQ (Data Quality aware learning). Both modules are designed based on the assumption that data and label should form coherent probabilistic distributions. DPM-LQ is able to produce robust feature representations without overfitting to the distribution of noisy semantic labels. DPM-DQ can eliminate data noise from False Reject' and
False Accept’ during inference by correcting the prediction confidence of noisy data based on its quality distribution. Both modules can be incorporated into existing deep networks seamlessly and efficiently. Furthermore, we propose the generalized DPM to address the noise problem in practical usage without the need of semantic annotations. Extensive experiments demonstrate that this probabilistic modeling can 1) significantly improve the accuracy, and 2) make the model robust to the noise in real-world datasets. Without bells and whistles, our proposed DPM achieves state-of-the-art performance on multiple standard FAS benchmarks.
PDF
点此查看论文截图
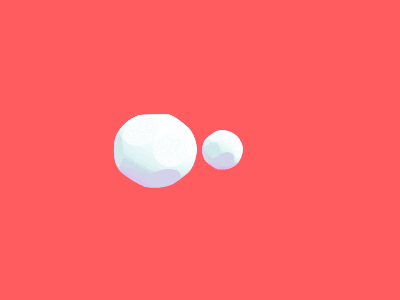
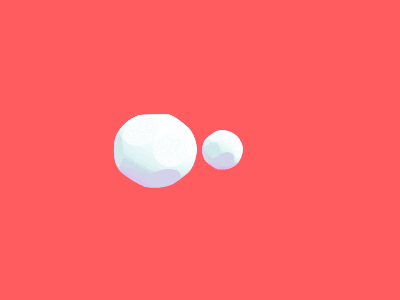
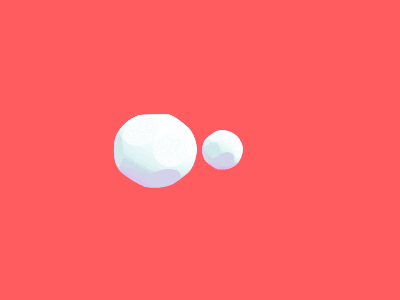
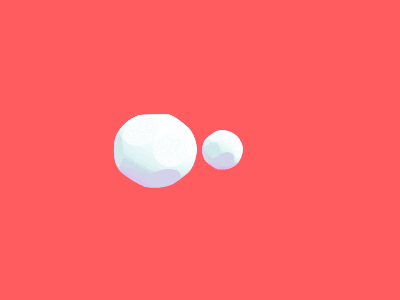
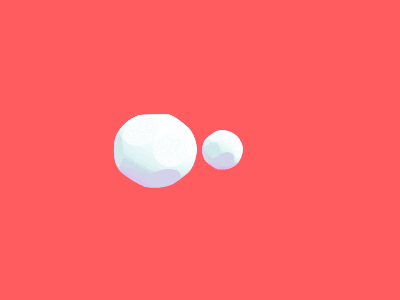
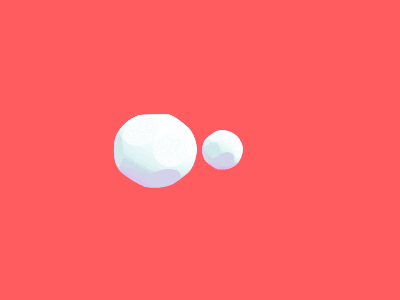
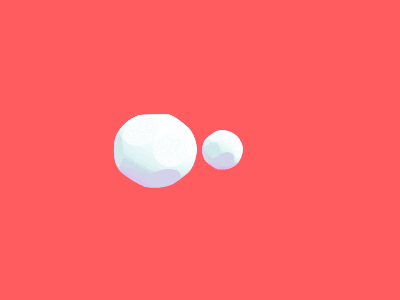
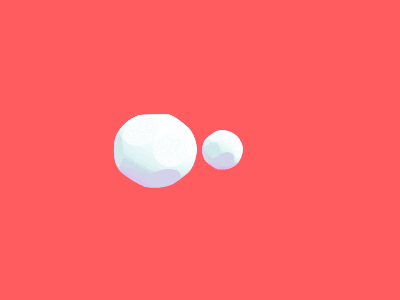