2022-10-11 更新
LAPFormer: A Light and Accurate Polyp Segmentation Transformer
Authors:Mai Nguyen, Tung Thanh Bui, Quan Van Nguyen, Thanh Tung Nguyen, Toan Van Pham
Polyp segmentation is still known as a difficult problem due to the large variety of polyp shapes, scanning and labeling modalities. This prevents deep learning model to generalize well on unseen data. However, Transformer-based approach recently has achieved some remarkable results on performance with the ability of extracting global context better than CNN-based architecture and yet lead to better generalization. To leverage this strength of Transformer, we propose a new model with encoder-decoder architecture named LAPFormer, which uses a hierarchical Transformer encoder to better extract global feature and combine with our novel CNN (Convolutional Neural Network) decoder for capturing local appearance of the polyps. Our proposed decoder contains a progressive feature fusion module designed for fusing feature from upper scales and lower scales and enable multi-scale features to be more correlative. Besides, we also use feature refinement module and feature selection module for processing feature. We test our model on five popular benchmark datasets for polyp segmentation, including Kvasir, CVC-Clinic DB, CVC-ColonDB, CVC-T, and ETIS-Larib
PDF 7 pages, 7 figures, ACL 2023 underreview
点此查看论文截图
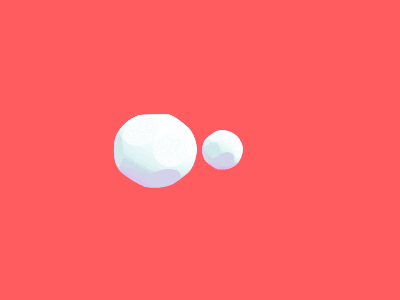
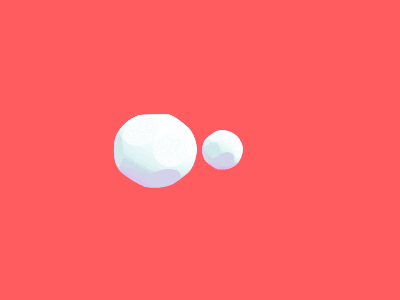
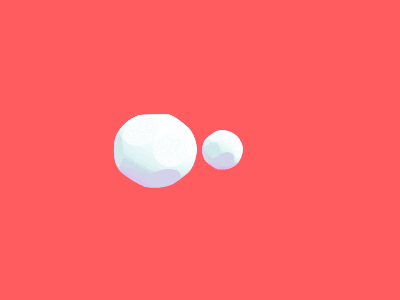
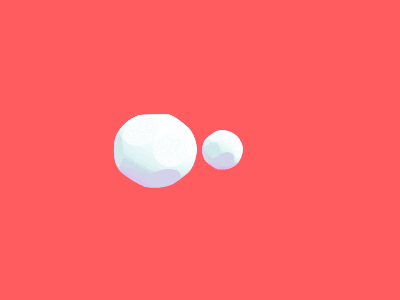
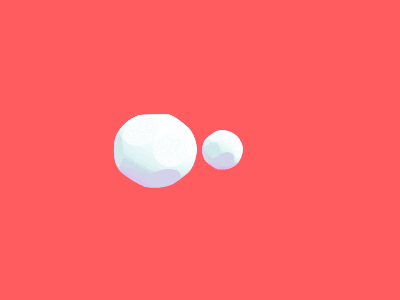