2022-09-29 更新
Weighted Contrastive Hashing
Authors:Jiaguo Yu, Huming Qiu, Dubing Chen, Haofeng Zhang
The development of unsupervised hashing is advanced by the recent popular contrastive learning paradigm. However, previous contrastive learning-based works have been hampered by (1) insufficient data similarity mining based on global-only image representations, and (2) the hash code semantic loss caused by the data augmentation. In this paper, we propose a novel method, namely Weighted Contrative Hashing (WCH), to take a step towards solving these two problems. We introduce a novel mutual attention module to alleviate the problem of information asymmetry in network features caused by the missing image structure during contrative augmentation. Furthermore, we explore the fine-grained semantic relations between images, i.e., we divide the images into multiple patches and calculate similarities between patches. The aggregated weighted similarities, which reflect the deep image relations, are distilled to facilitate the hash codes learning with a distillation loss, so as to obtain better retrieval performance. Extensive experiments show that the proposed WCH significantly outperforms existing unsupervised hashing methods on three benchmark datasets.
PDF
点此查看论文截图
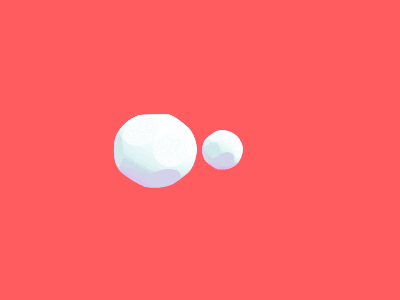
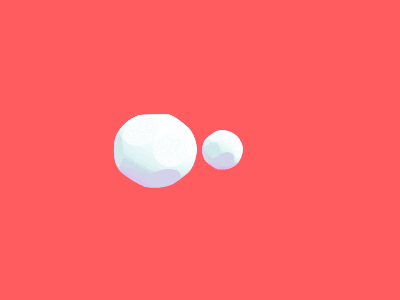
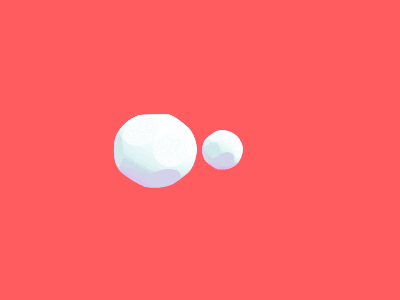
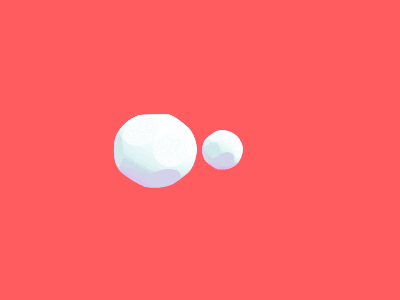
UniCLIP: Unified Framework for Contrastive Language-Image Pre-training
Authors:Janghyeon Lee, Jongsuk Kim, Hyounguk Shon, Bumsoo Kim, Seung Hwan Kim, Honglak Lee, Junmo Kim
Pre-training vision-language models with contrastive objectives has shown promising results that are both scalable to large uncurated datasets and transferable to many downstream applications. Some following works have targeted to improve data efficiency by adding self-supervision terms, but inter-domain (image-text) contrastive loss and intra-domain (image-image) contrastive loss are defined on individual spaces in those works, so many feasible combinations of supervision are overlooked. To overcome this issue, we propose UniCLIP, a Unified framework for Contrastive Language-Image Pre-training. UniCLIP integrates the contrastive loss of both inter-domain pairs and intra-domain pairs into a single universal space. The discrepancies that occur when integrating contrastive loss between different domains are resolved by the three key components of UniCLIP: (1) augmentation-aware feature embedding, (2) MP-NCE loss, and (3) domain dependent similarity measure. UniCLIP outperforms previous vision-language pre-training methods on various single- and multi-modality downstream tasks. In our experiments, we show that each component that comprises UniCLIP contributes well to the final performance.
PDF Neural Information Processing Systems (NeurIPS) 2022
点此查看论文截图
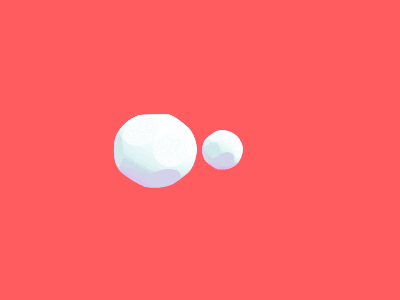
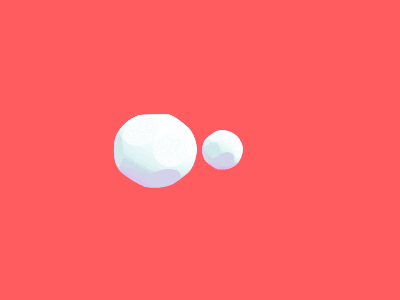
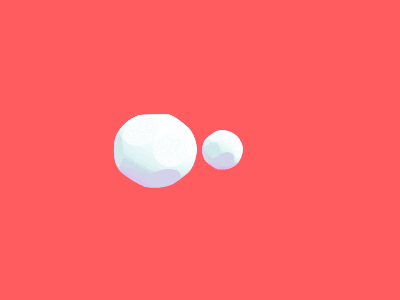
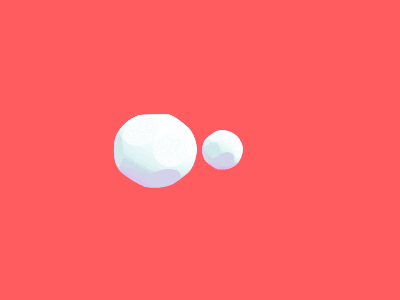
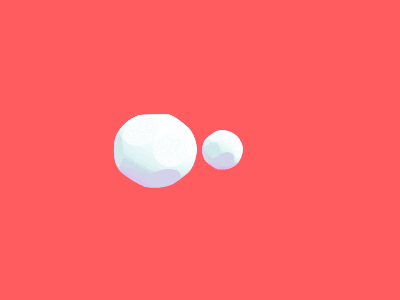
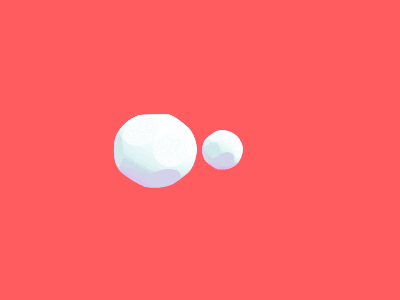
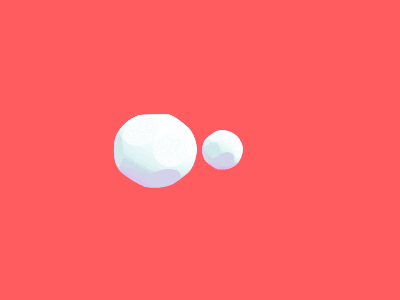
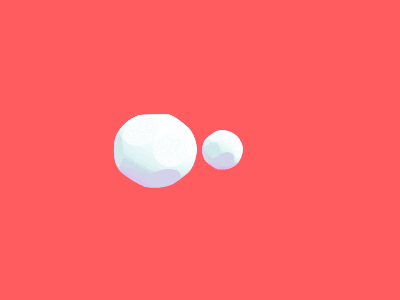
FreeSeg: Free Mask from Interpretable Contrastive Language-Image Pretraining for Semantic Segmentation
Authors:Yi Li, Huifeng Yao, Hualiang Wang, Xiaomeng Li
Fully supervised semantic segmentation learns from dense masks, which requires heavy annotation cost for closed set. In this paper, we use natural language as supervision without any pixel-level annotation for open world segmentation. We call the proposed framework as FreeSeg, where the mask is freely available from raw feature map of pretraining model. Compared with zero-shot or openset segmentation, FreeSeg doesn’t require any annotated masks, and it widely predicts categories beyond class-agnostic unsupervised segmentation. Specifically, FreeSeg obtains free mask from Image-Text Similarity Map (ITSM) of Interpretable Contrastive Language-Image Pretraining (ICLIP). And our core improvements are the smoothed min pooling for dense ICLIP, with the partial label and pixel strategies for segmentation. Furthermore, FreeSeg is very straight forward without complex design like grouping, clustering or retrieval. Besides the simplicity, the performances of FreeSeg surpass previous state-of-the-art at large margins, e.g. 13.4% higher at mIoU on VOC dataset in the same settings.
PDF Conference paper, segmentation via ICLIP
点此查看论文截图
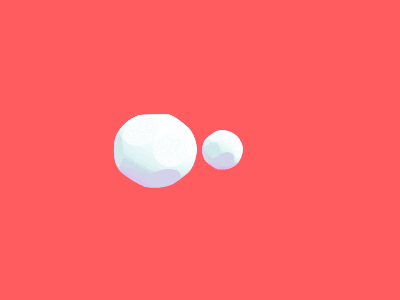
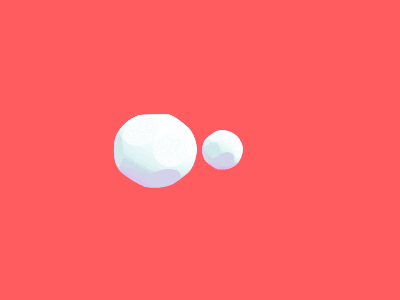
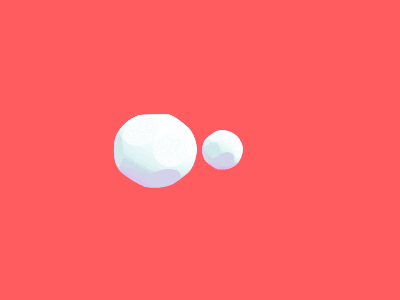
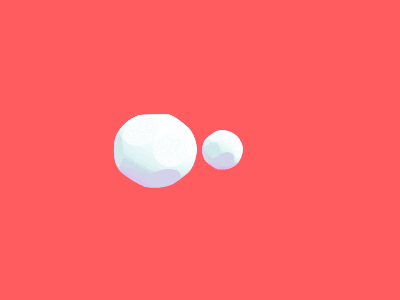
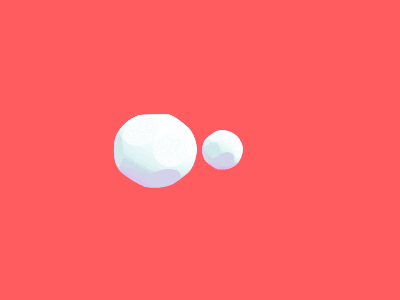
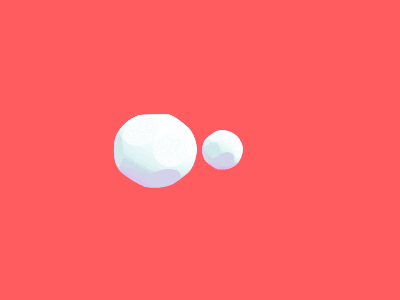
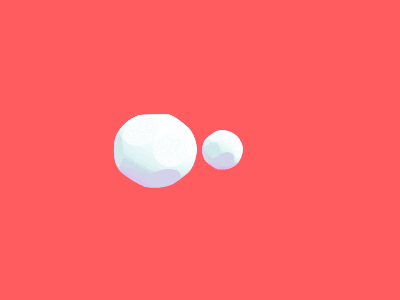
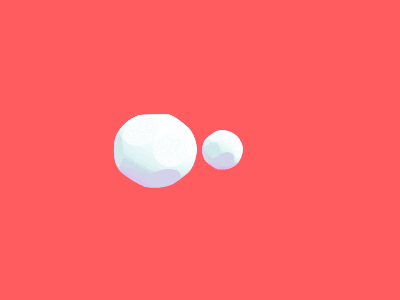