2024-04-14 更新
UDiFF: Generating Conditional Unsigned Distance Fields with Optimal Wavelet Diffusion
Authors:Junsheng Zhou, Weiqi Zhang, Baorui Ma, Kanle Shi, Yu-Shen Liu, Zhizhong Han
Diffusion models have shown remarkable results for image generation, editing and inpainting. Recent works explore diffusion models for 3D shape generation with neural implicit functions, i.e., signed distance function and occupancy function. However, they are limited to shapes with closed surfaces, which prevents them from generating diverse 3D real-world contents containing open surfaces. In this work, we present UDiFF, a 3D diffusion model for unsigned distance fields (UDFs) which is capable to generate textured 3D shapes with open surfaces from text conditions or unconditionally. Our key idea is to generate UDFs in spatial-frequency domain with an optimal wavelet transformation, which produces a compact representation space for UDF generation. Specifically, instead of selecting an appropriate wavelet transformation which requires expensive manual efforts and still leads to large information loss, we propose a data-driven approach to learn the optimal wavelet transformation for UDFs. We evaluate UDiFF to show our advantages by numerical and visual comparisons with the latest methods on widely used benchmarks. Page: https://weiqi-zhang.github.io/UDiFF.
PDF To appear at CVPR2024. Project page: https://weiqi-zhang.github.io/UDiFF
点此查看论文截图
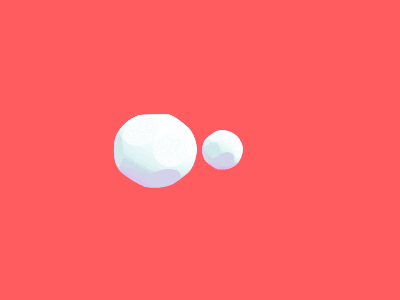
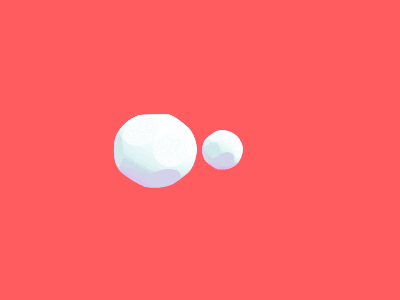
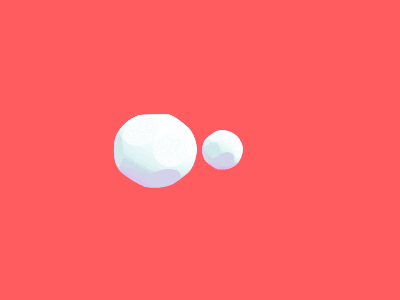
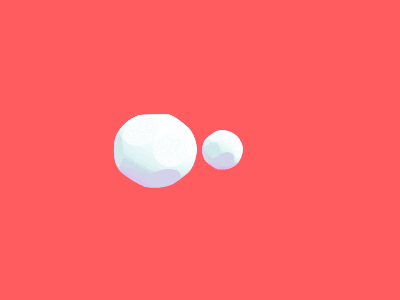
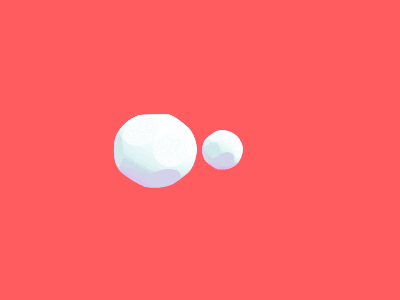
DreamScene360: Unconstrained Text-to-3D Scene Generation with Panoramic Gaussian Splatting
Authors:Shijie Zhou, Zhiwen Fan, Dejia Xu, Haoran Chang, Pradyumna Chari, Tejas Bharadwaj, Suya You, Zhangyang Wang, Achuta Kadambi
The increasing demand for virtual reality applications has highlighted the significance of crafting immersive 3D assets. We present a text-to-3D 360$^{\circ}$ scene generation pipeline that facilitates the creation of comprehensive 360$^{\circ}$ scenes for in-the-wild environments in a matter of minutes. Our approach utilizes the generative power of a 2D diffusion model and prompt self-refinement to create a high-quality and globally coherent panoramic image. This image acts as a preliminary “flat” (2D) scene representation. Subsequently, it is lifted into 3D Gaussians, employing splatting techniques to enable real-time exploration. To produce consistent 3D geometry, our pipeline constructs a spatially coherent structure by aligning the 2D monocular depth into a globally optimized point cloud. This point cloud serves as the initial state for the centroids of 3D Gaussians. In order to address invisible issues inherent in single-view inputs, we impose semantic and geometric constraints on both synthesized and input camera views as regularizations. These guide the optimization of Gaussians, aiding in the reconstruction of unseen regions. In summary, our method offers a globally consistent 3D scene within a 360$^{\circ}$ perspective, providing an enhanced immersive experience over existing techniques. Project website at: http://dreamscene360.github.io/
PDF
点此查看论文截图
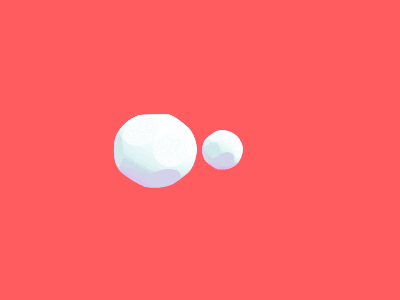
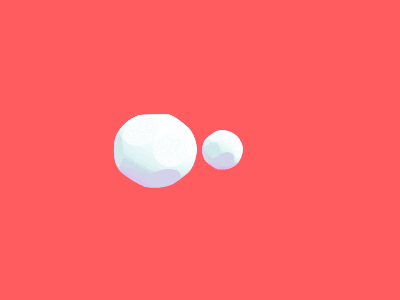
Diffusion-based inpainting of incomplete Euclidean distance matrices of trajectories generated by a fractional Brownian motion
Authors:Alexander Lobashev, Kirill Polovnikov
Fractional Brownian trajectories (fBm) feature both randomness and strong scale-free correlations, challenging generative models to reproduce the intrinsic memory characterizing the underlying process. Here we test a diffusion probabilistic model on a specific dataset of corrupted images corresponding to incomplete Euclidean distance matrices of fBm at various memory exponents $H$. Our dataset implies uniqueness of the data imputation in the regime of low missing ratio, where the remaining partial graph is rigid, providing the ground truth for the inpainting. We find that the conditional diffusion generation stably reproduces the statistics of missing fBm-distributed distances for different values of $H$ exponent. Furthermore, while diffusion models have been recently shown to remember samples from the training database, we show that diffusion-based inpainting behaves qualitatively different from the database search with the increasing database size. Finally, we apply our fBm-trained diffusion model with $H=1/3$ for completion of chromosome distance matrices obtained in single-cell microscopy experiments, showing its superiority over the standard bioinformatics algorithms. Our source code is available on GitHub at https://github.com/alobashev/diffusion_fbm.
PDF
点此查看论文截图
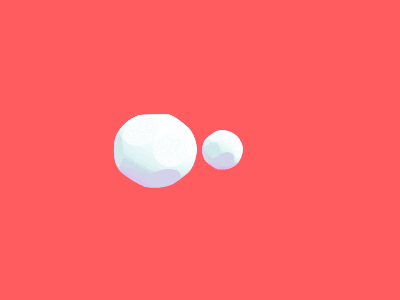
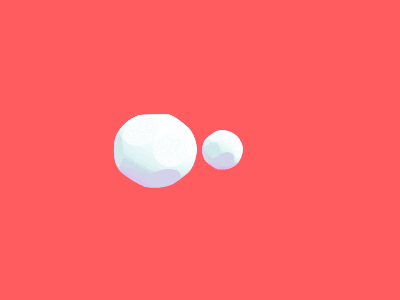
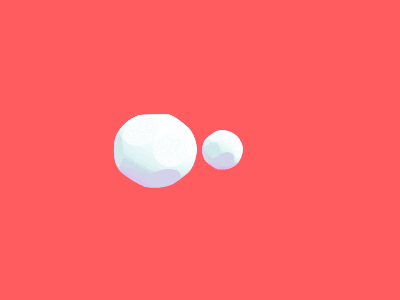
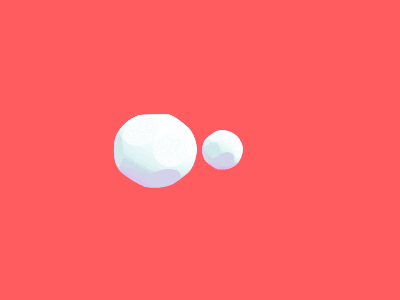
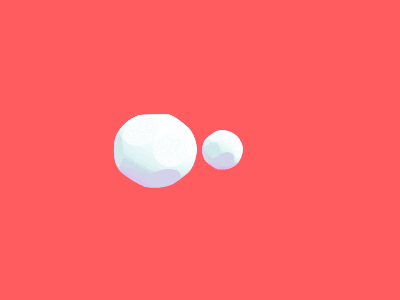
InstantMesh: Efficient 3D Mesh Generation from a Single Image with Sparse-view Large Reconstruction Models
Authors:Jiale Xu, Weihao Cheng, Yiming Gao, Xintao Wang, Shenghua Gao, Ying Shan
We present InstantMesh, a feed-forward framework for instant 3D mesh generation from a single image, featuring state-of-the-art generation quality and significant training scalability. By synergizing the strengths of an off-the-shelf multiview diffusion model and a sparse-view reconstruction model based on the LRM architecture, InstantMesh is able to create diverse 3D assets within 10 seconds. To enhance the training efficiency and exploit more geometric supervisions, e.g, depths and normals, we integrate a differentiable iso-surface extraction module into our framework and directly optimize on the mesh representation. Experimental results on public datasets demonstrate that InstantMesh significantly outperforms other latest image-to-3D baselines, both qualitatively and quantitatively. We release all the code, weights, and demo of InstantMesh, with the intention that it can make substantial contributions to the community of 3D generative AI and empower both researchers and content creators.
PDF Technical report. Project: https://github.com/TencentARC/InstantMesh
点此查看论文截图
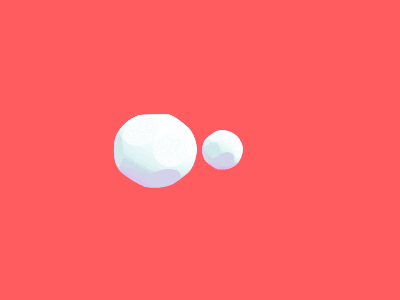
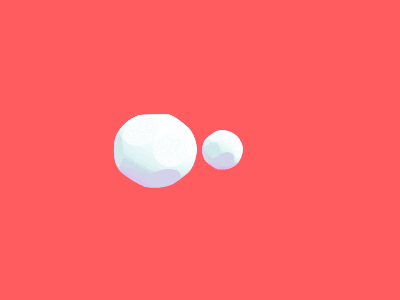
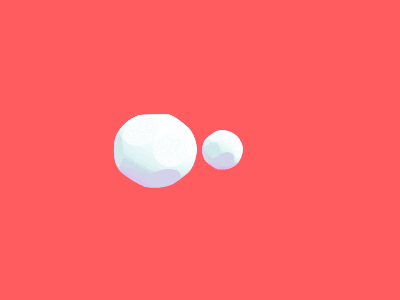
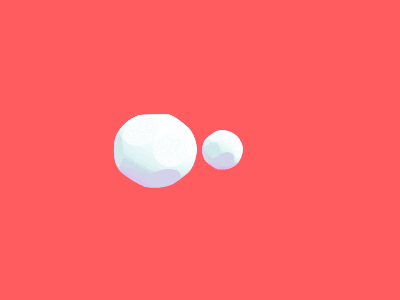
RealmDreamer: Text-Driven 3D Scene Generation with Inpainting and Depth Diffusion
Authors:Jaidev Shriram, Alex Trevithick, Lingjie Liu, Ravi Ramamoorthi
We introduce RealmDreamer, a technique for generation of general forward-facing 3D scenes from text descriptions. Our technique optimizes a 3D Gaussian Splatting representation to match complex text prompts. We initialize these splats by utilizing the state-of-the-art text-to-image generators, lifting their samples into 3D, and computing the occlusion volume. We then optimize this representation across multiple views as a 3D inpainting task with image-conditional diffusion models. To learn correct geometric structure, we incorporate a depth diffusion model by conditioning on the samples from the inpainting model, giving rich geometric structure. Finally, we finetune the model using sharpened samples from image generators. Notably, our technique does not require video or multi-view data and can synthesize a variety of high-quality 3D scenes in different styles, consisting of multiple objects. Its generality additionally allows 3D synthesis from a single image.
PDF Project Page: https://realmdreamer.github.io/
点此查看论文截图
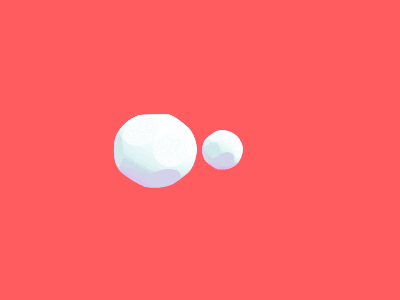
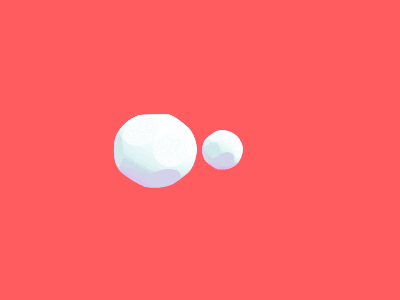
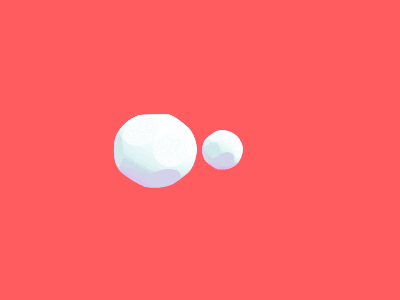
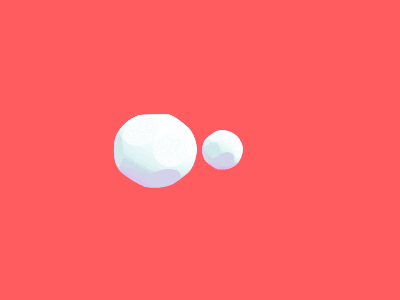
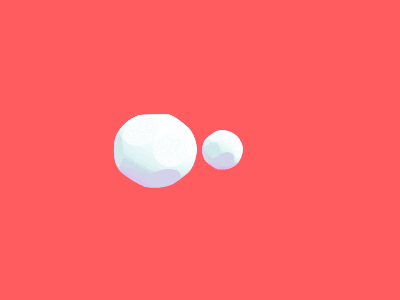
GoodDrag: Towards Good Practices for Drag Editing with Diffusion Models
Authors:Zewei Zhang, Huan Liu, Jun Chen, Xiangyu Xu
In this paper, we introduce GoodDrag, a novel approach to improve the stability and image quality of drag editing. Unlike existing methods that struggle with accumulated perturbations and often result in distortions, GoodDrag introduces an AlDD framework that alternates between drag and denoising operations within the diffusion process, effectively improving the fidelity of the result. We also propose an information-preserving motion supervision operation that maintains the original features of the starting point for precise manipulation and artifact reduction. In addition, we contribute to the benchmarking of drag editing by introducing a new dataset, Drag100, and developing dedicated quality assessment metrics, Dragging Accuracy Index and Gemini Score, utilizing Large Multimodal Models. Extensive experiments demonstrate that the proposed GoodDrag compares favorably against the state-of-the-art approaches both qualitatively and quantitatively. The project page is https://gooddrag.github.io.
PDF
点此查看论文截图
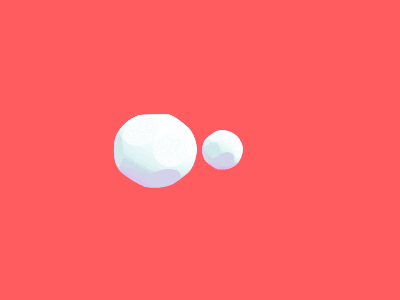
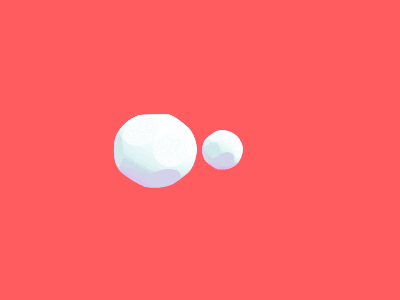
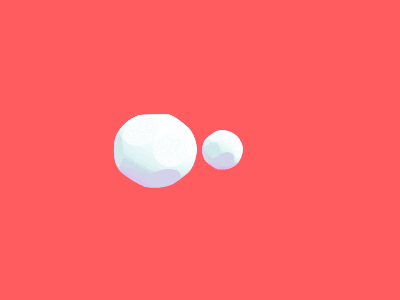
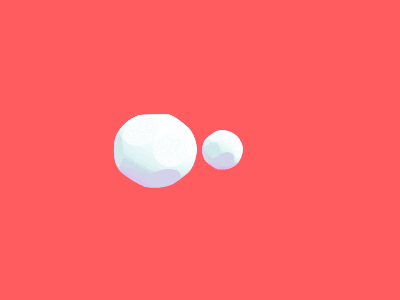
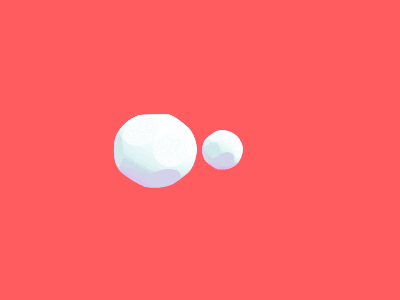
Solving Masked Jigsaw Puzzles with Diffusion Vision Transformers
Authors:Jinyang Liu, Wondmgezahu Teshome, Sandesh Ghimire, Mario Sznaier, Octavia Camps
Solving image and video jigsaw puzzles poses the challenging task of rearranging image fragments or video frames from unordered sequences to restore meaningful images and video sequences. Existing approaches often hinge on discriminative models tasked with predicting either the absolute positions of puzzle elements or the permutation actions applied to the original data. Unfortunately, these methods face limitations in effectively solving puzzles with a large number of elements. In this paper, we propose JPDVT, an innovative approach that harnesses diffusion transformers to address this challenge. Specifically, we generate positional information for image patches or video frames, conditioned on their underlying visual content. This information is then employed to accurately assemble the puzzle pieces in their correct positions, even in scenarios involving missing pieces. Our method achieves state-of-the-art performance on several datasets.
PDF 8 pages, 7 figures
点此查看论文截图
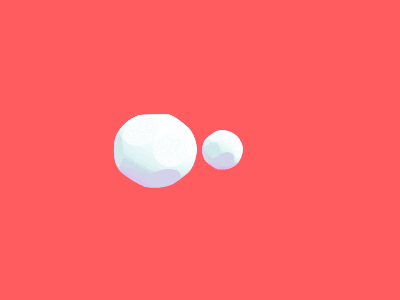
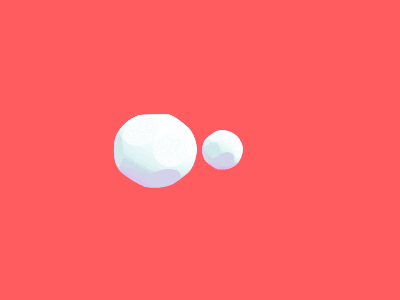
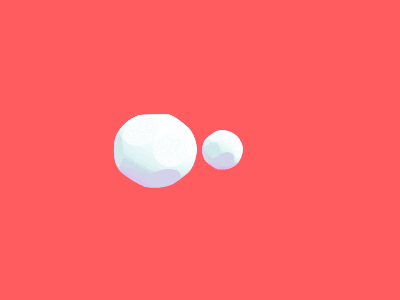
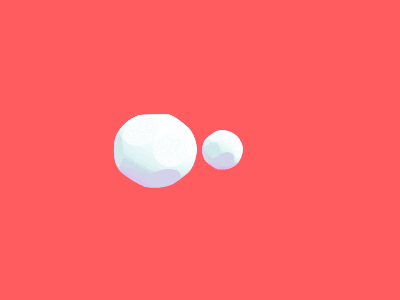
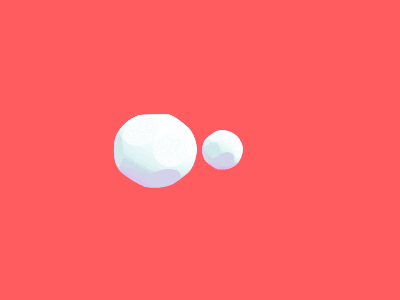
ObjBlur: A Curriculum Learning Approach With Progressive Object-Level Blurring for Improved Layout-to-Image Generation
Authors:Stanislav Frolov, Brian B. Moser, Sebastian Palacio, Andreas Dengel
We present ObjBlur, a novel curriculum learning approach to improve layout-to-image generation models, where the task is to produce realistic images from layouts composed of boxes and labels. Our method is based on progressive object-level blurring, which effectively stabilizes training and enhances the quality of generated images. This curriculum learning strategy systematically applies varying degrees of blurring to individual objects or the background during training, starting from strong blurring to progressively cleaner images. Our findings reveal that this approach yields significant performance improvements, stabilized training, smoother convergence, and reduced variance between multiple runs. Moreover, our technique demonstrates its versatility by being compatible with generative adversarial networks and diffusion models, underlining its applicability across various generative modeling paradigms. With ObjBlur, we reach new state-of-the-art results on the complex COCO and Visual Genome datasets.
PDF
点此查看论文截图
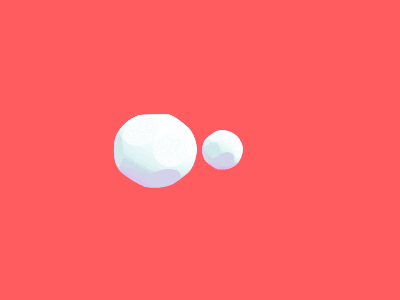
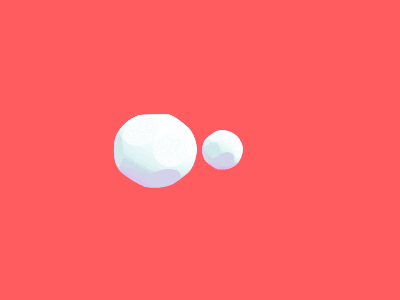
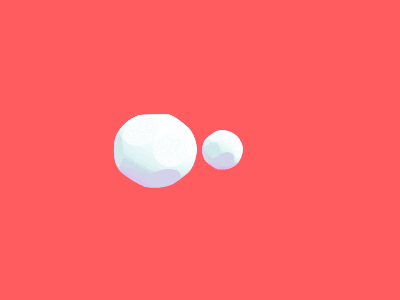
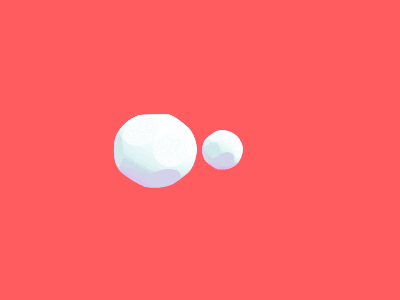
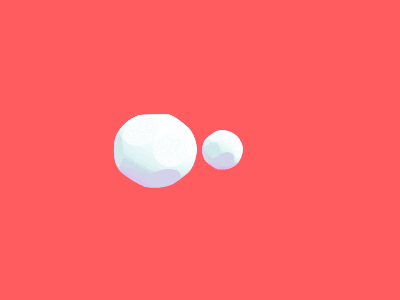
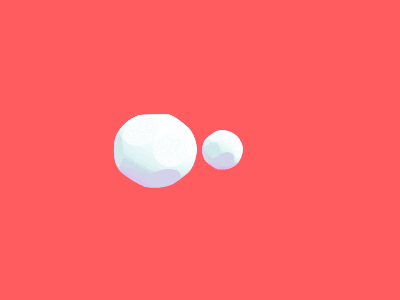
Implicit and Explicit Language Guidance for Diffusion-based Visual Perception
Authors:Hefeng Wang, Jiale Cao, Jin Xie, Aiping Yang, Yanwei Pang
Text-to-image diffusion models have shown powerful ability on conditional image synthesis. With large-scale vision-language pre-training, diffusion models are able to generate high-quality images with rich texture and reasonable structure under different text prompts. However, it is an open problem to adapt the pre-trained diffusion model for visual perception. In this paper, we propose an implicit and explicit language guidance framework for diffusion-based perception, named IEDP. Our IEDP comprises of an implicit language guidance branch and an explicit language guidance branch. The implicit branch employs frozen CLIP image encoder to directly generate implicit text embeddings that are fed to diffusion model, without using explicit text prompts. The explicit branch utilizes the ground-truth labels of corresponding images as text prompts to condition feature extraction of diffusion model. During training, we jointly train diffusion model by sharing the model weights of these two branches. As a result, implicit and explicit branches can jointly guide feature learning. During inference, we only employ implicit branch for final prediction, which does not require any ground-truth labels. Experiments are performed on two typical perception tasks, including semantic segmentation and depth estimation. Our IEDP achieves promising performance on both tasks. For semantic segmentation, our IEDP has the mIoU score of 55.9% on AD20K validation set, which outperforms the baseline method VPD by 2.2%. For depth estimation, our IEDP outperforms the baseline method VPD with a relative gain of 10.2%.
PDF
点此查看论文截图
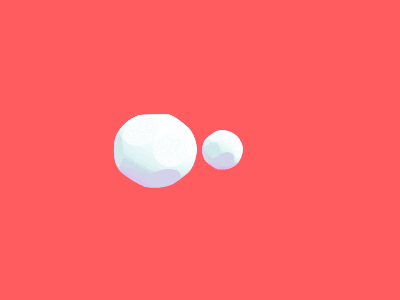
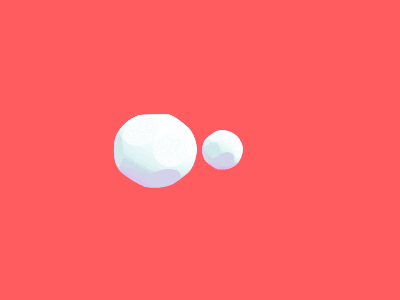
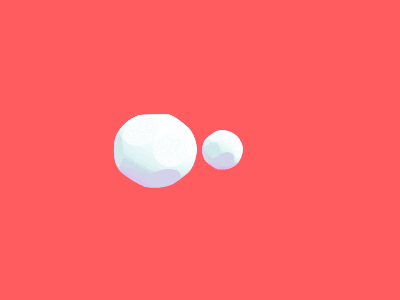
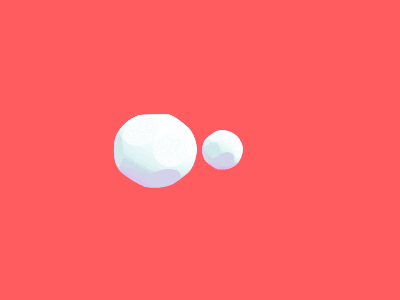
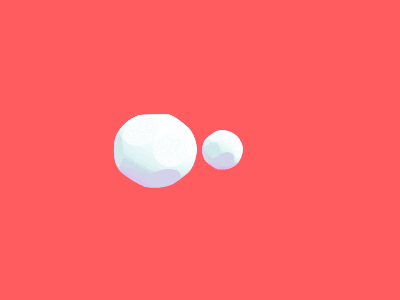
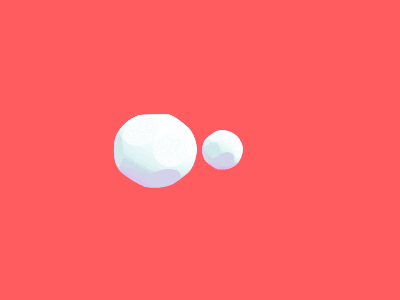
Applying Guidance in a Limited Interval Improves Sample and Distribution Quality in Diffusion Models
Authors:Tuomas Kynkäänniemi, Miika Aittala, Tero Karras, Samuli Laine, Timo Aila, Jaakko Lehtinen
Guidance is a crucial technique for extracting the best performance out of image-generating diffusion models. Traditionally, a constant guidance weight has been applied throughout the sampling chain of an image. We show that guidance is clearly harmful toward the beginning of the chain (high noise levels), largely unnecessary toward the end (low noise levels), and only beneficial in the middle. We thus restrict it to a specific range of noise levels, improving both the inference speed and result quality. This limited guidance interval improves the record FID in ImageNet-512 significantly, from 1.81 to 1.40. We show that it is quantitatively and qualitatively beneficial across different sampler parameters, network architectures, and datasets, including the large-scale setting of Stable Diffusion XL. We thus suggest exposing the guidance interval as a hyperparameter in all diffusion models that use guidance.
PDF
点此查看论文截图
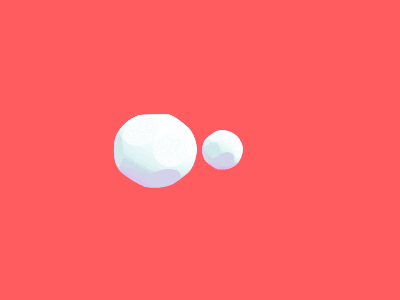
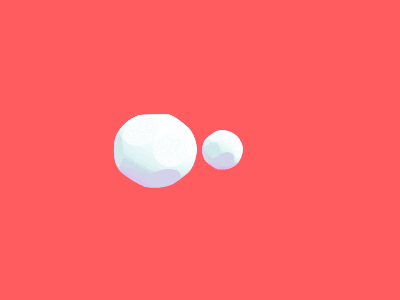
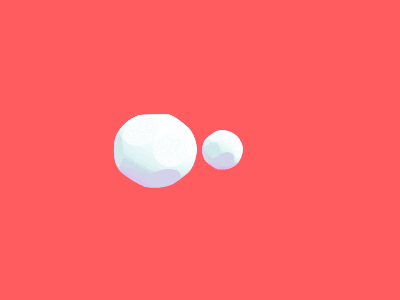
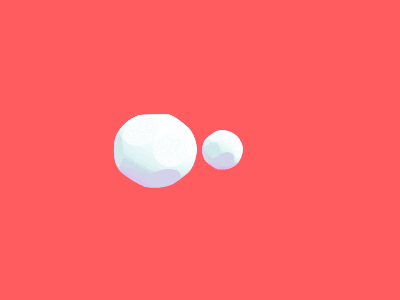
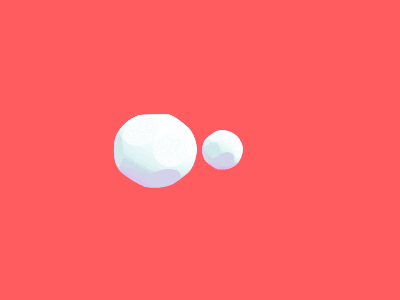
Generating Synthetic Satellite Imagery With Deep-Learning Text-to-Image Models — Technical Challenges and Implications for Monitoring and Verification
Authors:Tuong Vy Nguyen, Alexander Glaser, Felix Biessmann
Novel deep-learning (DL) architectures have reached a level where they can generate digital media, including photorealistic images, that are difficult to distinguish from real data. These technologies have already been used to generate training data for Machine Learning (ML) models, and large text-to-image models like DALL-E 2, Imagen, and Stable Diffusion are achieving remarkable results in realistic high-resolution image generation. Given these developments, issues of data authentication in monitoring and verification deserve a careful and systematic analysis: How realistic are synthetic images? How easily can they be generated? How useful are they for ML researchers, and what is their potential for Open Science? In this work, we use novel DL models to explore how synthetic satellite images can be created using conditioning mechanisms. We investigate the challenges of synthetic satellite image generation and evaluate the results based on authenticity and state-of-the-art metrics. Furthermore, we investigate how synthetic data can alleviate the lack of data in the context of ML methods for remote-sensing. Finally we discuss implications of synthetic satellite imagery in the context of monitoring and verification.
PDF https://resources.inmm.org/annual-meeting-proceedings/generating-synthetic-satellite-imagery-deep-learning-text-image-models
点此查看论文截图
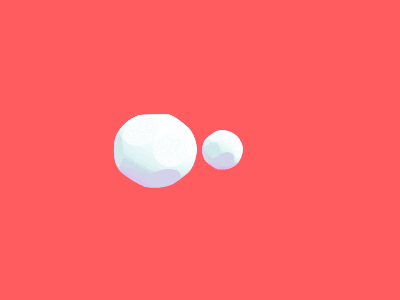
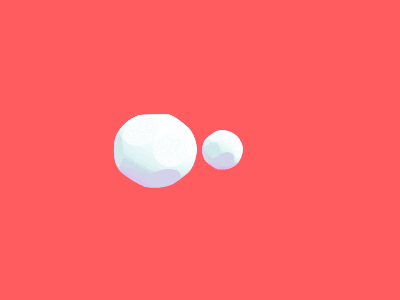
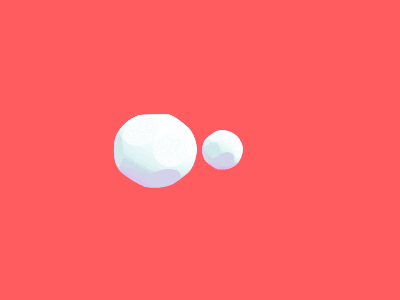
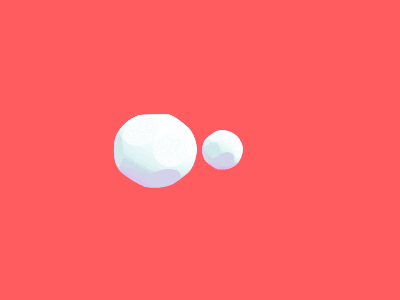
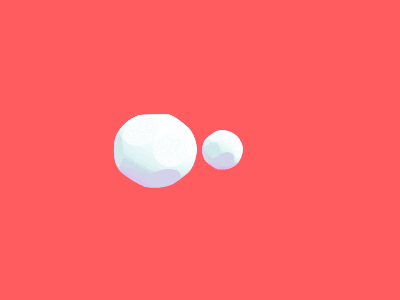
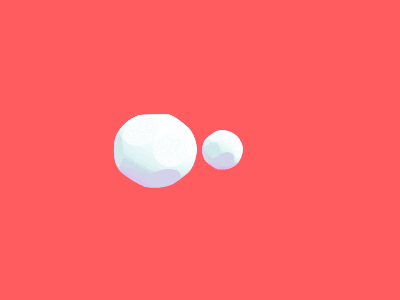
Joint Conditional Diffusion Model for Image Restoration with Mixed Degradations
Authors:Yufeng Yue, Meng Yu, Luojie Yang, Yi Yang
Image restoration is rather challenging in adverse weather conditions, especially when multiple degradations occur simultaneously. Blind image decomposition was proposed to tackle this issue, however, its effectiveness heavily relies on the accurate estimation of each component. Although diffusion-based models exhibit strong generative abilities in image restoration tasks, they may generate irrelevant contents when the degraded images are severely corrupted. To address these issues, we leverage physical constraints to guide the whole restoration process, where a mixed degradation model based on atmosphere scattering model is constructed. Then we formulate our Joint Conditional Diffusion Model (JCDM) by incorporating the degraded image and degradation mask to provide precise guidance. To achieve better color and detail recovery results, we further integrate a refinement network to reconstruct the restored image, where Uncertainty Estimation Block (UEB) is employed to enhance the features. Extensive experiments performed on both multi-weather and weather-specific datasets demonstrate the superiority of our method over state-of-the-art competing methods.
PDF
点此查看论文截图
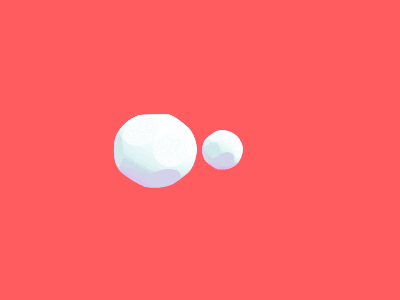
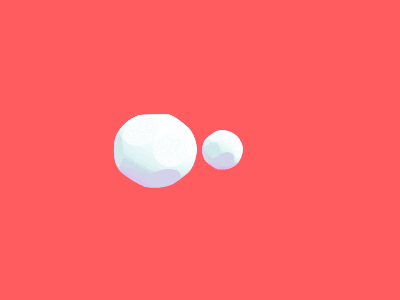
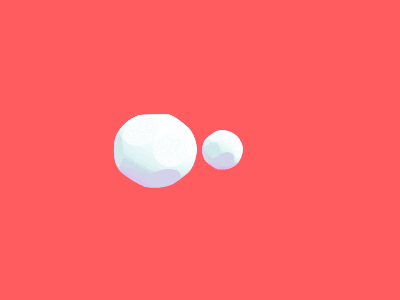
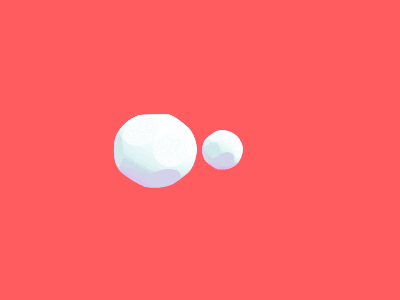
Taming Stable Diffusion for Text to 360° Panorama Image Generation
Authors:Cheng Zhang, Qianyi Wu, Camilo Cruz Gambardella, Xiaoshui Huang, Dinh Phung, Wanli Ouyang, Jianfei Cai
Generative models, e.g., Stable Diffusion, have enabled the creation of photorealistic images from text prompts. Yet, the generation of 360-degree panorama images from text remains a challenge, particularly due to the dearth of paired text-panorama data and the domain gap between panorama and perspective images. In this paper, we introduce a novel dual-branch diffusion model named PanFusion to generate a 360-degree image from a text prompt. We leverage the stable diffusion model as one branch to provide prior knowledge in natural image generation and register it to another panorama branch for holistic image generation. We propose a unique cross-attention mechanism with projection awareness to minimize distortion during the collaborative denoising process. Our experiments validate that PanFusion surpasses existing methods and, thanks to its dual-branch structure, can integrate additional constraints like room layout for customized panorama outputs. Code is available at https://chengzhag.github.io/publication/panfusion.
PDF CVPR 2024. Project Page: https://chengzhag.github.io/publication/panfusion Code: https://github.com/chengzhag/PanFusion
点此查看论文截图
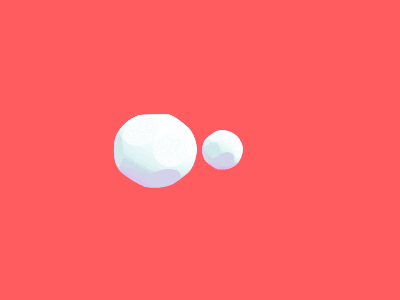
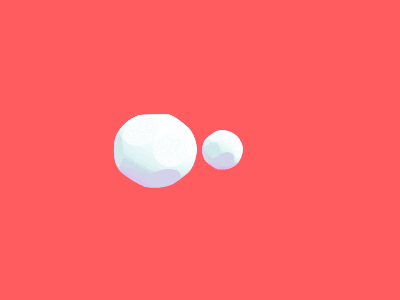
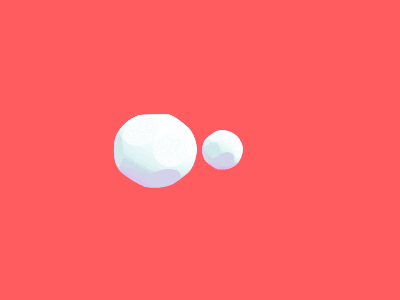
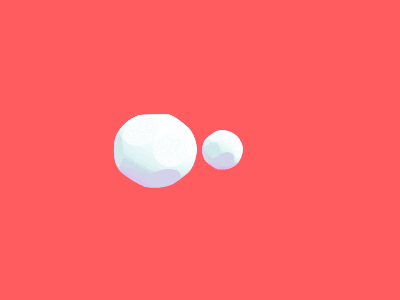
View Selection for 3D Captioning via Diffusion Ranking
Authors:Tiange Luo, Justin Johnson, Honglak Lee
Scalable annotation approaches are crucial for constructing extensive 3D-text datasets, facilitating a broader range of applications. However, existing methods sometimes lead to the generation of hallucinated captions, compromising caption quality. This paper explores the issue of hallucination in 3D object captioning, with a focus on Cap3D method, which renders 3D objects into 2D views for captioning using pre-trained models. We pinpoint a major challenge: certain rendered views of 3D objects are atypical, deviating from the training data of standard image captioning models and causing hallucinations. To tackle this, we present DiffuRank, a method that leverages a pre-trained text-to-3D model to assess the alignment between 3D objects and their 2D rendered views, where the view with high alignment closely represent the object’s characteristics. By ranking all rendered views and feeding the top-ranked ones into GPT4-Vision, we enhance the accuracy and detail of captions, enabling the correction of 200k captions in the Cap3D dataset and extending it to 1 million captions across Objaverse and Objaverse-XL datasets. Additionally, we showcase the adaptability of DiffuRank by applying it to pre-trained text-to-image models for a Visual Question Answering task, where it outperforms the CLIP model.
PDF Dataset link: https://huggingface.co/datasets/tiange/Cap3D
点此查看论文截图
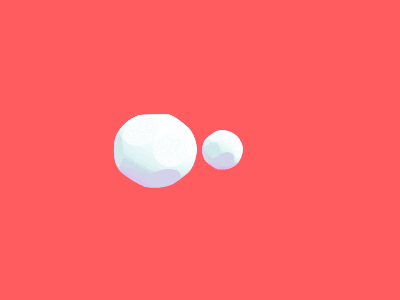
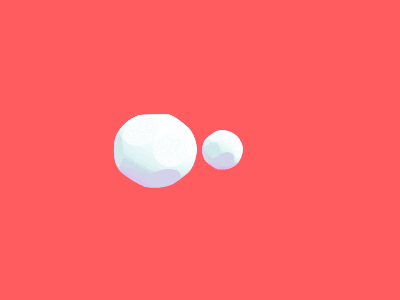
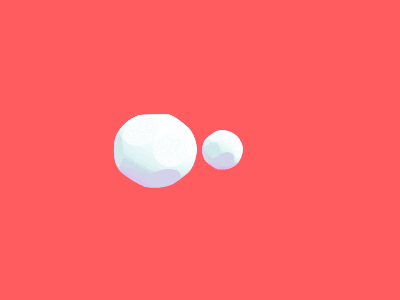
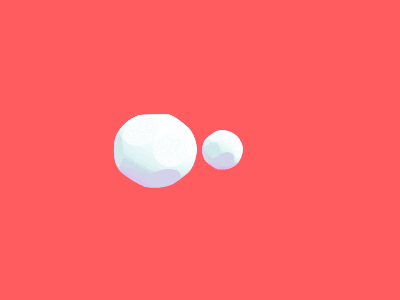
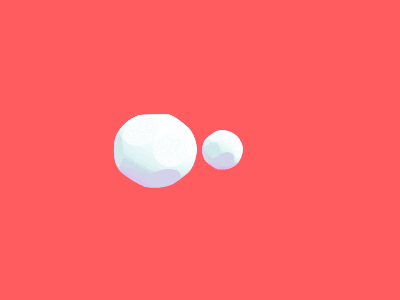