2024-04-01 更新
HGT: Leveraging Heterogeneous Graph-enhanced Large Language Models for Few-shot Complex Table Understanding
Authors:Rihui Jin, Yu Li, Guilin Qi, Nan Hu, Yuan-Fang Li, Jiaoyan Chen, Jianan Wang, Yongrui Chen, Dehai Min
Table understanding (TU) has achieved promising advancements, but it faces the challenges of the scarcity of manually labeled tables and the presence of complex table structures.To address these challenges, we propose HGT, a framework with a heterogeneous graph (HG)-enhanced large language model (LLM) to tackle few-shot TU tasks.It leverages the LLM by aligning the table semantics with the LLM’s parametric knowledge through soft prompts and instruction turning and deals with complex tables by a multi-task pre-training scheme involving three novel multi-granularity self-supervised HG pre-training objectives.We empirically demonstrate the effectiveness of HGT, showing that it outperforms the SOTA for few-shot complex TU on several benchmarks.
PDF
点此查看论文截图
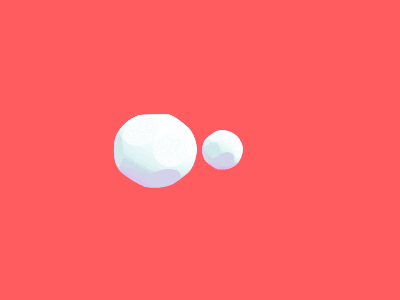
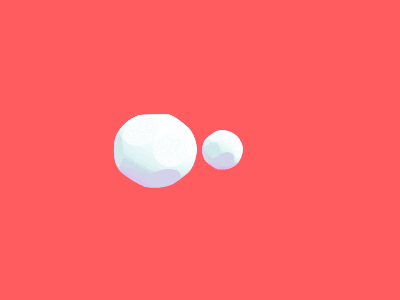
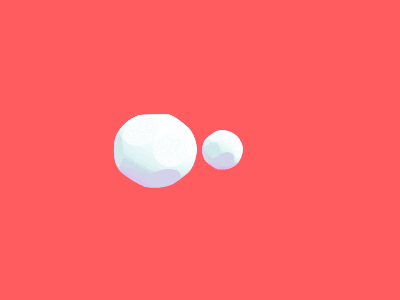
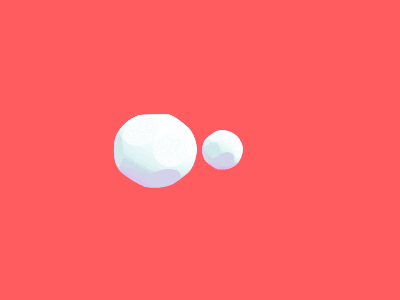
Heterogeneous Network Based Contrastive Learning Method for PolSAR Land Cover Classification
Authors:Jianfeng Cai, Yue Ma, Zhixi Feng, Shuyuan Yang
Polarimetric synthetic aperture radar (PolSAR) image interpretation is widely used in various fields. Recently, deep learning has made significant progress in PolSAR image classification. Supervised learning (SL) requires a large amount of labeled PolSAR data with high quality to achieve better performance, however, manually labeled data is insufficient. This causes the SL to fail into overfitting and degrades its generalization performance. Furthermore, the scattering confusion problem is also a significant challenge that attracts more attention. To solve these problems, this article proposes a Heterogeneous Network based Contrastive Learning method(HCLNet). It aims to learn high-level representation from unlabeled PolSAR data for few-shot classification according to multi-features and superpixels. Beyond the conventional CL, HCLNet introduces the heterogeneous architecture for the first time to utilize heterogeneous PolSAR features better. And it develops two easy-to-use plugins to narrow the domain gap between optics and PolSAR, including feature filter and superpixel-based instance discrimination, which the former is used to enhance the complementarity of multi-features, and the latter is used to increase the diversity of negative samples. Experiments demonstrate the superiority of HCLNet on three widely used PolSAR benchmark datasets compared with state-of-the-art methods. Ablation studies also verify the importance of each component. Besides, this work has implications for how to efficiently utilize the multi-features of PolSAR data to learn better high-level representation in CL and how to construct networks suitable for PolSAR data better.
PDF
点此查看论文截图
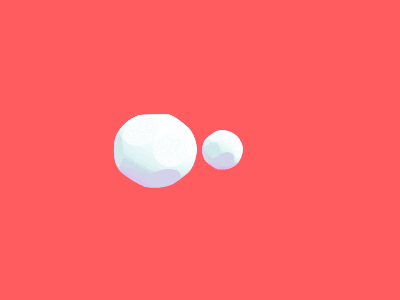
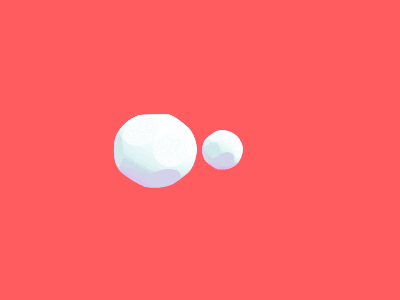
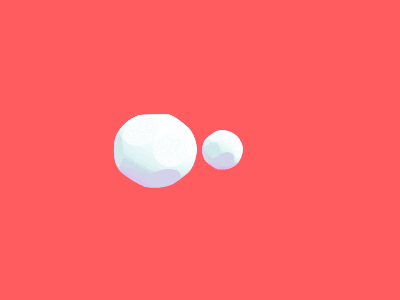
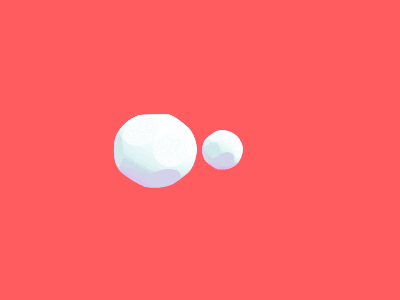
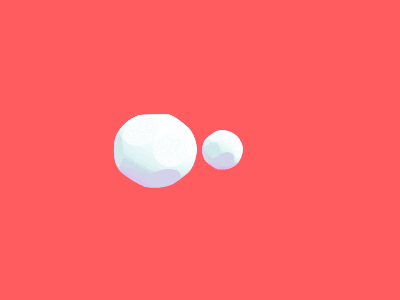
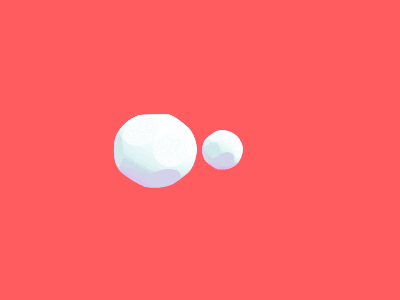
Stable Surface Regularization for Fast Few-Shot NeRF
Authors:Byeongin Joung, Byeong-Uk Lee, Jaesung Choe, Ukcheol Shin, Minjun Kang, Taeyeop Lee, In So Kweon, Kuk-Jin Yoon
This paper proposes an algorithm for synthesizing novel views under few-shot setup. The main concept is to develop a stable surface regularization technique called Annealing Signed Distance Function (ASDF), which anneals the surface in a coarse-to-fine manner to accelerate convergence speed. We observe that the Eikonal loss - which is a widely known geometric regularization - requires dense training signal to shape different level-sets of SDF, leading to low-fidelity results under few-shot training. In contrast, the proposed surface regularization successfully reconstructs scenes and produce high-fidelity geometry with stable training. Our method is further accelerated by utilizing grid representation and monocular geometric priors. Finally, the proposed approach is up to 45 times faster than existing few-shot novel view synthesis methods, and it produces comparable results in the ScanNet dataset and NeRF-Real dataset.
PDF 3DV 2024
点此查看论文截图
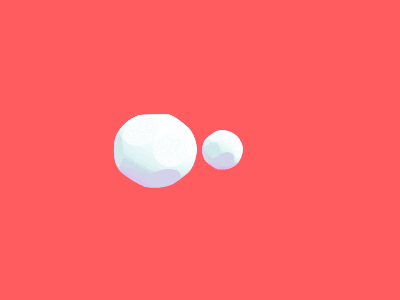
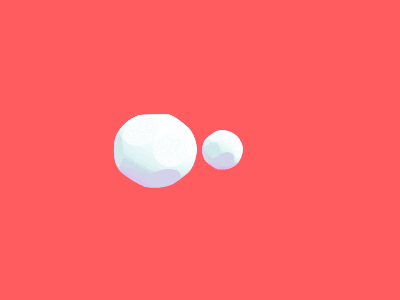
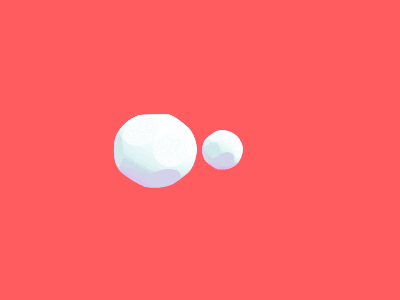
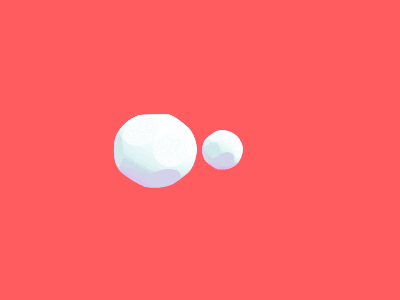
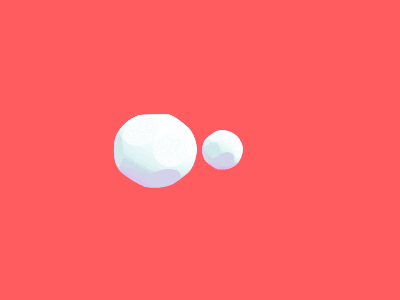
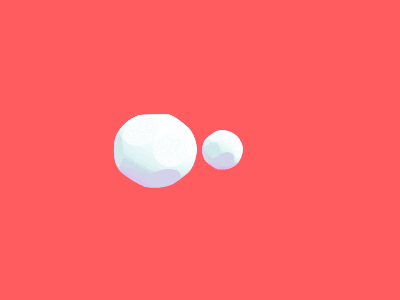
Unleashing the Potential of Large Language Models for Predictive Tabular Tasks in Data Science
Authors:Yazheng Yang, Yuqi Wang, Sankalok Sen, Lei Li, Qi Liu
In the domain of data science, the predictive tasks of classification, regression, and imputation of missing values are commonly encountered challenges associated with tabular data. This research endeavors to apply Large Language Models (LLMs) towards addressing these predictive tasks. Despite their proficiency in comprehending natural language, LLMs fall short in dealing with structured tabular data. This limitation stems from their lacking exposure to the intricacies of tabular data during their foundational training. Our research aims to mitigate this gap by compiling a comprehensive corpus of tables annotated with instructions and executing large-scale training of Llama-2 on this enriched dataset. Furthermore, we investigate the practical application of applying the trained model to zero-shot prediction, few-shot prediction, and in-context learning scenarios. Through extensive experiments, our methodology has shown significant improvements over existing benchmarks. These advancements highlight the efficacy of tailoring LLM training to solve table-related problems in data science, thereby establishing a new benchmark in the utilization of LLMs for enhancing tabular intelligence.
PDF 10 pages
点此查看论文截图
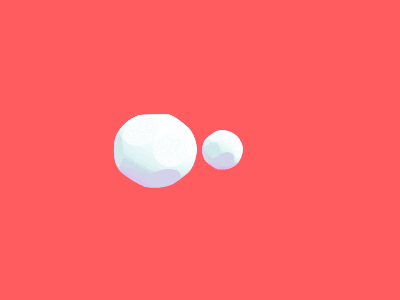
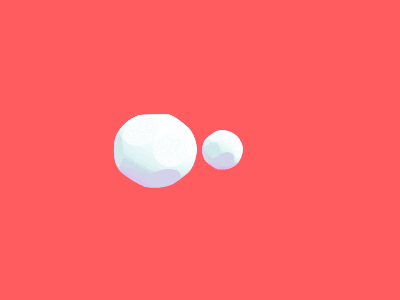
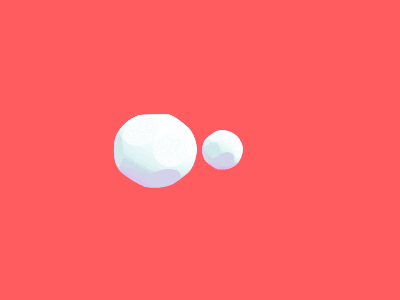
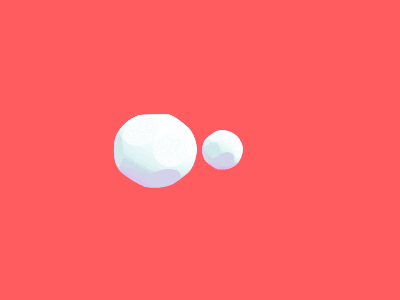
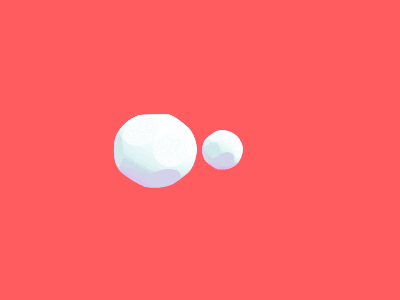
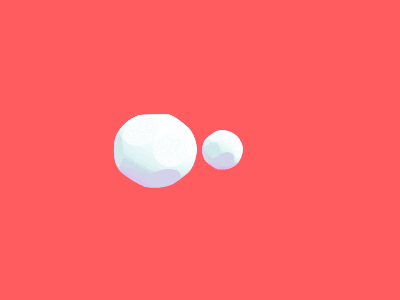