2024-01-04 更新
Video Understanding with Large Language Models: A Survey
Authors:Yunlong Tang, Jing Bi, Siting Xu, Luchuan Song, Susan Liang, Teng Wang, Daoan Zhang, Jie An, Jingyang Lin, Rongyi Zhu, Ali Vosoughi, Chao Huang, Zeliang Zhang, Feng Zheng, Jianguo Zhang, Ping Luo, Jiebo Luo, Chenliang Xu
With the burgeoning growth of online video platforms and the escalating volume of video content, the demand for proficient video understanding tools has intensified markedly. With Large Language Models (LLMs) showcasing remarkable capabilities in key language tasks, this survey provides a detailed overview of the recent advancements in video understanding harnessing the power of LLMs (Vid-LLMs). The emergent capabilities of Vid-LLMs are surprisingly advanced, particularly their ability for open-ended spatial-temporal reasoning combined with commonsense knowledge, suggesting a promising path for future video understanding. We examine the unique characteristics and capabilities of Vid-LLMs, categorizing the approaches into four main types: LLM-based Video Agents, Vid-LLMs Pretraining, Vid-LLMs Instruction Tuning, and Hybrid Methods. Furthermore, this survey also presents a comprehensive study of the tasks and datasets for Vid-LLMs, along with the methodologies employed for evaluation. Additionally, the survey explores the expansive applications of Vid-LLMs across various domains, thereby showcasing their remarkable scalability and versatility in addressing challenges in real-world video understanding. Finally, the survey summarizes the limitations of existing Vid-LLMs and the directions for future research. For more information, we recommend readers visit the repository at https://github.com/yunlong10/Awesome-LLMs-for-Video-Understanding.
PDF
点此查看论文截图
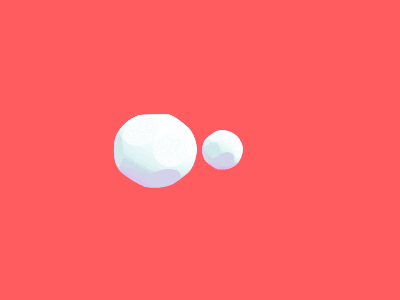
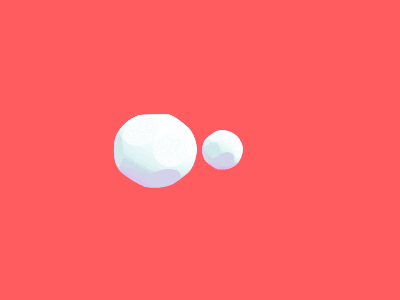
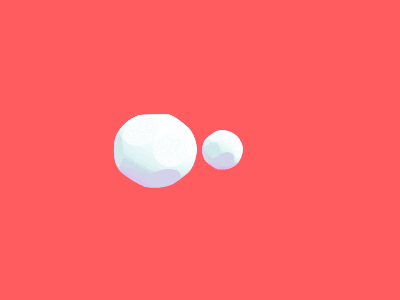
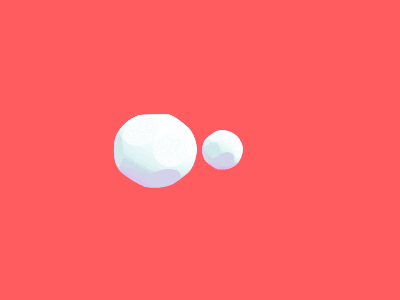