2023-12-26 更新
Revisiting Few-Shot Object Detection with Vision-Language Models
Authors:Anish Madan, Neehar Peri, Shu Kong, Deva Ramanan
Few-shot object detection (FSOD) benchmarks have advanced techniques for detecting new categories with limited annotations. Existing benchmarks repurpose well-established datasets like COCO by partitioning categories into base and novel classes for pre-training and fine-tuning respectively. However, these benchmarks do not reflect how FSOD is deployed in practice. Rather than only pre-training on a small number of base categories, we argue that it is more practical to fine-tune a foundation model (e.g., a vision-language model (VLM) pre-trained on web-scale data) for a target domain. Surprisingly, we find that zero-shot inference from VLMs like GroundingDINO significantly outperforms the state-of-the-art (48.3 vs. 33.1 AP) on COCO. However, such zero-shot models can still be misaligned to target concepts of interest. For example, trailers on the web may be different from trailers in the context of autonomous vehicles. In this work, we propose Foundational FSOD, a new benchmark protocol that evaluates detectors pre-trained on any external datasets and fine-tuned on K-shots per target class. Further, we note that current FSOD benchmarks are actually federated datasets containing exhaustive annotations for each category on a subset of the data. We leverage this insight to propose simple strategies for fine-tuning VLMs with federated losses. We demonstrate the effectiveness of our approach on LVIS and nuImages, improving over prior work by 5.9 AP.
PDF
点此查看论文截图
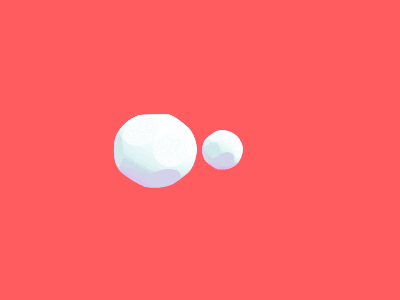
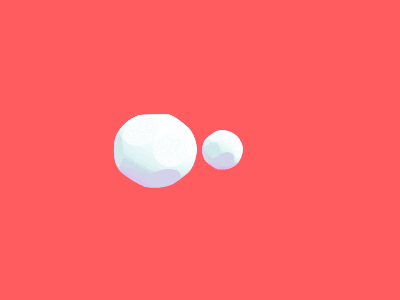
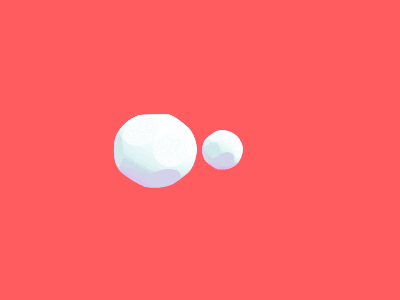
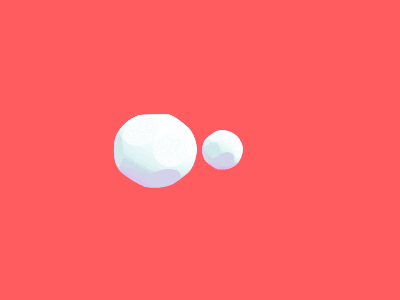
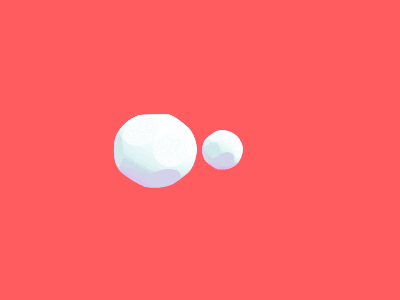
BSS-Bench: Towards Reproducible and Effective Band Selection Search
Authors:Wenshuai Xu, Zhenbo Xu
The key technology to overcome the drawbacks of hyperspectral imaging (expensive, high capture delay, and low spatial resolution) and make it widely applicable is to select only a few representative bands from hundreds of bands. However, current band selection (BS) methods face challenges in fair comparisons due to inconsistent train/validation settings, including the number of bands, dataset splits, and retraining settings. To make BS methods easy and reproducible, this paper presents the first band selection search benchmark (BSS-Bench) containing 52k training and evaluation records of numerous band combinations (BC) with different backbones for various hyperspectral analysis tasks. The creation of BSS-Bench required a significant computational effort of 1.26k GPU days. By querying BSS-Bench, BS experiments can be performed easily and reproducibly, and the gap between the searched result and the best achievable performance can be measured. Based on BSS-Bench, we further discuss the impact of various factors on BS, such as the number of bands, unsupervised statistics, and different backbones. In addition to BSS-Bench, we present an effective one-shot BS method called Single Combination One Shot (SCOS), which learns the priority of any BCs through one-time training, eliminating the need for repetitive retraining on different BCs. Furthermore, the search process of SCOS is flexible and does not require training, making it efficient and effective. Our extensive evaluations demonstrate that SCOS outperforms current BS methods on multiple tasks, even with much fewer bands. Our BSS-Bench and codes are available in the supplementary material and will be publicly available.
PDF 11 pages,6 figures
点此查看论文截图
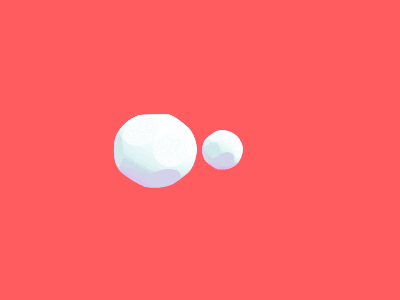
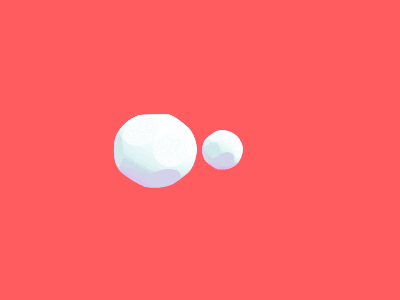
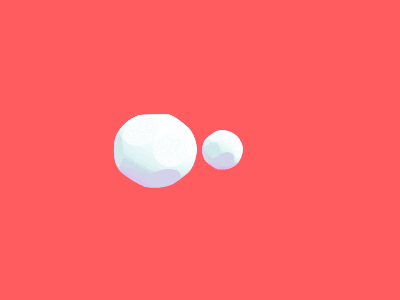
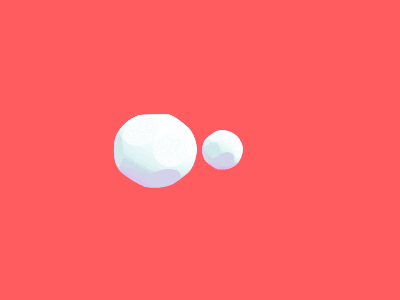
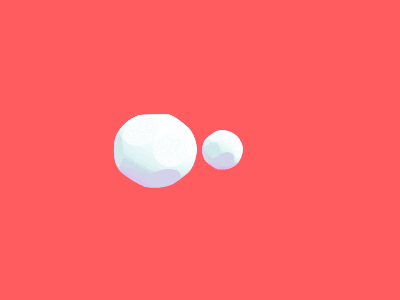