2023-12-07 更新
Optimizing rgb-d semantic segmentation through multi-modal interaction and pooling attention
Authors:Shuai Zhang, Minghong Xie
Semantic segmentation of RGB-D images involves understanding the appearance and spatial relationships of objects within a scene, which requires careful consideration of various factors. However, in indoor environments, the simple input of RGB and depth images often results in a relatively limited acquisition of semantic and spatial information, leading to suboptimal segmentation outcomes. To address this, we propose the Multi-modal Interaction and Pooling Attention Network (MIPANet), a novel approach designed to harness the interactive synergy between RGB and depth modalities, optimizing the utilization of complementary information. Specifically, we incorporate a Multi-modal Interaction Fusion Module (MIM) into the deepest layers of the network. This module is engineered to facilitate the fusion of RGB and depth information, allowing for mutual enhancement and correction. Additionally, we introduce a Pooling Attention Module (PAM) at various stages of the encoder. This module serves to amplify the features extracted by the network and integrates the module’s output into the decoder in a targeted manner, significantly improving semantic segmentation performance. Our experimental results demonstrate that MIPANet outperforms existing methods on two indoor scene datasets, NYUDv2 and SUN-RGBD, underscoring its effectiveness in enhancing RGB-D semantic segmentation.
PDF
点此查看论文截图
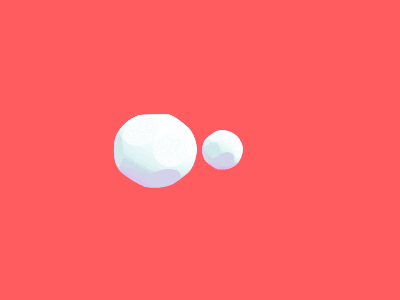
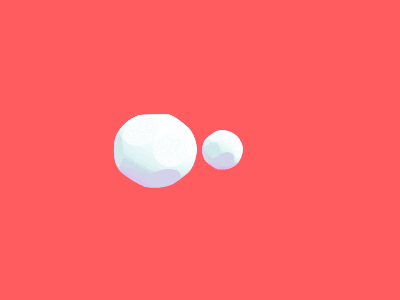
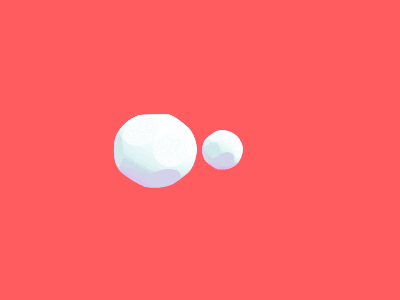
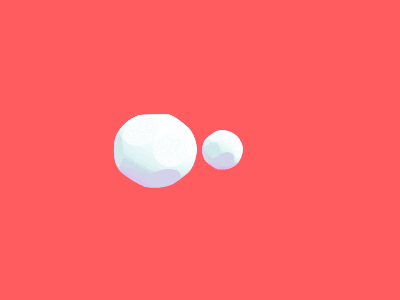
ShareCMP: Polarization-Aware RGB-P Semantic Segmentation
Authors:Zhuoyan Liu, Bo Wang, Lizhi Wang, Chenyu Mao, Ye Li
Multimodal semantic segmentation is developing rapidly, but the modality of RGB-Polarization remains underexplored. To delve into this problem, we construct a UPLight RGB-P segmentation benchmark with 12 typical underwater semantic classes which provides data support for Autonomous Underwater Vehicles (AUVs) to perform special perception tasks. In this work, we design the ShareCMP, an RGB-P semantic segmentation framework with a shared dual-branch architecture, which reduces the number of parameters by about 26-33% compared to previous dual-branch models. It encompasses a Polarization Generate Attention (PGA) module designed to generate polarization modal images with richer polarization properties for the encoder. In addition, we introduce the Class Polarization-Aware Loss (CPALoss) to improve the learning and understanding of the encoder for polarization modal information and to optimize the PGA module. With extensive experiments on a total of three RGB-P benchmarks, our ShareCMP achieves state-of-the-art performance in mIoU with fewer parameters on the UPLight (92.45%), ZJU (92.7%), and MCubeS (50.99%) datasets. The code is available at https://github.com/LEFTeyex/ShareCMP.
PDF 10 pages, 5 figures
点此查看论文截图
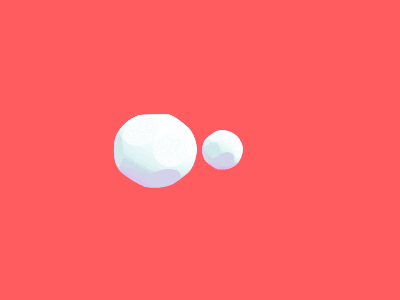
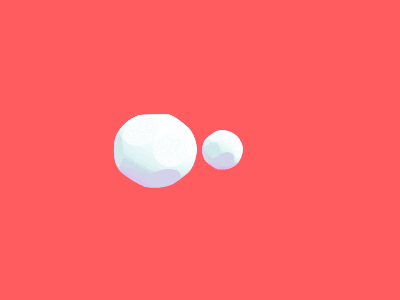
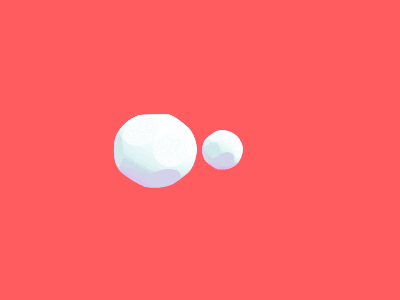
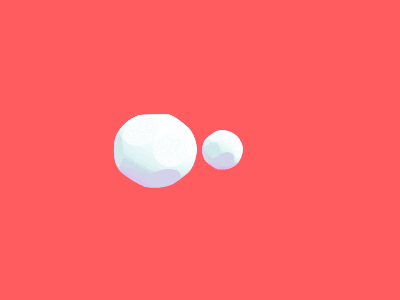
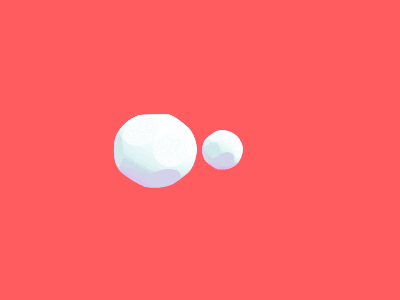
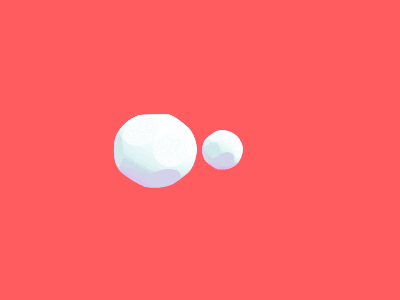
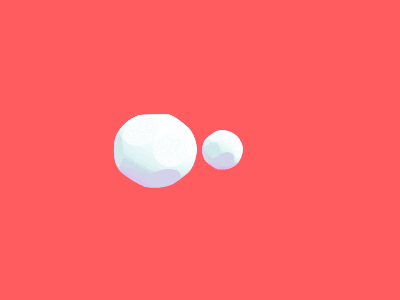
Texture-Semantic Collaboration Network for ORSI Salient Object Detection
Authors:Gongyang Li, Zhen Bai, Zhi Liu
Salient object detection (SOD) in optical remote sensing images (ORSIs) has become increasingly popular recently. Due to the characteristics of ORSIs, ORSI-SOD is full of challenges, such as multiple objects, small objects, low illuminations, and irregular shapes. To address these challenges, we propose a concise yet effective Texture-Semantic Collaboration Network (TSCNet) to explore the collaboration of texture cues and semantic cues for ORSI-SOD. Specifically, TSCNet is based on the generic encoder-decoder structure. In addition to the encoder and decoder, TSCNet includes a vital Texture-Semantic Collaboration Module (TSCM), which performs valuable feature modulation and interaction on basic features extracted from the encoder. The main idea of our TSCM is to make full use of the texture features at the lowest level and the semantic features at the highest level to achieve the expression enhancement of salient regions on features. In the TSCM, we first enhance the position of potential salient regions using semantic features. Then, we render and restore the object details using the texture features. Meanwhile, we also perceive regions of various scales, and construct interactions between different regions. Thanks to the perfect combination of TSCM and generic structure, our TSCNet can take care of both the position and details of salient objects, effectively handling various scenes. Extensive experiments on three datasets demonstrate that our TSCNet achieves competitive performance compared to 14 state-of-the-art methods. The code and results of our method are available at https://github.com/MathLee/TSCNet.
PDF 5 pages, 3 figures, Accepted by IEEE Transactions on Circuits and Systems II: Express Briefs 2023
点此查看论文截图
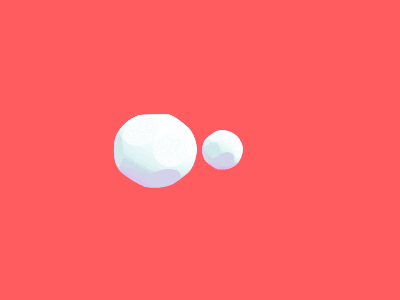
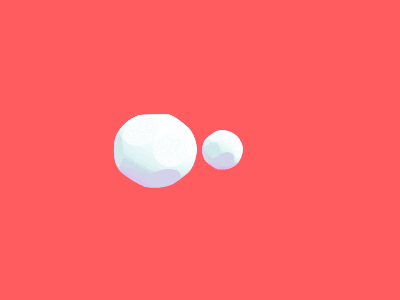
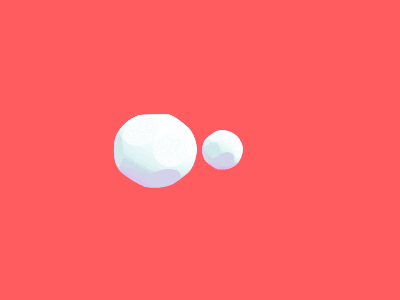
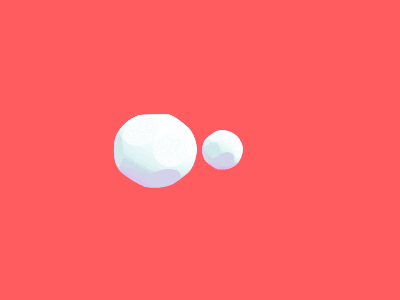
Foundation Model Assisted Weakly Supervised Semantic Segmentation
Authors:Xiaobo Yang, Xiaojin Gong
This work aims to leverage pre-trained foundation models, such as contrastive language-image pre-training (CLIP) and segment anything model (SAM), to address weakly supervised semantic segmentation (WSSS) using image-level labels. To this end, we propose a coarse-to-fine framework based on CLIP and SAM for generating high-quality segmentation seeds. Specifically, we construct an image classification task and a seed segmentation task, which are jointly performed by CLIP with frozen weights and two sets of learnable task-specific prompts. A SAM-based seeding (SAMS) module is designed and applied to each task to produce either coarse or fine seed maps. Moreover, we design a multi-label contrastive loss supervised by image-level labels and a CAM activation loss supervised by the generated coarse seed map. These losses are used to learn the prompts, which are the only parts need to be learned in our framework. Once the prompts are learned, we input each image along with the learned segmentation-specific prompts into CLIP and the SAMS module to produce high-quality segmentation seeds. These seeds serve as pseudo labels to train an off-the-shelf segmentation network like other two-stage WSSS methods. Experiments show that our method achieves the state-of-the-art performance on PASCAL VOC 2012 and competitive results on MS COCO 2014.
PDF
点此查看论文截图
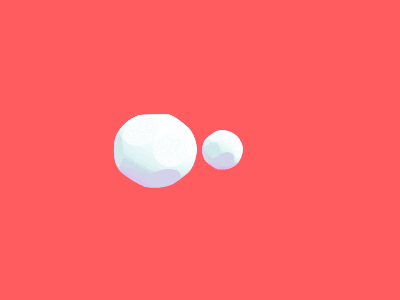
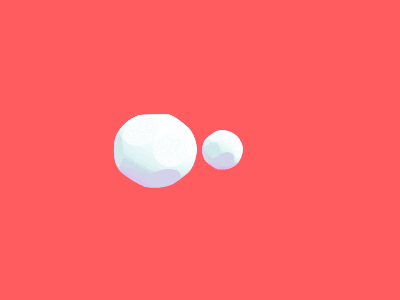
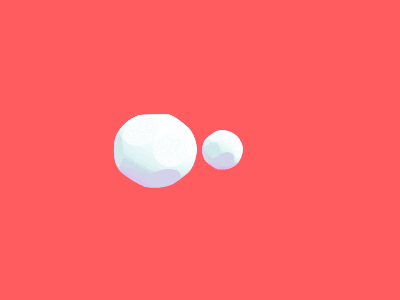
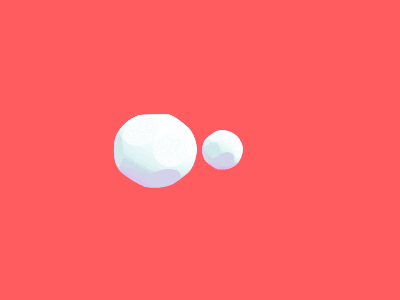