2023-12-01 更新
Editing 3D Scenes via Text Prompts without Retraining
Authors:Shuangkang Fang, Yufeng Wang, Yi Yang, Yi-Hsuan Tsai, Wenrui Ding, Shuchang Zhou, Ming-Hsuan Yang
Numerous diffusion models have recently been applied to image synthesis and editing. However, editing 3D scenes is still in its early stages. It poses various challenges, such as the requirement to design specific methods for different editing types, retraining new models for various 3D scenes, and the absence of convenient human interaction during editing. To tackle these issues, we introduce a text-driven editing method, termed DN2N, which allows for the direct acquisition of a NeRF model with universal editing capabilities, eliminating the requirement for retraining. Our method employs off-the-shelf text-based editing models of 2D images to modify the 3D scene images, followed by a filtering process to discard poorly edited images that disrupt 3D consistency. We then consider the remaining inconsistency as a problem of removing noise perturbation, which can be solved by generating training data with similar perturbation characteristics for training. We further propose cross-view regularization terms to help the generalized NeRF model mitigate these perturbations. Our text-driven method allows users to edit a 3D scene with their desired description, which is more friendly, intuitive, and practical than prior works. Empirical results show that our method achieves multiple editing types, including but not limited to appearance editing, weather transition, material changing, and style transfer. Most importantly, our method generalizes well with editing abilities shared among a set of model parameters without requiring a customized editing model for some specific scenes, thus inferring novel views with editing effects directly from user input. The project website is available at https://sk-fun.fun/DN2N
PDF Project Website: https://sk-fun.fun/DN2N
点此查看论文截图
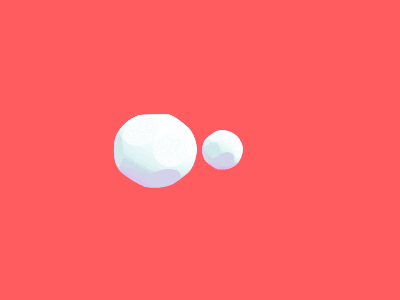
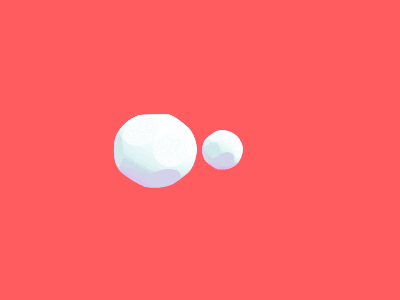
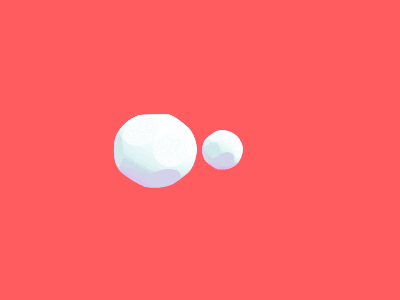
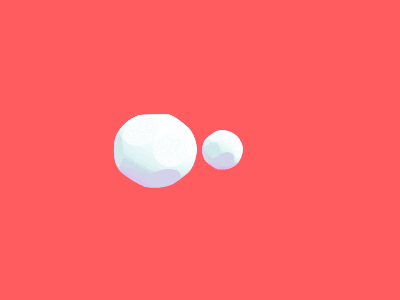
PEAN: A Diffusion-based Prior-Enhanced Attention Network for Scene Text Image Super-Resolution
Authors:Zuoyan Zhao, Shipeng Zhu, Pengfei Fang, Hui Xue
Scene text image super-resolution (STISR) aims at simultaneously increasing the resolution and readability of low-resolution scene text images, thus boosting the performance of the downstream recognition task. Two factors in scene text images, semantic information and visual structure, affect the recognition performance significantly. To mitigate the effects from these factors, this paper proposes a Prior-Enhanced Attention Network (PEAN). Specifically, a diffusion-based module is developed to enhance the text prior, hence offering better guidance for the SR network to generate SR images with higher semantic accuracy. Meanwhile, the proposed PEAN leverages an attention-based modulation module to understand scene text images by neatly perceiving the local and global dependence of images, despite the shape of the text. A multi-task learning paradigm is employed to optimize the network, enabling the model to generate legible SR images. As a result, PEAN establishes new SOTA results on the TextZoom benchmark. Experiments are also conducted to analyze the importance of the enhanced text prior as a means of improving the performance of the SR network. Code will be made available at https://github.com/jdfxzzy/PEAN.
PDF
点此查看论文截图
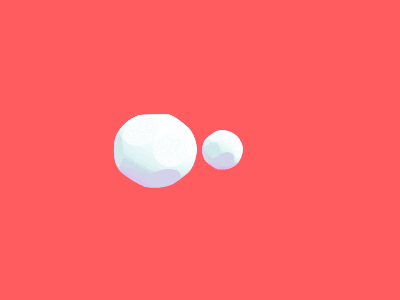
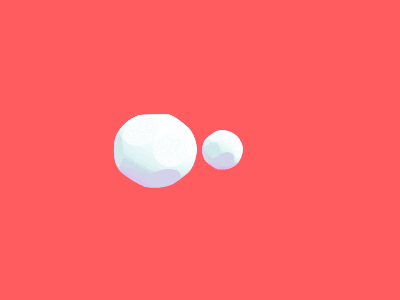
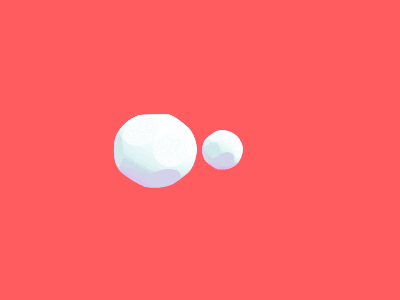
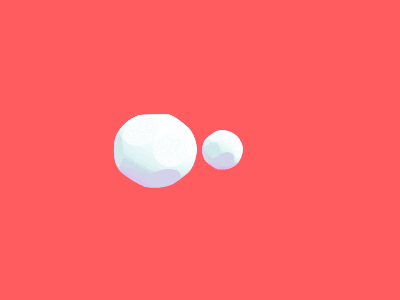
Detailed Human-Centric Text Description-Driven Large Scene Synthesis
Authors:Gwanghyun Kim, Dong Un Kang, Hoigi Seo, Hayeon Kim, Se Young Chun
Text-driven large scene image synthesis has made significant progress with diffusion models, but controlling it is challenging. While using additional spatial controls with corresponding texts has improved the controllability of large scene synthesis, it is still challenging to faithfully reflect detailed text descriptions without user-provided controls. Here, we propose DetText2Scene, a novel text-driven large-scale image synthesis with high faithfulness, controllability, and naturalness in a global context for the detailed human-centric text description. Our DetText2Scene consists of 1) hierarchical keypoint-box layout generation from the detailed description by leveraging large language model (LLM), 2) view-wise conditioned joint diffusion process to synthesize a large scene from the given detailed text with LLM-generated grounded keypoint-box layout and 3) pixel perturbation-based pyramidal interpolation to progressively refine the large scene for global coherence. Our DetText2Scene significantly outperforms prior arts in text-to-large scene synthesis qualitatively and quantitatively, demonstrating strong faithfulness with detailed descriptions, superior controllability, and excellent naturalness in a global context.
PDF
点此查看论文截图
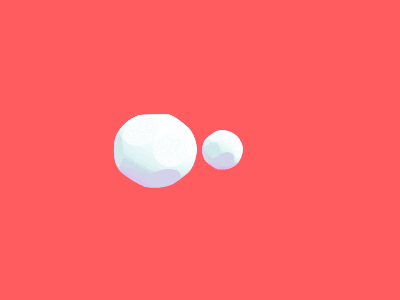
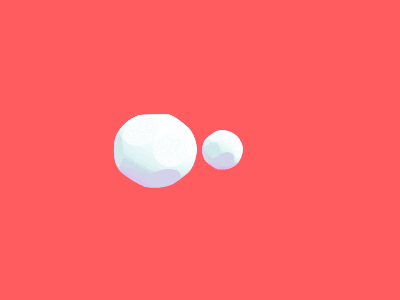
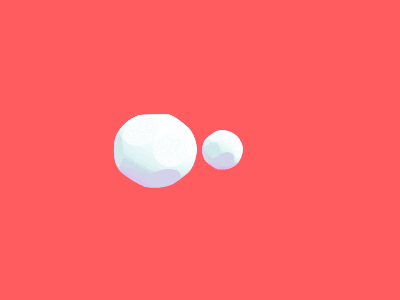
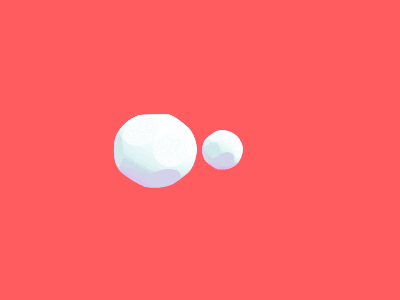
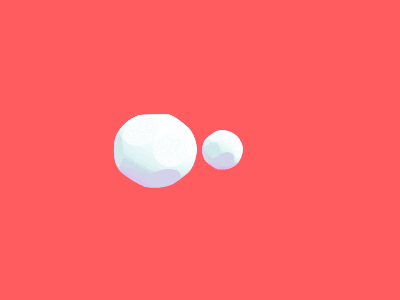
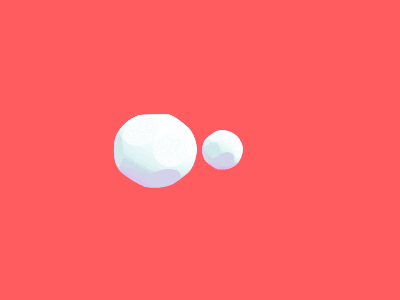