2023-11-25 更新
Semantic Face Compression for Metaverse: A Compact 3D Descriptor Based Approach
Authors:Binzhe Li, Bolin Chen, Zhao Wang, Shiqi Wang, Yan Ye
In this letter, we envision a new metaverse communication paradigm for virtual avatar faces, and develop the semantic face compression with compact 3D facial descriptors. The fundamental principle is that the communication of virtual avatar faces primarily emphasizes the conveyance of semantic information. In light of this, the proposed scheme offers the advantages of being highly flexible, efficient and semantically meaningful. The semantic face compression, which allows the communication of the descriptors for artificial intelligence based understanding, could facilitate numerous applications without the involvement of humans in metaverse. The promise of the proposed paradigm is also demonstrated by performance comparisons with the state-of-the-art video coding standard, Versatile Video Coding. A significant improvement in terms of rate-accuracy performance has been achieved. The proposed scheme is expected to enable numerous applications, such as digital human communication based on machine analysis, and to form the cornerstone of interaction and communication in the metaverse.
PDF 5 pages, 3 figures
点此查看论文截图
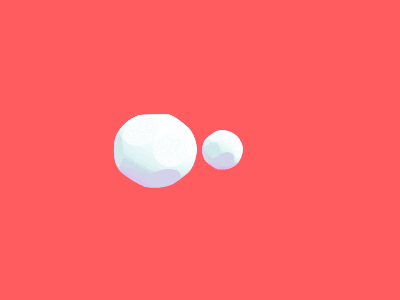
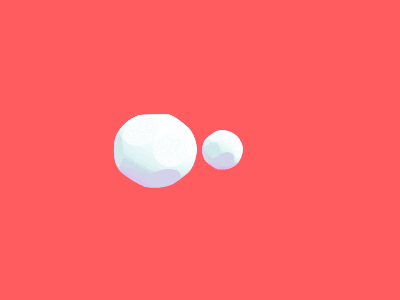
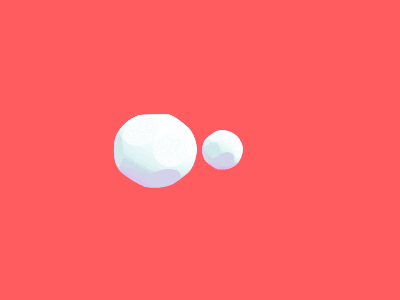
XAGen: 3D Expressive Human Avatars Generation
Authors:Zhongcong Xu, Jianfeng Zhang, Jun Hao Liew, Jiashi Feng, Mike Zheng Shou
Recent advances in 3D-aware GAN models have enabled the generation of realistic and controllable human body images. However, existing methods focus on the control of major body joints, neglecting the manipulation of expressive attributes, such as facial expressions, jaw poses, hand poses, and so on. In this work, we present XAGen, the first 3D generative model for human avatars capable of expressive control over body, face, and hands. To enhance the fidelity of small-scale regions like face and hands, we devise a multi-scale and multi-part 3D representation that models fine details. Based on this representation, we propose a multi-part rendering technique that disentangles the synthesis of body, face, and hands to ease model training and enhance geometric quality. Furthermore, we design multi-part discriminators that evaluate the quality of the generated avatars with respect to their appearance and fine-grained control capabilities. Experiments show that XAGen surpasses state-of-the-art methods in terms of realism, diversity, and expressive control abilities. Code and data will be made available at https://showlab.github.io/xagen.
PDF Accepted to NeurIPS 2023, Project Page at https://showlab.github.io/xagen
点此查看论文截图
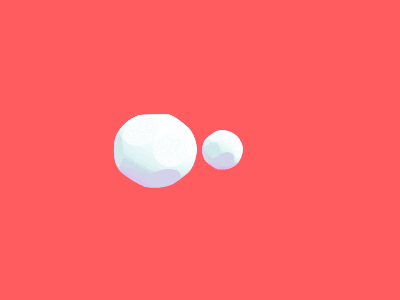
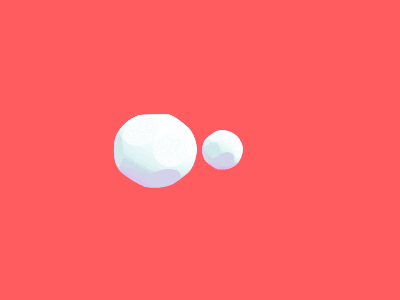
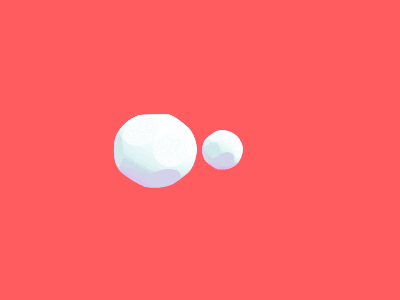