2023-11-14 更新
Monkey: Image Resolution and Text Label Are Important Things for Large Multi-modal Models
Authors:Zhang Li, Biao Yang, Qiang Liu, Zhiyin Ma, Shuo Zhang, Jingxu Yang, Yabo Sun, Yuliang Liu, Xiang Bai
Large Multimodal Models have demonstrated impressive capabilities in understanding general vision-language tasks. However, due to the limitation of supported input resolution (e.g., 448 x 448) as well as the inexhaustive description of the training image-text pair, these models often encounter challenges when dealing with intricate scene understandings and narratives. Here we address the problem by proposing the Monkey. Our contributions are two-fold: 1) without pretraining from the start, our method can be built upon an existing vision encoder (e.g., vit-BigHuge) to effectively improve the input resolution capacity up to 896 x 1344 pixels; 2) we propose a multi-level description generation method, which automatically provides rich information that can guide model to learn contextual association between scenes and objects. Our extensive testing across more than 16 distinct datasets reveals that Monkey achieves consistently competitive performance over the existing LMMs on fundamental tasks, such as Image Captioning, General Visual Question Answering (VQA), and Document-oriented VQA. Models, interactive demo, and the source code are provided at the following https://github.com/Yuliang-Liu/Monkey.
PDF
点此查看论文截图
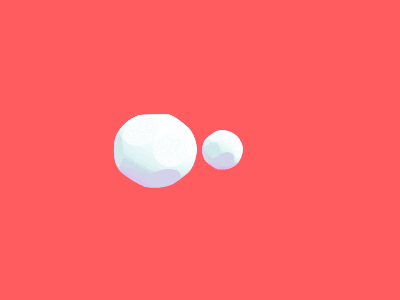
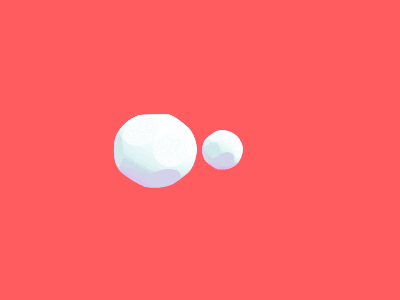
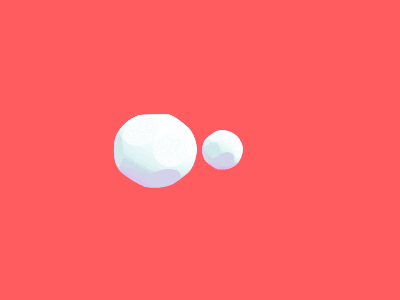
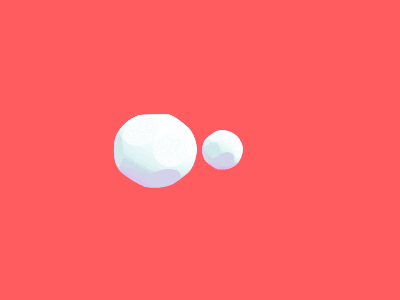
LT-ViT: A Vision Transformer for multi-label Chest X-ray classification
Authors:Umar Marikkar, Sara Atito, Muhammad Awais, Adam Mahdi
Vision Transformers (ViTs) are widely adopted in medical imaging tasks, and some existing efforts have been directed towards vision-language training for Chest X-rays (CXRs). However, we envision that there still exists a potential for improvement in vision-only training for CXRs using ViTs, by aggregating information from multiple scales, which has been proven beneficial for non-transformer networks. Hence, we have developed LT-ViT, a transformer that utilizes combined attention between image tokens and randomly initialized auxiliary tokens that represent labels. Our experiments demonstrate that LT-ViT (1) surpasses the state-of-the-art performance using pure ViTs on two publicly available CXR datasets, (2) is generalizable to other pre-training methods and therefore is agnostic to model initialization, and (3) enables model interpretability without grad-cam and its variants.
PDF 5 pages, 2 figures
点此查看论文截图
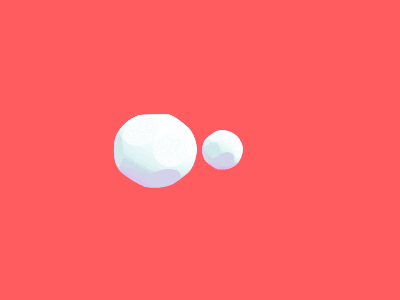
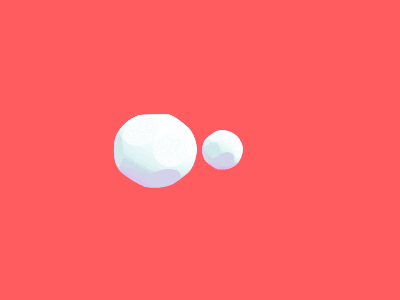
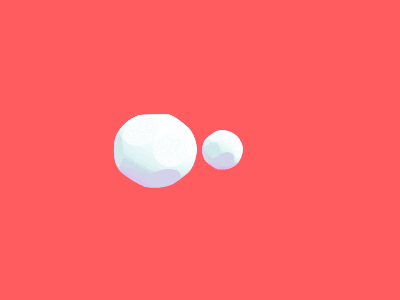
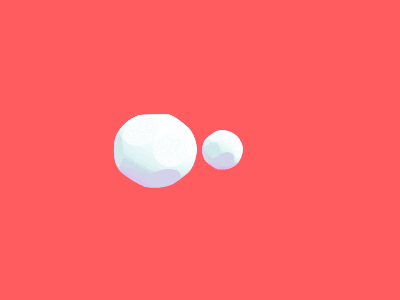