2023-10-05 更新
Confidence-based Visual Dispersal for Few-shot Unsupervised Domain Adaptation
Authors:Yizhe Xiong, Hui Chen, Zijia Lin, Sicheng Zhao, Guiguang Ding
Unsupervised domain adaptation aims to transfer knowledge from a fully-labeled source domain to an unlabeled target domain. However, in real-world scenarios, providing abundant labeled data even in the source domain can be infeasible due to the difficulty and high expense of annotation. To address this issue, recent works consider the Few-shot Unsupervised Domain Adaptation (FUDA) where only a few source samples are labeled, and conduct knowledge transfer via self-supervised learning methods. Yet existing methods generally overlook that the sparse label setting hinders learning reliable source knowledge for transfer. Additionally, the learning difficulty difference in target samples is different but ignored, leaving hard target samples poorly classified. To tackle both deficiencies, in this paper, we propose a novel Confidence-based Visual Dispersal Transfer learning method (C-VisDiT) for FUDA. Specifically, C-VisDiT consists of a cross-domain visual dispersal strategy that transfers only high-confidence source knowledge for model adaptation and an intra-domain visual dispersal strategy that guides the learning of hard target samples with easy ones. We conduct extensive experiments on Office-31, Office-Home, VisDA-C, and DomainNet benchmark datasets and the results demonstrate that the proposed C-VisDiT significantly outperforms state-of-the-art FUDA methods. Our code is available at https://github.com/Bostoncake/C-VisDiT.
PDF Accepted as ICCV 2023 poster (https://openaccess.thecvf.com/content/ICCV2023/html/Xiong_Confidence-based_Visual_Dispersal_for_Few-shot_Unsupervised_Domain_Adaptation_ICCV_2023_paper.html)
点此查看论文截图
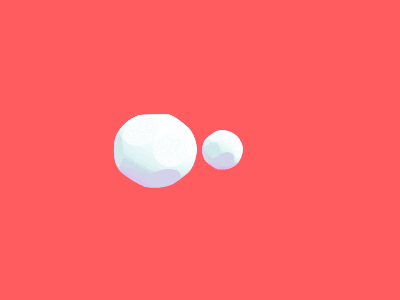
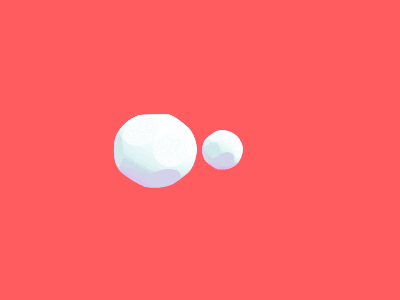
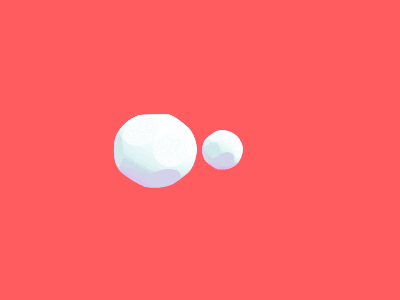
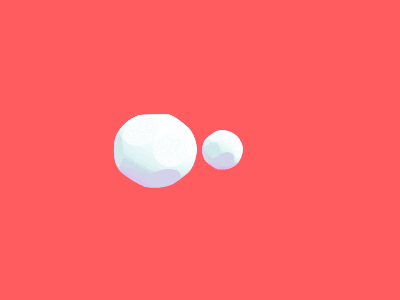
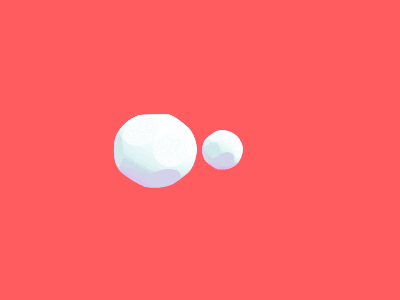
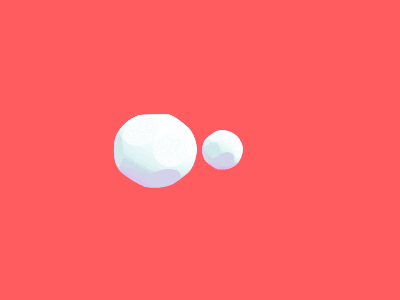
Open Compound Domain Adaptation with Object Style Compensation for Semantic Segmentation
Authors:Tingliang Feng, Hao Shi, Xueyang Liu, Wei Feng, Liang Wan, Yanlin Zhou, Di Lin
Many methods of semantic image segmentation have borrowed the success of open compound domain adaptation. They minimize the style gap between the images of source and target domains, more easily predicting the accurate pseudo annotations for target domain’s images that train segmentation network. The existing methods globally adapt the scene style of the images, whereas the object styles of different categories or instances are adapted improperly. This paper proposes the Object Style Compensation, where we construct the Object-Level Discrepancy Memory with multiple sets of discrepancy features. The discrepancy features in a set capture the style changes of the same category’s object instances adapted from target to source domains. We learn the discrepancy features from the images of source and target domains, storing the discrepancy features in memory. With this memory, we select appropriate discrepancy features for compensating the style information of the object instances of various categories, adapting the object styles to a unified style of source domain. Our method enables a more accurate computation of the pseudo annotations for target domain’s images, thus yielding state-of-the-art results on different datasets.
PDF Accepted by NeurlPS2023
点此查看论文截图
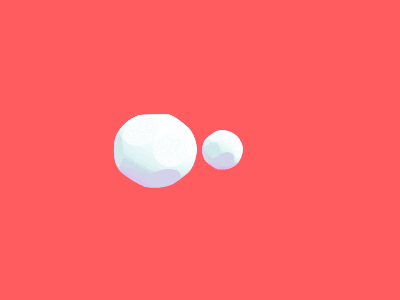
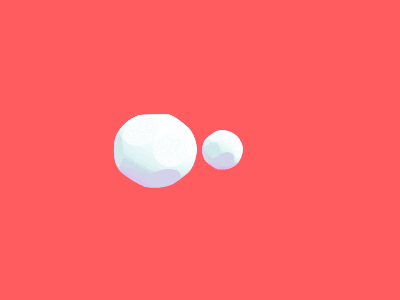
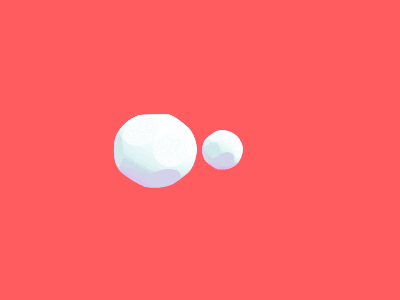
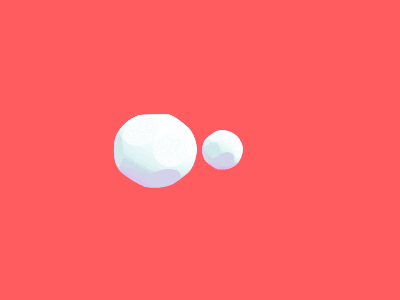
Cross-City Matters: A Multimodal Remote Sensing Benchmark Dataset for Cross-City Semantic Segmentation using High-Resolution Domain Adaptation Networks
Authors:Danfeng Hong, Bing Zhang, Hao Li, Yuxuan Li, Jing Yao, Chenyu Li, Martin Werner, Jocelyn Chanussot, Alexander Zipf, Xiao Xiang Zhu
Artificial intelligence (AI) approaches nowadays have gained remarkable success in single-modality-dominated remote sensing (RS) applications, especially with an emphasis on individual urban environments (e.g., single cities or regions). Yet these AI models tend to meet the performance bottleneck in the case studies across cities or regions, due to the lack of diverse RS information and cutting-edge solutions with high generalization ability. To this end, we build a new set of multimodal remote sensing benchmark datasets (including hyperspectral, multispectral, SAR) for the study purpose of the cross-city semantic segmentation task (called C2Seg dataset), which consists of two cross-city scenes, i.e., Berlin-Augsburg (in Germany) and Beijing-Wuhan (in China). Beyond the single city, we propose a high-resolution domain adaptation network, HighDAN for short, to promote the AI model’s generalization ability from the multi-city environments. HighDAN is capable of retaining the spatially topological structure of the studied urban scene well in a parallel high-to-low resolution fusion fashion but also closing the gap derived from enormous differences of RS image representations between different cities by means of adversarial learning. In addition, the Dice loss is considered in HighDAN to alleviate the class imbalance issue caused by factors across cities. Extensive experiments conducted on the C2Seg dataset show the superiority of our HighDAN in terms of segmentation performance and generalization ability, compared to state-of-the-art competitors. The C2Seg dataset and the semantic segmentation toolbox (involving the proposed HighDAN) will be available publicly at https://github.com/danfenghong.
PDF
点此查看论文截图
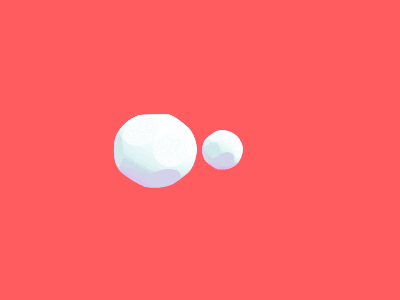
PC-Adapter: Topology-Aware Adapter for Efficient Domain Adaption on Point Clouds with Rectified Pseudo-label
Authors:Joonhyung Park, Hyunjin Seo, Eunho Yang
Understanding point clouds captured from the real-world is challenging due to shifts in data distribution caused by varying object scales, sensor angles, and self-occlusion. Prior works have addressed this issue by combining recent learning principles such as self-supervised learning, self-training, and adversarial training, which leads to significant computational overhead.Toward succinct yet powerful domain adaptation for point clouds, we revisit the unique challenges of point cloud data under domain shift scenarios and discover the importance of the global geometry of source data and trends of target pseudo-labels biased to the source label distribution. Motivated by our observations, we propose an adapter-guided domain adaptation method, PC-Adapter, that preserves the global shape information of the source domain using an attention-based adapter, while learning the local characteristics of the target domain via another adapter equipped with graph convolution. Additionally, we propose a novel pseudo-labeling strategy resilient to the classifier bias by adjusting confidence scores using their class-wise confidence distributions to consider relative confidences. Our method demonstrates superiority over baselines on various domain shift settings in benchmark datasets - PointDA, GraspNetPC, and PointSegDA.
PDF 11 pages; Accepted to ICCV 2023
点此查看论文截图
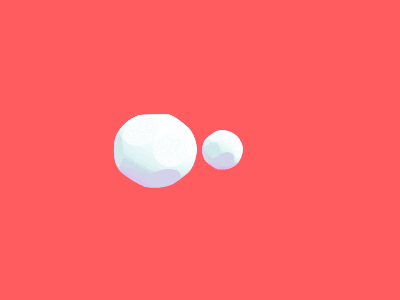
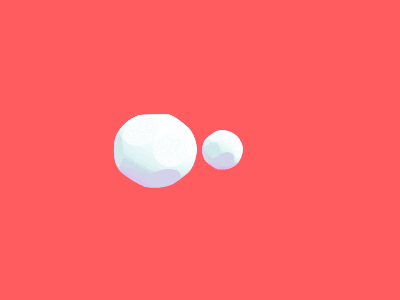
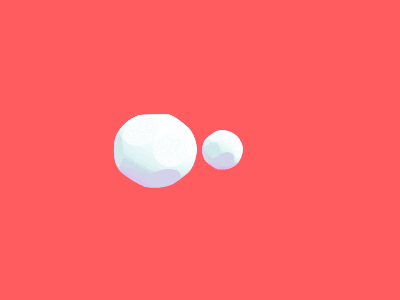
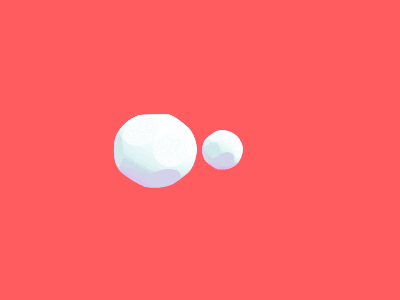
Domain-Adaptive Learning: Unsupervised Adaptation for Histology Images with Improved Loss Function Combination
Authors:Ravi Kant Gupta, Shounak Das, Amit Sethi
This paper presents a novel approach for unsupervised domain adaptation (UDA) targeting H&E stained histology images. Existing adversarial domain adaptation methods may not effectively align different domains of multimodal distributions associated with classification problems. The objective is to enhance domain alignment and reduce domain shifts between these domains by leveraging their unique characteristics. Our approach proposes a novel loss function along with carefully selected existing loss functions tailored to address the challenges specific to histology images. This loss combination not only makes the model accurate and robust but also faster in terms of training convergence. We specifically focus on leveraging histology-specific features, such as tissue structure and cell morphology, to enhance adaptation performance in the histology domain. The proposed method is extensively evaluated in accuracy, robustness, and generalization, surpassing state-of-the-art techniques for histology images. We conducted extensive experiments on the FHIST dataset and the results show that our proposed method - Domain Adaptive Learning (DAL) significantly surpasses the ViT-based and CNN-based SoTA methods by 1.41% and 6.56% respectively.
PDF
点此查看论文截图
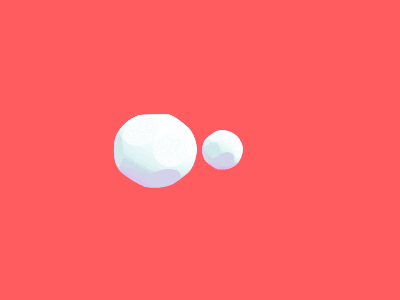
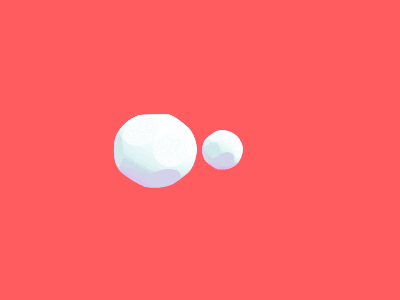
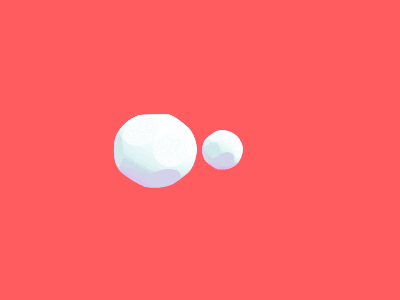
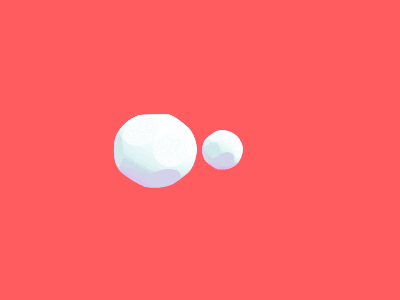
Few-Shot Domain Adaptation for Charge Prediction on Unprofessional Descriptions
Authors:Jie Zhao, Ziyu Guan, Wei Zhao, Yue Jiang, Xiaofei He
Recent works considering professional legal-linguistic style (PLLS) texts have shown promising results on the charge prediction task. However, unprofessional users also show an increasing demand on such a prediction service. There is a clear domain discrepancy between PLLS texts and non-PLLS texts expressed by those laypersons, which degrades the current SOTA models’ performance on non-PLLS texts. A key challenge is the scarcity of non-PLLS data for most charge classes. This paper proposes a novel few-shot domain adaptation (FSDA) method named Disentangled Legal Content for Charge Prediction (DLCCP). Compared with existing FSDA works, which solely perform instance-level alignment without considering the negative impact of text style information existing in latent features, DLCCP (1) disentangles the content and style representations for better domain-invariant legal content learning with carefully designed optimization goals for content and style spaces and, (2) employs the constitutive elements knowledge of charges to extract and align element-level and instance-level content representations simultaneously. We contribute the first publicly available non-PLLS dataset named NCCP for developing layperson-friendly charge prediction models. Experiments on NCCP show the superiority of our methods over competitive baselines.
PDF
点此查看论文截图
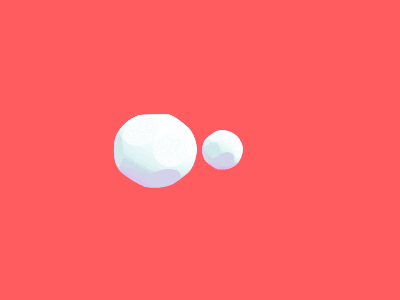
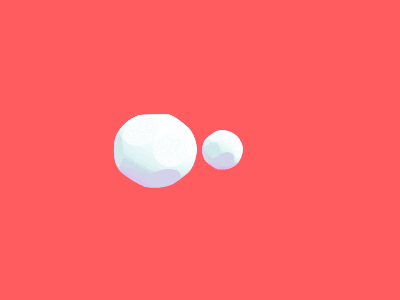
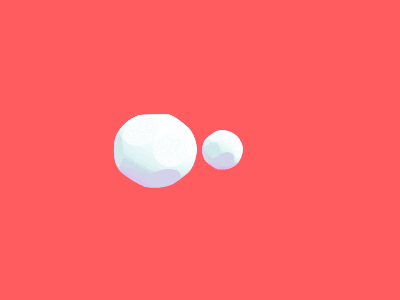
CLIPSelf: Vision Transformer Distills Itself for Open-Vocabulary Dense Prediction
Authors:Size Wu, Wenwei Zhang, Lumin Xu, Sheng Jin, Xiangtai Li, Wentao Liu, Chen Change Loy
Open-vocabulary dense prediction tasks including object detection and image segmentation have been advanced by the success of Contrastive Language-Image Pre-training (CLIP). CLIP models, particularly those incorporating vision transformers (ViTs), have exhibited remarkable generalization ability in zero-shot image classification. However, when transferring the vision-language alignment of CLIP from global image representation to local region representation for the open-vocabulary dense prediction tasks, CLIP ViTs suffer from the domain shift from full images to local image regions. In this paper, we embark on an in-depth analysis of the region-language alignment in CLIP models, which is essential for downstream open-vocabulary dense prediction tasks. Subsequently, we propose an approach named CLIPSelf, which adapts the image-level recognition ability of CLIP ViT to local image regions without needing any region-text pairs. CLIPSelf empowers ViTs to distill itself by aligning a region representation extracted from its dense feature map with the image-level representation of the corresponding image crop. With the enhanced CLIP ViTs, we achieve new state-of-the-art performance on open-vocabulary object detection, semantic segmentation, and panoptic segmentation across various benchmarks. Models and code will be available at https://github.com/wusize/CLIPSelf.
PDF
点此查看论文截图
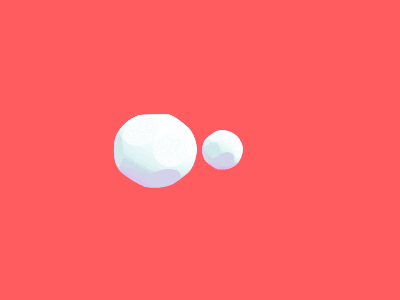
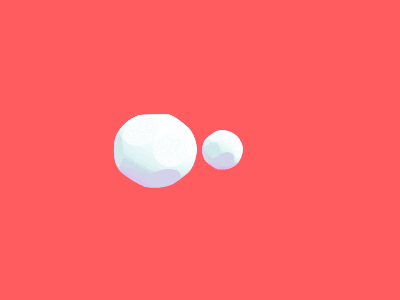
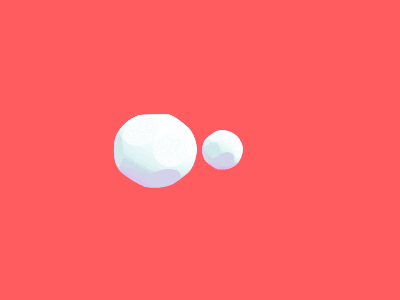
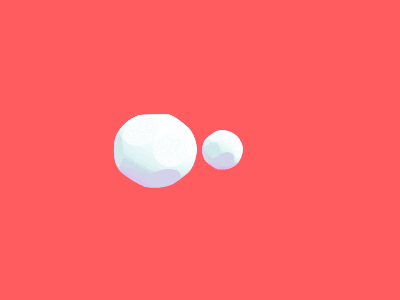
Empirical Study of PEFT techniques for Winter Wheat Segmentation
Authors:Mohamad Hasan Zahweh, Hasan Nasrallah, Mustafa Shukor, Ghaleb Faour, Ali J. Ghandour
Parameter Efficient Fine Tuning (PEFT) techniques have recently experienced significant growth and have been extensively employed to adapt large vision and language models to various domains, enabling satisfactory model performance with minimal computational needs. Despite these advances, more research has yet to delve into potential PEFT applications in real-life scenarios, particularly in the critical domains of remote sensing and crop monitoring. The diversity of climates across different regions and the need for comprehensive large-scale datasets have posed significant obstacles to accurately identify crop types across varying geographic locations and changing growing seasons. This study seeks to bridge this gap by comprehensively exploring the feasibility of cross-area and cross-year out-of-distribution generalization using the State-of-the-Art (SOTA) wheat crop monitoring model. The aim of this work is to explore PEFT approaches for crop monitoring. Specifically, we focus on adapting the SOTA TSViT model to address winter wheat field segmentation, a critical task for crop monitoring and food security. This adaptation process involves integrating different PEFT techniques, including BigFit, LoRA, Adaptformer, and prompt tuning. Using PEFT techniques, we achieved notable results comparable to those achieved using full fine-tuning methods while training only a mere 0.7% parameters of the whole TSViT architecture. The in-house labeled data-set, referred to as the Beqaa-Lebanon dataset, comprises high-quality annotated polygons for wheat and non-wheat classes with a total surface of 170 kmsq, over five consecutive years. Using Sentinel-2 images, our model achieved a 84% F1-score. We intend to publicly release the Lebanese winter wheat data set, code repository, and model weights.
PDF
点此查看论文截图
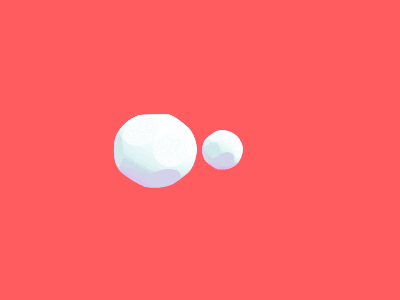
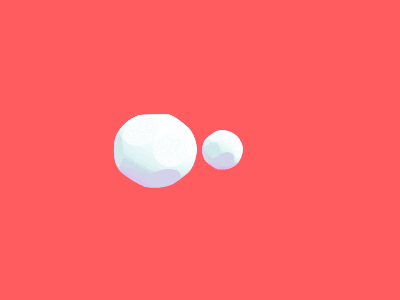
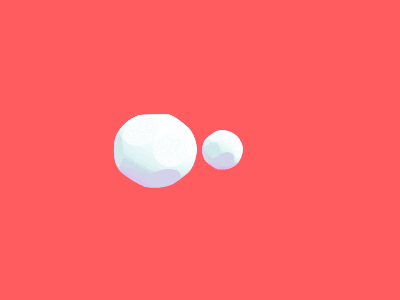
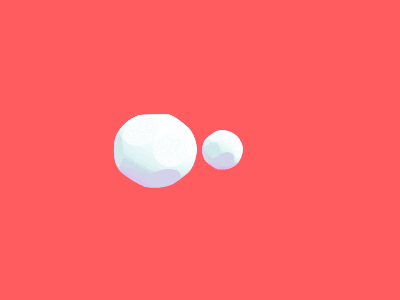
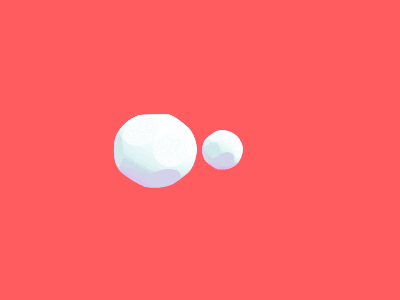
DARTH: Holistic Test-time Adaptation for Multiple Object Tracking
Authors:Mattia Segu, Bernt Schiele, Fisher Yu
Multiple object tracking (MOT) is a fundamental component of perception systems for autonomous driving, and its robustness to unseen conditions is a requirement to avoid life-critical failures. Despite the urge of safety in driving systems, no solution to the MOT adaptation problem to domain shift in test-time conditions has ever been proposed. However, the nature of a MOT system is manifold - requiring object detection and instance association - and adapting all its components is non-trivial. In this paper, we analyze the effect of domain shift on appearance-based trackers, and introduce DARTH, a holistic test-time adaptation framework for MOT. We propose a detection consistency formulation to adapt object detection in a self-supervised fashion, while adapting the instance appearance representations via our novel patch contrastive loss. We evaluate our method on a variety of domain shifts - including sim-to-real, outdoor-to-indoor, indoor-to-outdoor - and substantially improve the source model performance on all metrics. Code: https://github.com/mattiasegu/darth.
PDF Proceedings of the IEEE/CVF International Conference on Computer Vision
点此查看论文截图
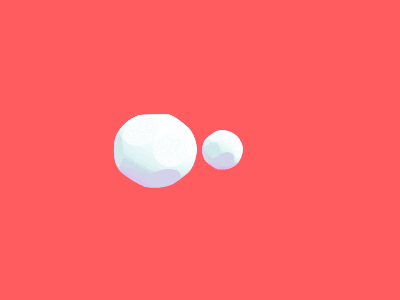
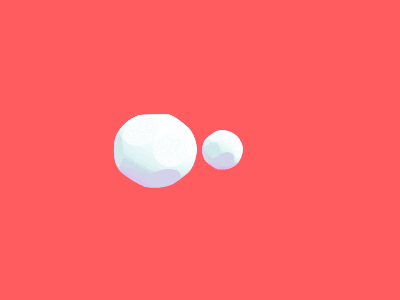
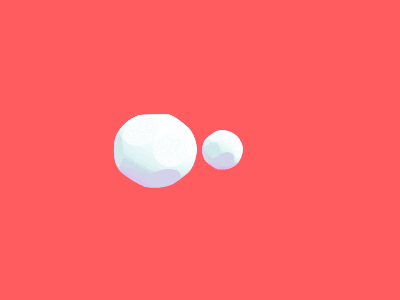
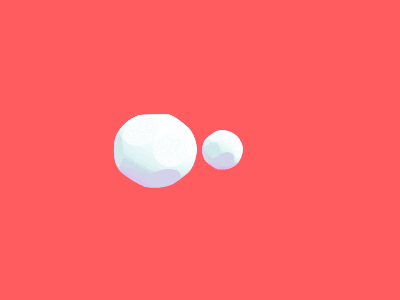
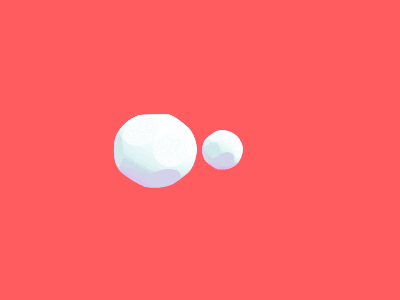
Learnable Data Augmentation for One-Shot Unsupervised Domain Adaptation
Authors:Julio Ivan Davila Carrazco, Pietro Morerio, Alessio Del Bue, Vittorio Murino
This paper presents a classification framework based on learnable data augmentation to tackle the One-Shot Unsupervised Domain Adaptation (OS-UDA) problem. OS-UDA is the most challenging setting in Domain Adaptation, as only one single unlabeled target sample is assumed to be available for model adaptation. Driven by such single sample, our method LearnAug-UDA learns how to augment source data, making it perceptually similar to the target. As a result, a classifier trained on such augmented data will generalize well for the target domain. To achieve this, we designed an encoder-decoder architecture that exploits a perceptual loss and style transfer strategies to augment the source data. Our method achieves state-of-the-art performance on two well-known Domain Adaptation benchmarks, DomainNet and VisDA. The project code is available at https://github.com/IIT-PAVIS/LearnAug-UDA
PDF Accepted to The 34th British Machine Vision Conference (BMVC 2023)
点此查看论文截图
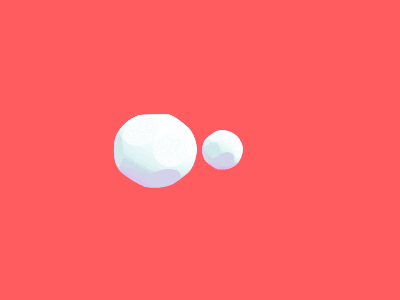
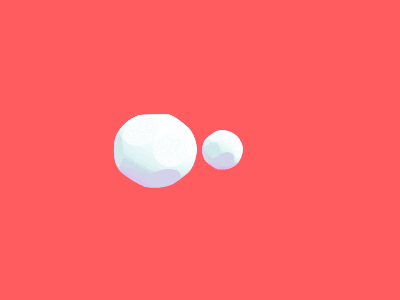
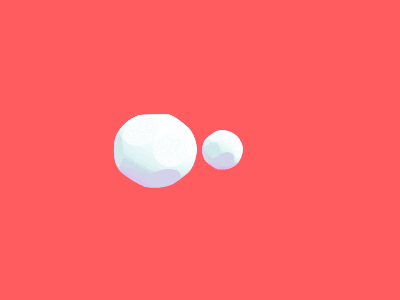
Prompting-based Efficient Temporal Domain Generalization
Authors:Sepidehsadat Hosseini, Mengyao Zhai, Hossein Hajimirsadegh, Frederick Tung
Machine learning traditionally assumes that training and testing data are distributed independently and identically. However, in many real-world settings, the data distribution can shift over time, leading to poor generalization of trained models in future time periods. Our paper presents a novel prompting-based approach to temporal domain generalization that is parameter-efficient, time-efficient, and does not require access to the target domain data (i.e., unseen future time periods) during training. Our method adapts a target pre-trained model to temporal drift by learning global prompts, domain-specific prompts, and drift-aware prompts that capture underlying temporal dynamics. It is compatible across diverse tasks, such as classification, regression, and time series forecasting, and sets a new state-of-the-art benchmark in temporal domain generalization. The code repository will be publicly shared.
PDF
点此查看论文截图
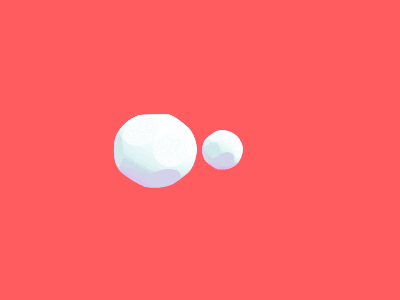
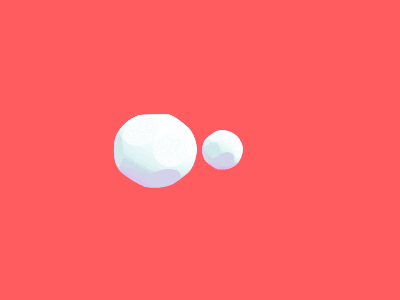
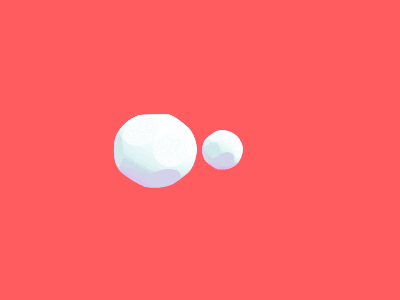
MedDiffusion: Boosting Health Risk Prediction via Diffusion-based Data Augmentation
Authors:Yuan Zhong, Suhan Cui, Jiaqi Wang, Xiaochen Wang, Ziyi Yin, Yaqing Wang, Houping Xiao, Mengdi Huai, Ting Wang, Fenglong Ma
Health risk prediction is one of the fundamental tasks under predictive modeling in the medical domain, which aims to forecast the potential health risks that patients may face in the future using their historical Electronic Health Records (EHR). Researchers have developed several risk prediction models to handle the unique challenges of EHR data, such as its sequential nature, high dimensionality, and inherent noise. These models have yielded impressive results. Nonetheless, a key issue undermining their effectiveness is data insufficiency. A variety of data generation and augmentation methods have been introduced to mitigate this issue by expanding the size of the training data set through the learning of underlying data distributions. However, the performance of these methods is often limited due to their task-unrelated design. To address these shortcomings, this paper introduces a novel, end-to-end diffusion-based risk prediction model, named MedDiffusion. It enhances risk prediction performance by creating synthetic patient data during training to enlarge sample space. Furthermore, MedDiffusion discerns hidden relationships between patient visits using a step-wise attention mechanism, enabling the model to automatically retain the most vital information for generating high-quality data. Experimental evaluation on four real-world medical datasets demonstrates that MedDiffusion outperforms 14 cutting-edge baselines in terms of PR-AUC, F1, and Cohen’s Kappa. We also conduct ablation studies and benchmark our model against GAN-based alternatives to further validate the rationality and adaptability of our model design. Additionally, we analyze generated data to offer fresh insights into the model’s interpretability.
PDF 9 main pages + 3 appendix pages , submitted to SDM 2024
点此查看论文截图
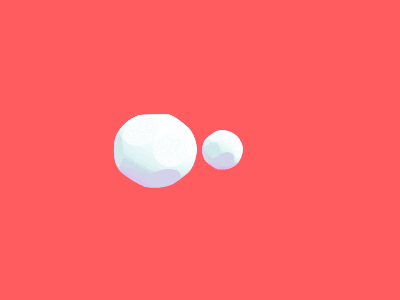
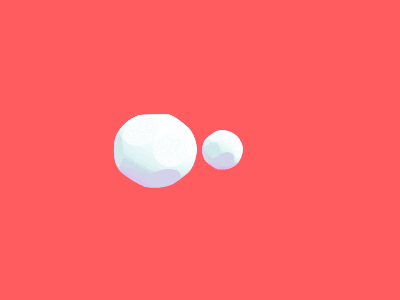
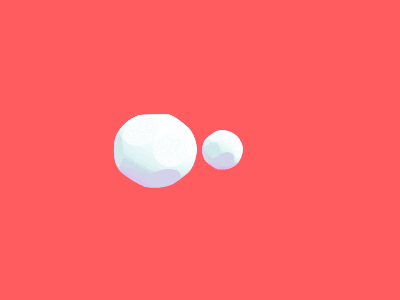
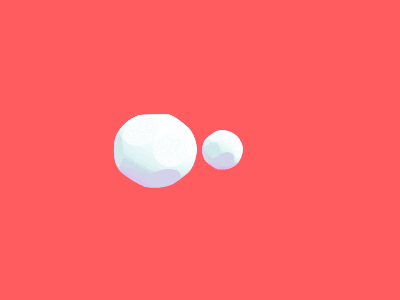
Low Resource Summarization using Pre-trained Language Models
Authors:Mubashir Munaf, Hammad Afzal, Naima Iltaf, Khawir Mahmood
With the advent of Deep Learning based Artificial Neural Networks models, Natural Language Processing (NLP) has witnessed significant improvements in textual data processing in terms of its efficiency and accuracy. However, the research is mostly restricted to high-resource languages such as English and low-resource languages still suffer from a lack of available resources in terms of training datasets as well as models with even baseline evaluation results. Considering the limited availability of resources for low-resource languages, we propose a methodology for adapting self-attentive transformer-based architecture models (mBERT, mT5) for low-resource summarization, supplemented by the construction of a new baseline dataset (76.5k article, summary pairs) in a low-resource language Urdu. Choosing news (a publicly available source) as the application domain has the potential to make the proposed methodology useful for reproducing in other languages with limited resources. Our adapted summarization model \textit{urT5} with up to 44.78\% reduction in size as compared to \textit{mT5} can capture contextual information of low resource language effectively with evaluation score (up to 46.35 ROUGE-1, 77 BERTScore) at par with state-of-the-art models in high resource language English \textit{(PEGASUS: 47.21, BART: 45.14 on XSUM Dataset)}. The proposed method provided a baseline approach towards extractive as well as abstractive summarization with competitive evaluation results in a limited resource setup.
PDF 17 pages, 7 figures, 3 tables
点此查看论文截图
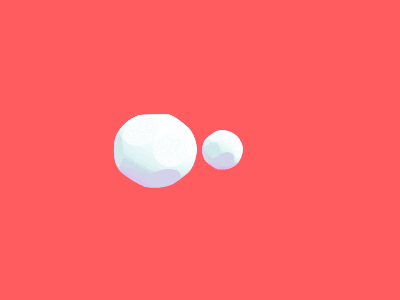
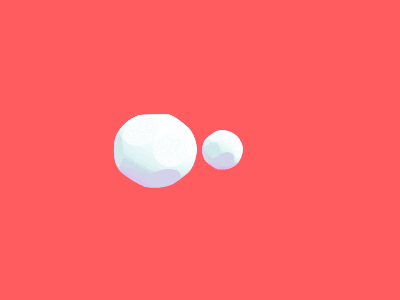
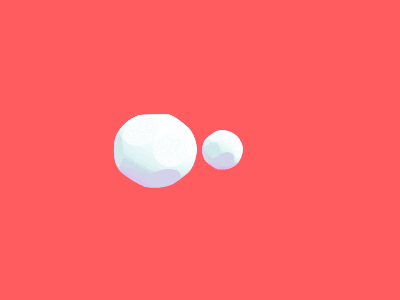
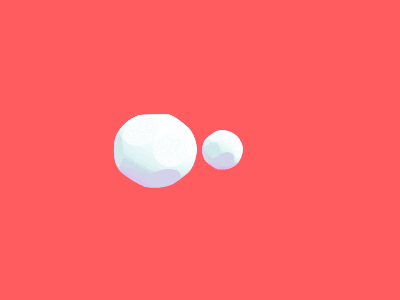
A novel asymmetrical autoencoder with a sparsifying discrete cosine Stockwell transform layer for gearbox sensor data compression
Authors:Xin Zhu, Daoguang Yang, Hongyi Pan, Hamid Reza Karimi, Didem Ozevin, Ahmet Enis Cetin
The lack of an efficient compression model remains a challenge for the wireless transmission of gearbox data in non-contact gear fault diagnosis problems. In this paper, we present a signal-adaptive asymmetrical autoencoder with a transform domain layer to compress sensor signals. First, a new discrete cosine Stockwell transform (DCST) layer is introduced to replace linear layers in a multi-layer autoencoder. A trainable filter is implemented in the DCST domain by utilizing the multiplication property of the convolution. A trainable hard-thresholding layer is applied to reduce redundant data in the DCST layer to make the feature map sparse. In comparison to the linear layer, the DCST layer reduces the number of trainable parameters and improves the accuracy of data reconstruction. Second, training the autoencoder with a sparsifying DCST layer only requires a small number of datasets. The proposed method is superior to other autoencoder-based methods on the University of Connecticut (UoC) and Southeast University (SEU) gearbox datasets, as the average quality score is improved by 2.00% at the lowest and 32.35% at the highest with a limited number of training samples
PDF
点此查看论文截图
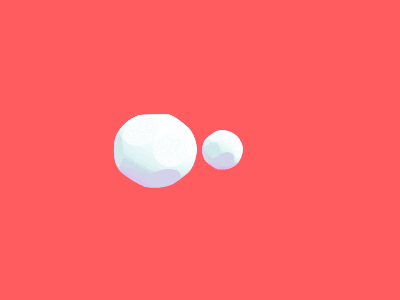
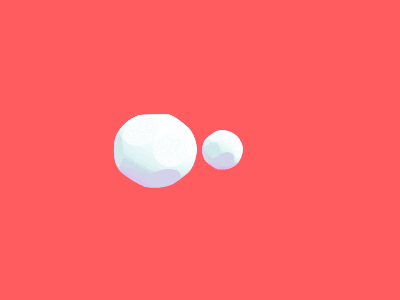