2023-07-15 更新
KIT’s Multilingual Speech Translation System for IWSLT 2023
Authors:Danni Liu, Thai Binh Nguyen, Sai Koneru, Enes Yavuz Ugan, Ngoc-Quan Pham, Tuan-Nam Nguyen, Tu Anh Dinh, Carlos Mullov, Alexander Waibel, Jan Niehues
Many existing speech translation benchmarks focus on native-English speech in high-quality recording conditions, which often do not match the conditions in real-life use-cases. In this paper, we describe our speech translation system for the multilingual track of IWSLT 2023, which evaluates translation quality on scientific conference talks. The test condition features accented input speech and terminology-dense contents. The task requires translation into 10 languages of varying amounts of resources. In absence of training data from the target domain, we use a retrieval-based approach (kNN-MT) for effective adaptation (+0.8 BLEU for speech translation). We also use adapters to easily integrate incremental training data from data augmentation, and show that it matches the performance of re-training. We observe that cascaded systems are more easily adaptable towards specific target domains, due to their separate modules. Our cascaded speech system substantially outperforms its end-to-end counterpart on scientific talk translation, although their performance remains similar on TED talks.
PDF IWSLT 2023
点此查看论文截图
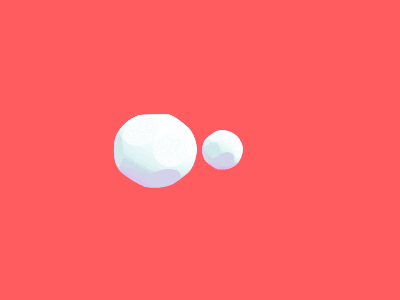
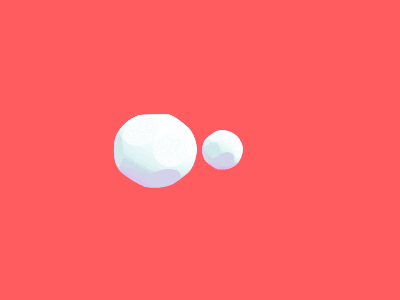
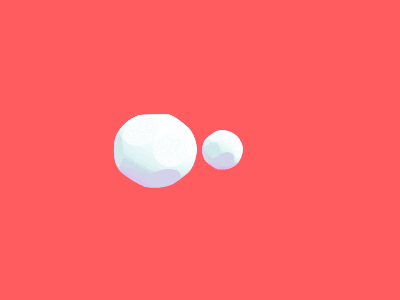
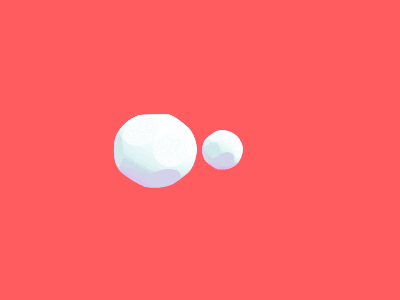
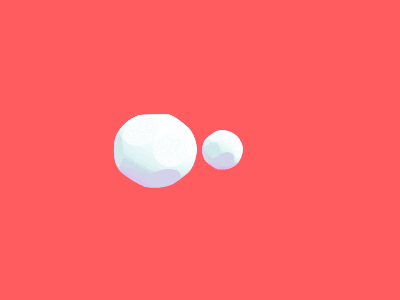
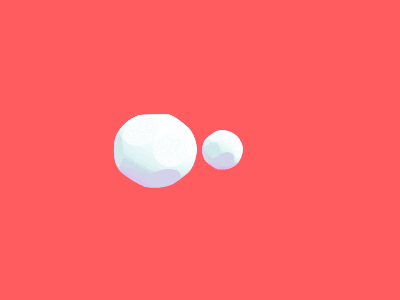
Token-Level Serialized Output Training for Joint Streaming ASR and ST Leveraging Textual Alignments
Authors:Sara Papi, Peidong Wan, Junkun Chen, Jian Xue, Jinyu Li, Yashesh Gaur
In real-world applications, users often require both translations and transcriptions of speech to enhance their comprehension, particularly in streaming scenarios where incremental generation is necessary. This paper introduces a streaming Transformer-Transducer that jointly generates automatic speech recognition (ASR) and speech translation (ST) outputs using a single decoder. To produce ASR and ST content effectively with minimal latency, we propose a joint token-level serialized output training method that interleaves source and target words by leveraging an off-the-shelf textual aligner. Experiments in monolingual (it-en) and multilingual ({de,es,it}-en) settings demonstrate that our approach achieves the best quality-latency balance. With an average ASR latency of 1s and ST latency of 1.3s, our model shows no degradation or even improves output quality compared to separate ASR and ST models, yielding an average improvement of 1.1 WER and 0.4 BLEU in the multilingual case.
PDF
点此查看论文截图
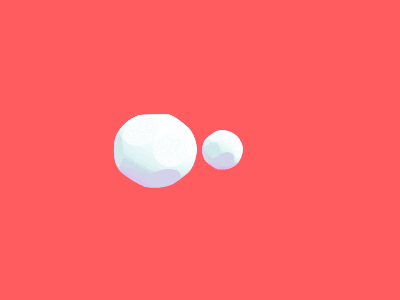
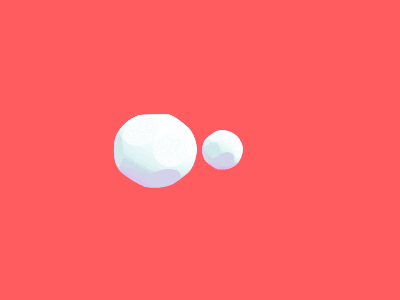
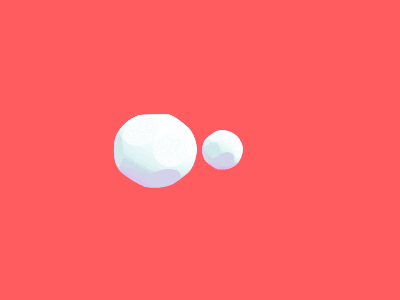
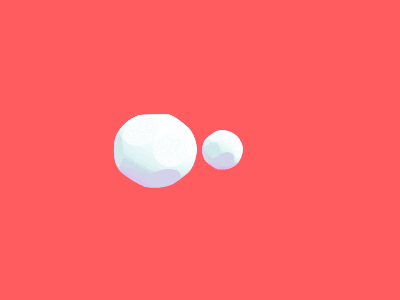
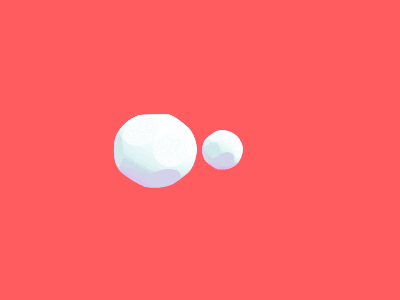
Can Generative Large Language Models Perform ASR Error Correction?
Authors:Rao Ma, Mengjie Qian, Potsawee Manakul, Mark Gales, Kate Knill
ASR error correction continues to serve as an important part of post-processing for speech recognition systems. Traditionally, these models are trained with supervised training using the decoding results of the underlying ASR system and the reference text. This approach is computationally intensive and the model needs to be re-trained when switching the underlying ASR model. Recent years have seen the development of large language models and their ability to perform natural language processing tasks in a zero-shot manner. In this paper, we take ChatGPT as an example to examine its ability to perform ASR error correction in the zero-shot or 1-shot settings. We use the ASR N-best list as model input and propose unconstrained error correction and N-best constrained error correction methods. Results on a Conformer-Transducer model and the pre-trained Whisper model show that we can largely improve the ASR system performance with error correction using the powerful ChatGPT model.
PDF
点此查看论文截图
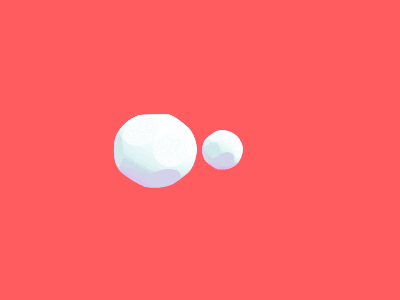
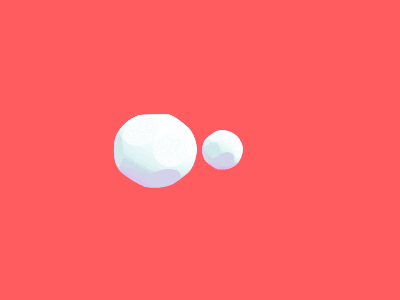
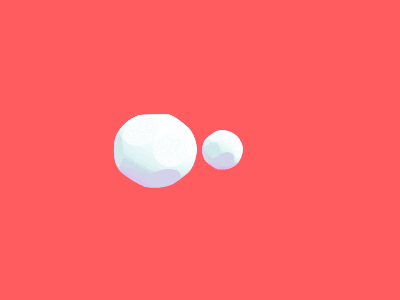
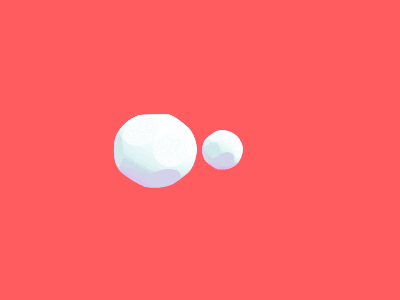
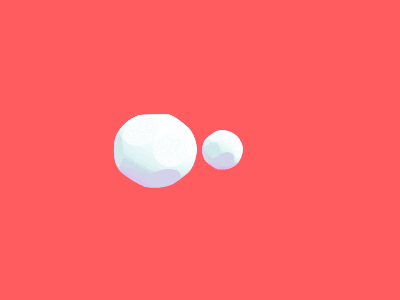
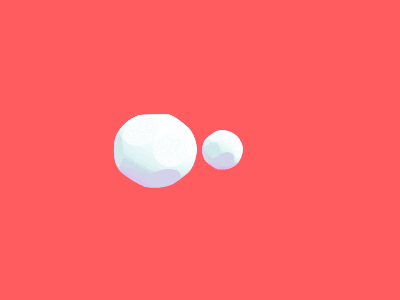
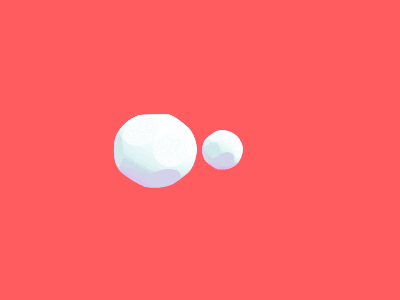
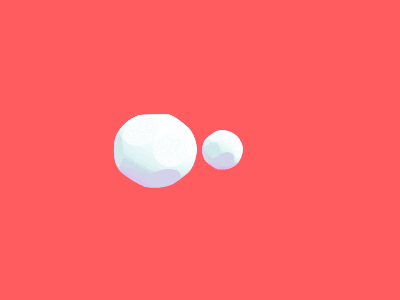
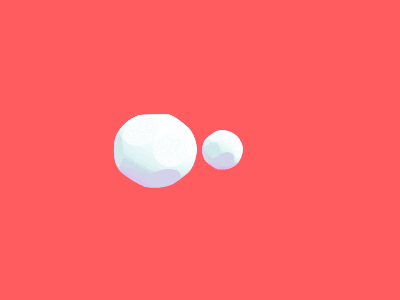
SparseVSR: Lightweight and Noise Robust Visual Speech Recognition
Authors:Adriana Fernandez-Lopez, Honglie Chen, Pingchuan Ma, Alexandros Haliassos, Stavros Petridis, Maja Pantic
Recent advances in deep neural networks have achieved unprecedented success in visual speech recognition. However, there remains substantial disparity between current methods and their deployment in resource-constrained devices. In this work, we explore different magnitude-based pruning techniques to generate a lightweight model that achieves higher performance than its dense model equivalent, especially under the presence of visual noise. Our sparse models achieve state-of-the-art results at 10% sparsity on the LRS3 dataset and outperform the dense equivalent up to 70% sparsity. We evaluate our 50% sparse model on 7 different visual noise types and achieve an overall absolute improvement of more than 2% WER compared to the dense equivalent. Our results confirm that sparse networks are more resistant to noise than dense networks.
PDF Accepted to Interspeech 2023
点此查看论文截图
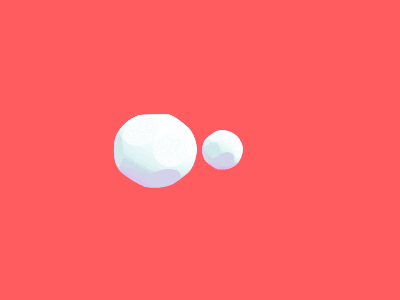
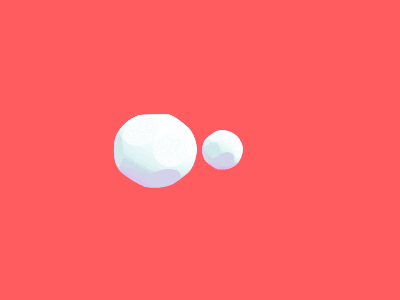
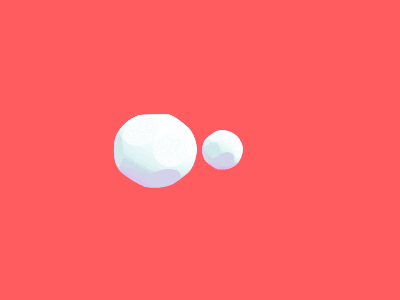
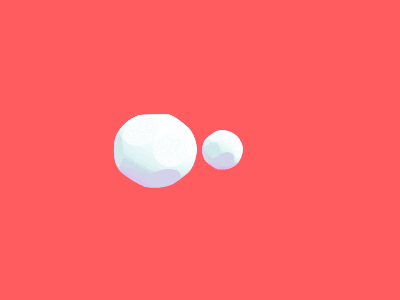
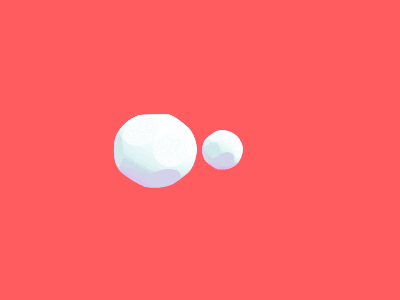
Personalization for BERT-based Discriminative Speech Recognition Rescoring
Authors:Jari Kolehmainen, Yile Gu, Aditya Gourav, Prashanth Gurunath Shivakumar, Ankur Gandhe, Ariya Rastrow, Ivan Bulyko
Recognition of personalized content remains a challenge in end-to-end speech recognition. We explore three novel approaches that use personalized content in a neural rescoring step to improve recognition: gazetteers, prompting, and a cross-attention based encoder-decoder model. We use internal de-identified en-US data from interactions with a virtual voice assistant supplemented with personalized named entities to compare these approaches. On a test set with personalized named entities, we show that each of these approaches improves word error rate by over 10%, against a neural rescoring baseline. We also show that on this test set, natural language prompts can improve word error rate by 7% without any training and with a marginal loss in generalization. Overall, gazetteers were found to perform the best with a 10% improvement in word error rate (WER), while also improving WER on a general test set by 1%.
PDF
点此查看论文截图
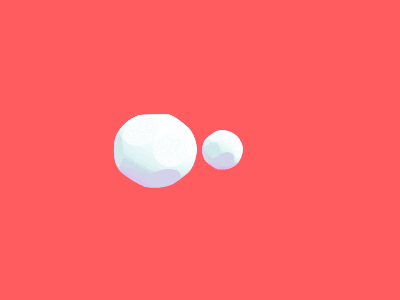
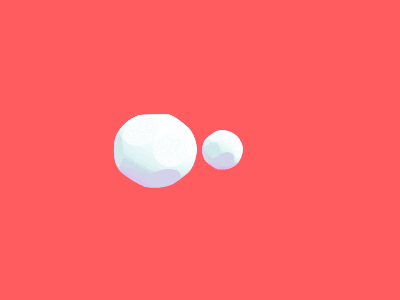
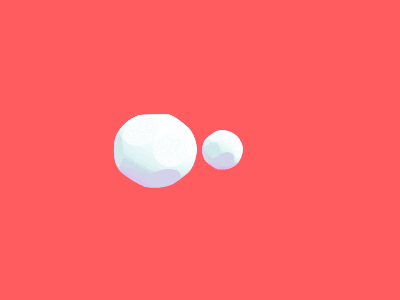
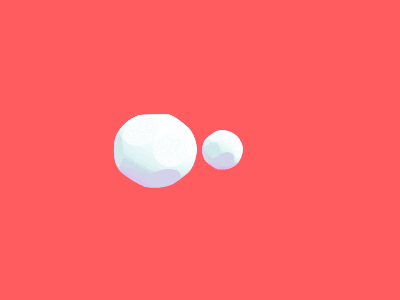
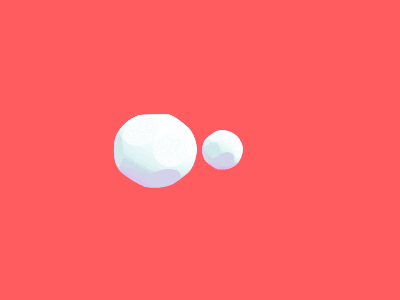