2023-06-22 更新
Chili Pepper Disease Diagnosis via Image Reconstruction Using GrabCut and Generative Adversarial Serial Autoencoder
Authors:Jongwook Si, Sungyoung Kim
With the recent development of smart farms, researchers are very interested in such fields. In particular, the field of disease diagnosis is the most important factor. Disease diagnosis belongs to the field of anomaly detection and aims to distinguish whether plants or fruits are normal or abnormal. The problem can be solved by binary or multi-classification based on CNN, but it can also be solved by image reconstruction. However, due to the limitation of the performance of image generation, SOTA’s methods propose a score calculation method using a latent vector error. In this paper, we propose a network that focuses on chili peppers and proceeds with background removal through Grabcut. It shows high performance through image-based score calculation method. Due to the difficulty of reconstructing the input image, the difference between the input and output images is large. However, the serial autoencoder proposed in this paper uses the difference between the two fake images except for the actual input as a score. We propose a method of generating meaningful images using the GAN structure and classifying three results simultaneously by one discriminator. The proposed method showed higher performance than previous researches, and image-based scores showed the best performanc
PDF 12 pages, 7 figures
点此查看论文截图
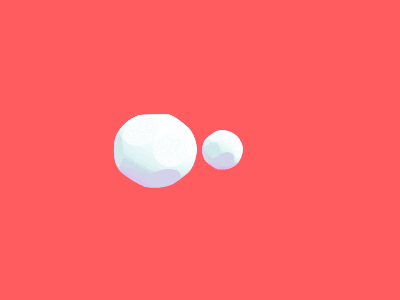
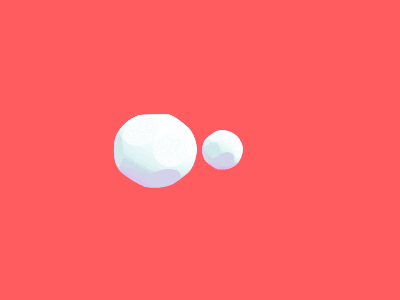
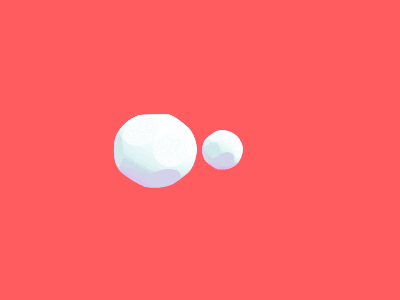
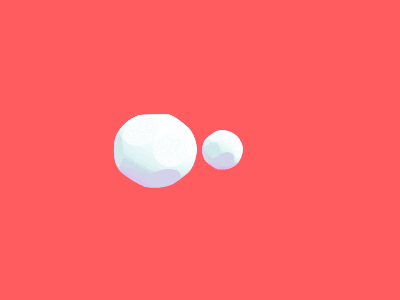
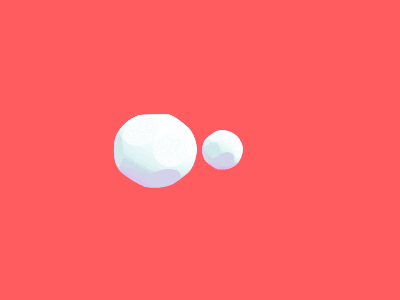
Benchmarking and Analyzing 3D-aware Image Synthesis with a Modularized Codebase
Authors:Qiuyu Wang, Zifan Shi, Kecheng Zheng, Yinghao Xu, Sida Peng, Yujun Shen
Despite the rapid advance of 3D-aware image synthesis, existing studies usually adopt a mixture of techniques and tricks, leaving it unclear how each part contributes to the final performance in terms of generality. Following the most popular and effective paradigm in this field, which incorporates a neural radiance field (NeRF) into the generator of a generative adversarial network (GAN), we build a well-structured codebase, dubbed Carver, through modularizing the generation process. Such a design allows researchers to develop and replace each module independently, and hence offers an opportunity to fairly compare various approaches and recognize their contributions from the module perspective. The reproduction of a range of cutting-edge algorithms demonstrates the availability of our modularized codebase. We also perform a variety of in-depth analyses, such as the comparison across different types of point feature, the necessity of the tailing upsampler in the generator, the reliance on the camera pose prior, etc., which deepen our understanding of existing methods and point out some further directions of the research work. We release code and models at https://github.com/qiuyu96/Carver to facilitate the development and evaluation of this field.
PDF Code: https://github.com/qiuyu96/Carver
点此查看论文截图
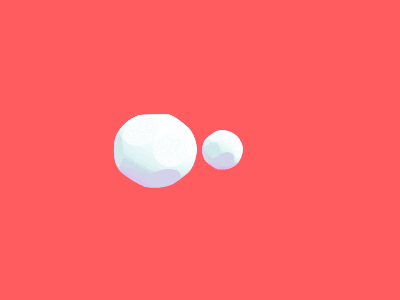
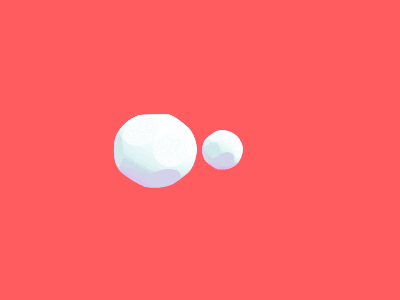
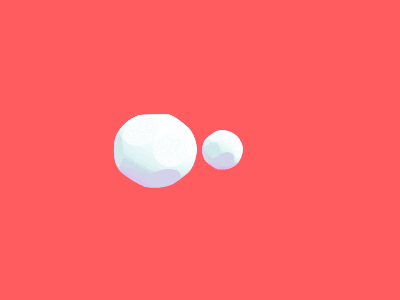
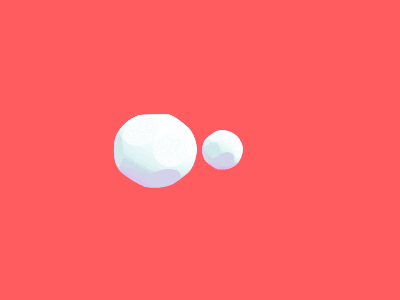
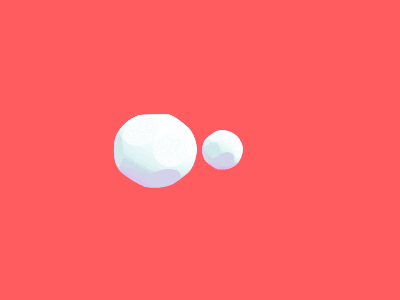
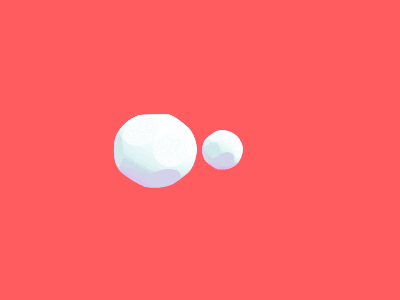