2023-05-29 更新
On the Efficacy and Noise-Robustness of Jointly Learned Speech Emotion and Automatic Speech Recognition
Authors:Lokesh Bansal, S. Pavankumar Dubagunta, Malolan Chetlur, Pushpak Jagtap, Aravind Ganapathiraju
New-age conversational agent systems perform both speech emotion recognition (SER) and automatic speech recognition (ASR) using two separate and often independent approaches for real-world application in noisy environments. In this paper, we investigate a joint ASR-SER multitask learning approach in a low-resource setting and show that improvements are observed not only in SER, but also in ASR. We also investigate the robustness of such jointly trained models to the presence of background noise, babble, and music. Experimental results on the IEMOCAP dataset show that joint learning can improve ASR word error rate (WER) and SER classification accuracy by 10.7% and 2.3% respectively in clean scenarios. In noisy scenarios, results on data augmented with MUSAN show that the joint approach outperforms the independent ASR and SER approaches across many noisy conditions. Overall, the joint ASR-SER approach yielded more noise-resistant models than the independent ASR and SER approaches.
PDF accepted to be part of INTERSPEECH 2023
点此查看论文截图
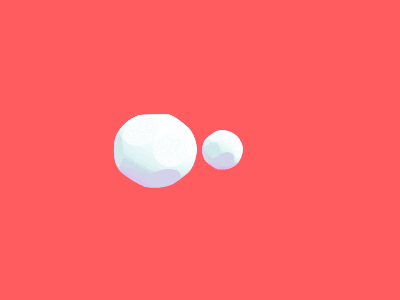
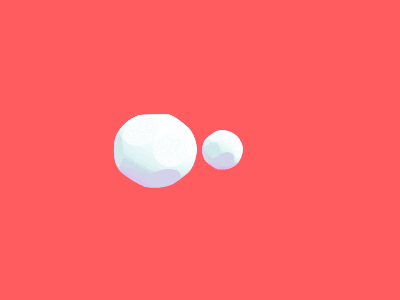
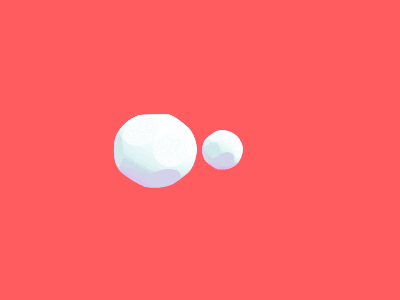
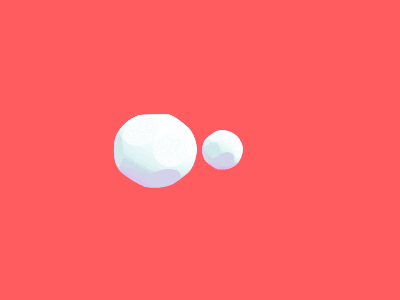
Comparative Study of Pre-Trained BERT Models for Code-Mixed Hindi-English Data
Authors:Aryan Patil, Varad Patwardhan, Abhishek Phaltankar, Gauri Takawane, Raviraj Joshi
The term “Code Mixed” refers to the use of more than one language in the same text. This phenomenon is predominantly observed on social media platforms, with an increasing amount of adaptation as time goes on. It is critical to detect foreign elements in a language and process them correctly, as a considerable number of individuals are using code-mixed languages that could not be comprehended by understanding one of those languages. In this work, we focus on low-resource Hindi-English code-mixed language and enhancing the performance of different code-mixed natural language processing tasks such as sentiment analysis, emotion recognition, and hate speech identification. We perform a comparative analysis of different Transformer-based language Models pre-trained using unsupervised approaches. We have included the code-mixed models like HingBERT, HingRoBERTa, HingRoBERTa-Mixed, mBERT, and non-code-mixed models like AlBERT, BERT, and RoBERTa for comparative analysis of code-mixed Hindi-English downstream tasks. We report state-of-the-art results on respective datasets using HingBERT-based models which are specifically pre-trained on real code-mixed text. Our HingBERT-based models provide significant improvements thus highlighting the poor performance of vanilla BERT models on code-mixed text.
PDF Accepted at IEEE 8th International Conference for Convergence in Technology
点此查看论文截图
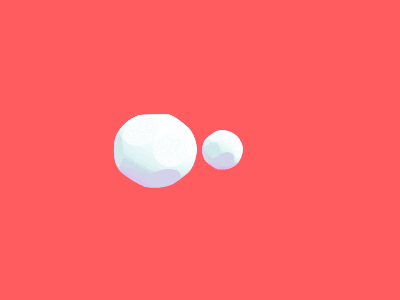
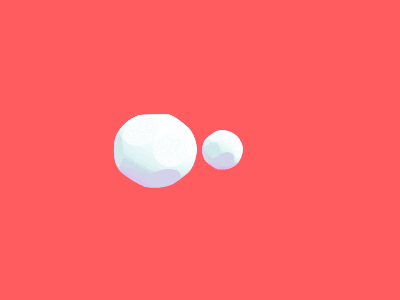
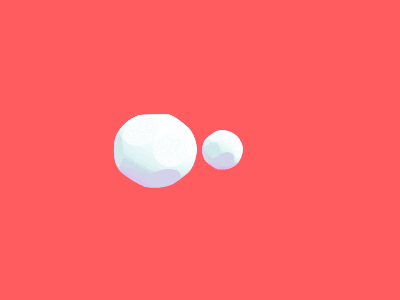
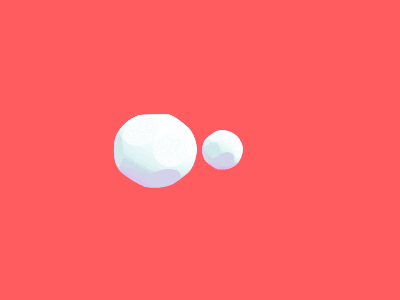
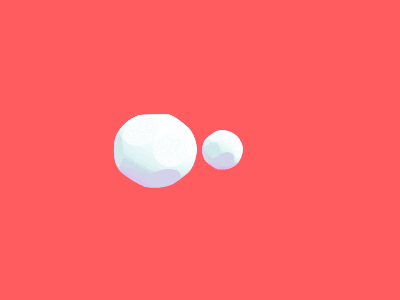
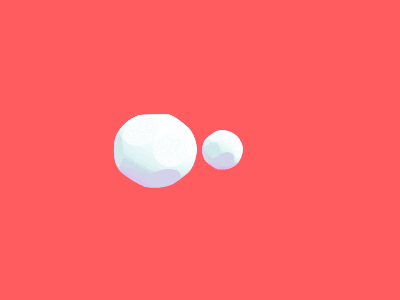
Context-Aware Attention Layers coupled with Optimal Transport Domain Adaptation methods for recognizing dementia from spontaneous speech
Authors:Loukas Ilias, Dimitris Askounis
Alzheimer’s disease (AD) constitutes a complex neurocognitive disease and is the main cause of dementia. Although many studies have been proposed targeting at diagnosing dementia through spontaneous speech, there are still limitations. Existing state-of-the-art approaches, which propose multimodal methods, train separately language and acoustic models, employ majority-vote approaches, and concatenate the representations of the different modalities either at the input level, i.e., early fusion, or during training. Also, some of them employ self-attention layers, which calculate the dependencies between representations without considering the contextual information. In addition, no prior work has taken into consideration the model calibration. To address these limitations, we propose some new methods for detecting AD patients, which capture the intra- and cross-modal interactions. First, we convert the audio files into log-Mel spectrograms, their delta, and delta-delta and create in this way an image per audio file consisting of three channels. Next, we pass each transcript and image through BERT and DeiT models respectively. After that, context-based self-attention layers, self-attention layers with a gate model, and optimal transport domain adaptation methods are employed for capturing the intra- and inter-modal interactions. Finally, we exploit two methods for fusing the self and cross-attended features. For taking into account the model calibration, we apply label smoothing. We use both performance and calibration metrics. Experiments conducted on the ADReSS Challenge dataset indicate the efficacy of our introduced approaches over existing research initiatives with our best performing model reaching Accuracy and F1-score up to 91.25% and 91.06% respectively.
PDF
点此查看论文截图
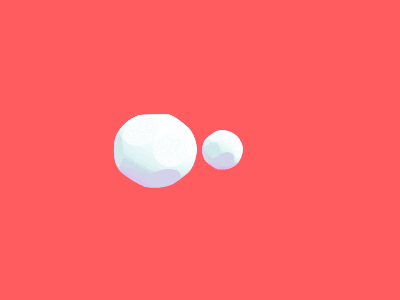
2-bit Conformer quantization for automatic speech recognition
Authors:Oleg Rybakov, Phoenix Meadowlark, Shaojin Ding, David Qiu, Jian Li, David Rim, Yanzhang He
Large speech models are rapidly gaining traction in research community. As a result, model compression has become an important topic, so that these models can fit in memory and be served with reduced cost. Practical approaches for compressing automatic speech recognition (ASR) model use int8 or int4 weight quantization. In this study, we propose to develop 2-bit ASR models. We explore the impact of symmetric and asymmetric quantization combined with sub-channel quantization and clipping on both LibriSpeech dataset and large-scale training data. We obtain a lossless 2-bit Conformer model with 32% model size reduction when compared to state of the art 4-bit Conformer model for LibriSpeech. With the large-scale training data, we obtain a 2-bit Conformer model with over 40% model size reduction against the 4-bit version at the cost of 17% relative word error rate degradation
PDF submitted to Interspeech
点此查看论文截图
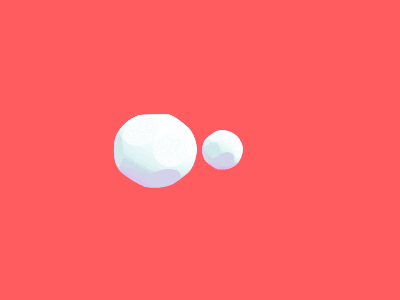
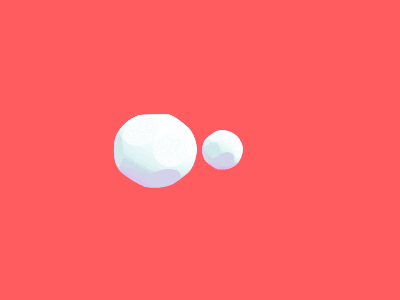
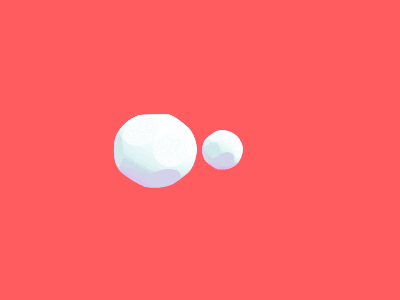
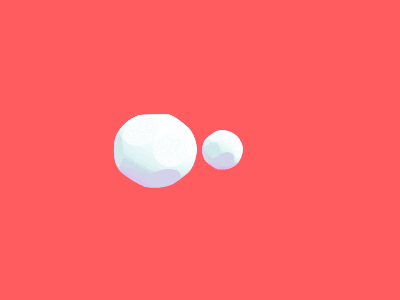
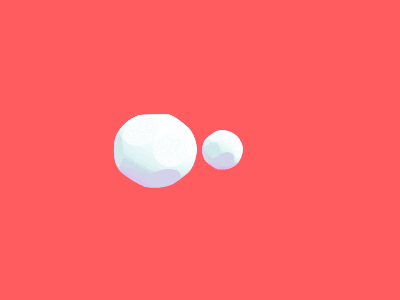
ABC-KD: Attention-Based-Compression Knowledge Distillation for Deep Learning-Based Noise Suppression
Authors:Yixin Wan, Yuan Zhou, Xiulian Peng, Kai-Wei Chang, Yan Lu
Noise suppression (NS) models have been widely applied to enhance speech quality. Recently, Deep Learning-Based NS, which we denote as Deep Noise Suppression (DNS), became the mainstream NS method due to its excelling performance over traditional ones. However, DNS models face 2 major challenges for supporting the real-world applications. First, high-performing DNS models are usually large in size, causing deployment difficulties. Second, DNS models require extensive training data, including noisy audios as inputs and clean audios as labels. It is often difficult to obtain clean labels for training DNS models. We propose the use of knowledge distillation (KD) to resolve both challenges. Our study serves 2 main purposes. To begin with, we are among the first to comprehensively investigate mainstream KD techniques on DNS models to resolve the two challenges. Furthermore, we propose a novel Attention-Based-Compression KD method that outperforms all investigated mainstream KD frameworks on DNS task.
PDF This paper was accepted to Interspeech 2023 Main Conference
点此查看论文截图
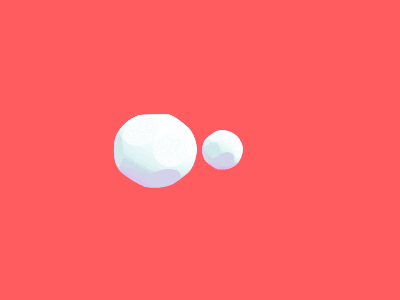
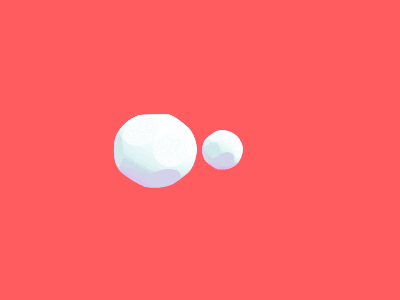
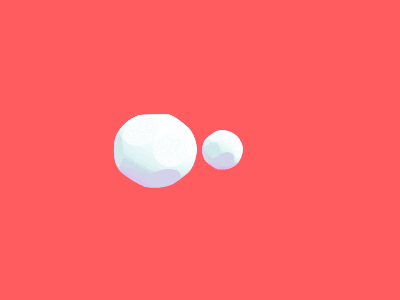
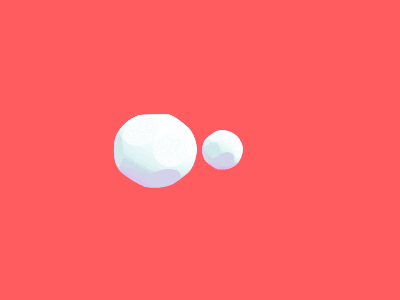