2023-05-18 更新
Dynamic Causal Explanation Based Diffusion-Variational Graph Neural Network for Spatio-temporal Forecasting
Authors:Guojun Liang, Prayag Tiwari, Sławomir Nowaczyk, Stefan Byttner, Fernando Alonso-Fernandez
Graph neural networks (GNNs), especially dynamic GNNs, have become a research hotspot in spatio-temporal forecasting problems. While many dynamic graph construction methods have been developed, relatively few of them explore the causal relationship between neighbour nodes. Thus, the resulting models lack strong explainability for the causal relationship between the neighbour nodes of the dynamically generated graphs, which can easily lead to a risk in subsequent decisions. Moreover, few of them consider the uncertainty and noise of dynamic graphs based on the time series datasets, which are ubiquitous in real-world graph structure networks. In this paper, we propose a novel Dynamic Diffusion-Variational Graph Neural Network (DVGNN) for spatio-temporal forecasting. For dynamic graph construction, an unsupervised generative model is devised. Two layers of graph convolutional network (GCN) are applied to calculate the posterior distribution of the latent node embeddings in the encoder stage. Then, a diffusion model is used to infer the dynamic link probability and reconstruct causal graphs in the decoder stage adaptively. The new loss function is derived theoretically, and the reparameterization trick is adopted in estimating the probability distribution of the dynamic graphs by Evidence Lower Bound during the backpropagation period. After obtaining the generated graphs, dynamic GCN and temporal attention are applied to predict future states. Experiments are conducted on four real-world datasets of different graph structures in different domains. The results demonstrate that the proposed DVGNN model outperforms state-of-the-art approaches and achieves outstanding Root Mean Squared Error result while exhibiting higher robustness. Also, by F1-score and probability distribution analysis, we demonstrate that DVGNN better reflects the causal relationship and uncertainty of dynamic graphs.
PDF
点此查看论文截图
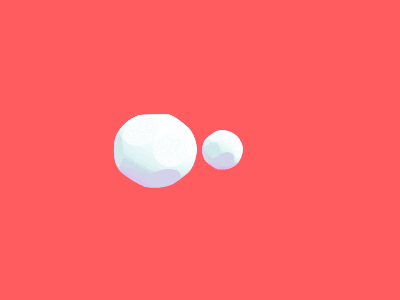
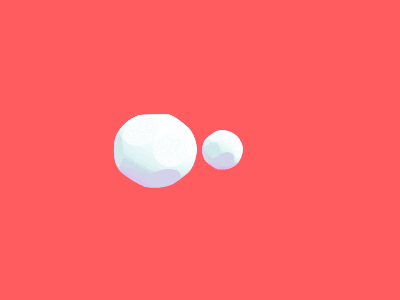
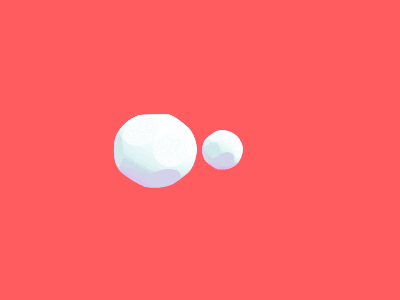
Joint Denoising and Few-angle Reconstruction for Low-dose Cardiac SPECT Using a Dual-domain Iterative Network with Adaptive Data Consistency
Authors:Xiongchao Chen, Bo Zhou, Huidong Xie, Xueqi Guo, Qiong Liu, Albert J. Sinusas, Chi Liu
Myocardial perfusion imaging (MPI) by single-photon emission computed tomography (SPECT) is widely applied for the diagnosis of cardiovascular diseases. Reducing the dose of the injected tracer is essential for lowering the patient’s radiation exposure, but it will lead to increased image noise. Additionally, the latest dedicated cardiac SPECT scanners typically acquire projections in fewer angles using fewer detectors to reduce hardware expenses, potentially resulting in lower reconstruction accuracy. To overcome these challenges, we propose a dual-domain iterative network for end-to-end joint denoising and reconstruction from low-dose and few-angle projections of cardiac SPECT. The image-domain network provides a prior estimate for the projection-domain networks. The projection-domain primary and auxiliary modules are interconnected for progressive denoising and few-angle reconstruction. Adaptive Data Consistency (ADC) modules improve prediction accuracy by efficiently fusing the outputs of the primary and auxiliary modules. Experiments using clinical MPI data show that our proposed method outperforms existing image-, projection-, and dual-domain techniques, producing more accurate projections and reconstructions. Ablation studies confirm the significance of the image-domain prior estimate and ADC modules in enhancing network performance.
PDF 13 pages, 8 figures, 3 tables
点此查看论文截图
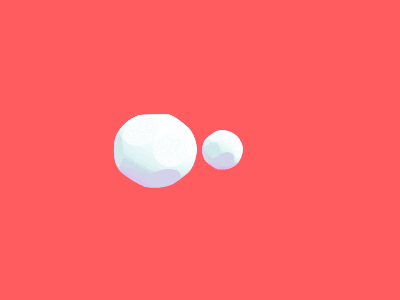
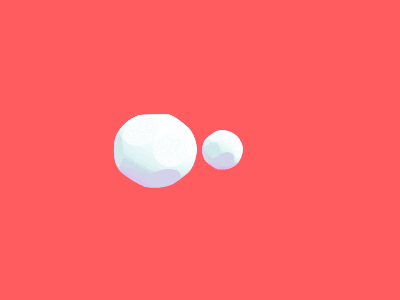
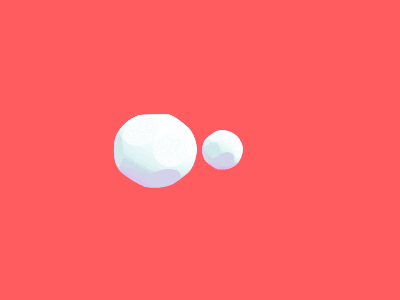
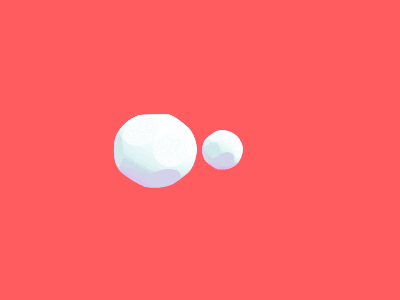