2023-05-15 更新
Investigating the Sensitivity of Automatic Speech Recognition Systems to Phonetic Variation in L2 Englishes
Authors:Emma O’Neill, Julie Carson-Berndsen
Automatic Speech Recognition (ASR) systems exhibit the best performance on speech that is similar to that on which it was trained. As such, underrepresented varieties including regional dialects, minority-speakers, and low-resource languages, see much higher word error rates (WERs) than those varieties seen as ‘prestigious’, ‘mainstream’, or ‘standard’. This can act as a barrier to incorporating ASR technology into the annotation process for large-scale linguistic research since the manual correction of the erroneous automated transcripts can be just as time and resource consuming as manual transcriptions. A deeper understanding of the behaviour of an ASR system is thus beneficial from a speech technology standpoint, in terms of improving ASR accuracy, and from an annotation standpoint, where knowing the likely errors made by an ASR system can aid in this manual correction. This work demonstrates a method of probing an ASR system to discover how it handles phonetic variation across a number of L2 Englishes. Specifically, how particular phonetic realisations which were rare or absent in the system’s training data can lead to phoneme level misrecognitions and contribute to higher WERs. It is demonstrated that the behaviour of the ASR is systematic and consistent across speakers with similar spoken varieties (in this case the same L1) and phoneme substitution errors are typically in agreement with human annotators. By identifying problematic productions specific weaknesses can be addressed by sourcing such realisations for training and fine-tuning thus making the system more robust to pronunciation variation.
PDF
点此查看论文截图
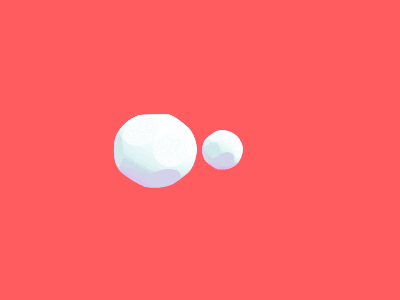
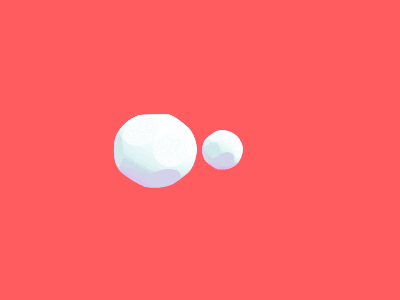
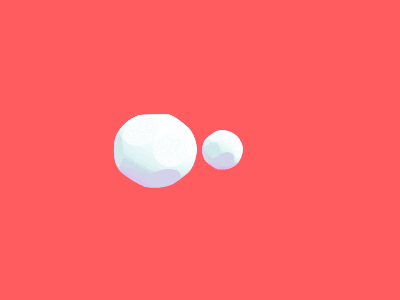
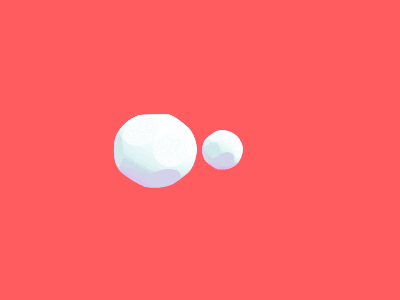
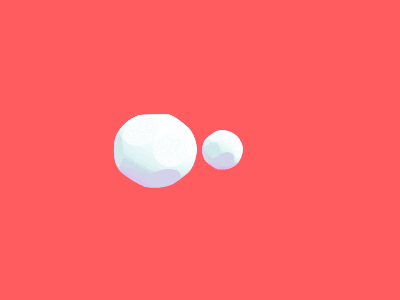
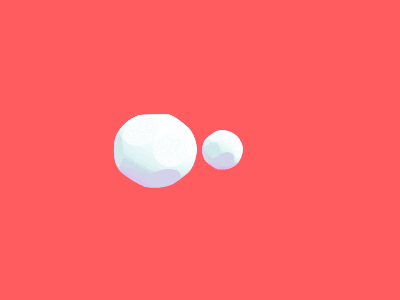