2023-04-24 更新
Distill the Image to Nowhere: Inversion Knowledge Distillation for Multimodal Machine Translation
Authors:Ru Peng, Yawen Zeng, Junbo Zhao
Past works on multimodal machine translation (MMT) elevate bilingual setup by incorporating additional aligned vision information. However, an image-must requirement of the multimodal dataset largely hinders MMT’s development — namely that it demands an aligned form of [image, source text, target text]. This limitation is generally troublesome during the inference phase especially when the aligned image is not provided as in the normal NMT setup. Thus, in this work, we introduce IKD-MMT, a novel MMT framework to support the image-free inference phase via an inversion knowledge distillation scheme. In particular, a multimodal feature generator is executed with a knowledge distillation module, which directly generates the multimodal feature from (only) source texts as the input. While there have been a few prior works entertaining the possibility to support image-free inference for machine translation, their performances have yet to rival the image-must translation. In our experiments, we identify our method as the first image-free approach to comprehensively rival or even surpass (almost) all image-must frameworks, and achieved the state-of-the-art result on the often-used Multi30k benchmark. Our code and data are available at: https://github.com/pengr/IKD-mmt/tree/master..
PDF EMNLP2022 Oral Long paper
点此查看论文截图
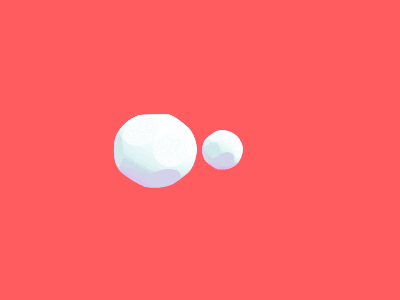
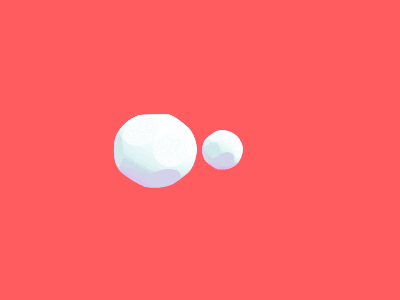
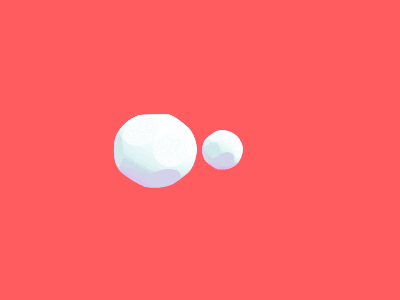
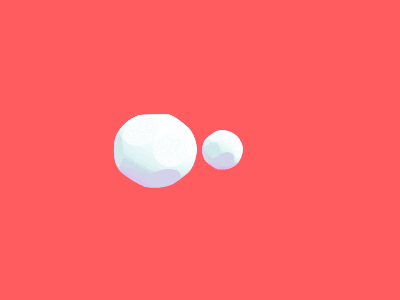