2023-04-19 更新
MA-ViT: Modality-Agnostic Vision Transformers for Face Anti-Spoofing
Authors:Ajian Liu, Yanyan Liang
The existing multi-modal face anti-spoofing (FAS) frameworks are designed based on two strategies: halfway and late fusion. However, the former requires test modalities consistent with the training input, which seriously limits its deployment scenarios. And the latter is built on multiple branches to process different modalities independently, which limits their use in applications with low memory or fast execution requirements. In this work, we present a single branch based Transformer framework, namely Modality-Agnostic Vision Transformer (MA-ViT), which aims to improve the performance of arbitrary modal attacks with the help of multi-modal data. Specifically, MA-ViT adopts the early fusion to aggregate all the available training modalities data and enables flexible testing of any given modal samples. Further, we develop the Modality-Agnostic Transformer Block (MATB) in MA-ViT, which consists of two stacked attentions named Modal-Disentangle Attention (MDA) and Cross-Modal Attention (CMA), to eliminate modality-related information for each modal sequences and supplement modality-agnostic liveness features from another modal sequences, respectively. Experiments demonstrate that the single model trained based on MA-ViT can not only flexibly evaluate different modal samples, but also outperforms existing single-modal frameworks by a large margin, and approaches the multi-modal frameworks introduced with smaller FLOPs and model parameters.
PDF 7 pages, 4 figures, conference
点此查看论文截图
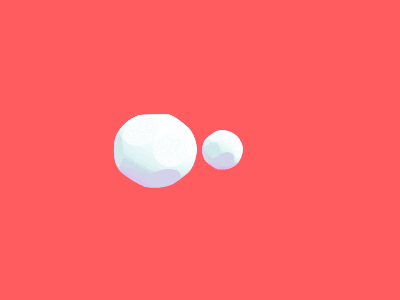
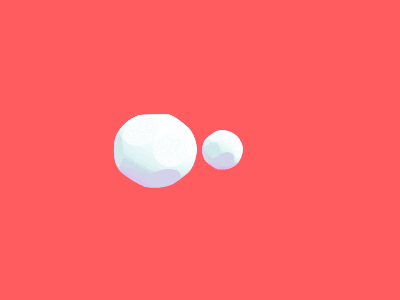
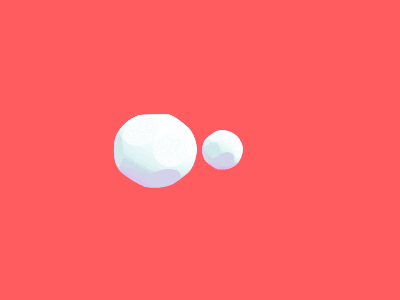
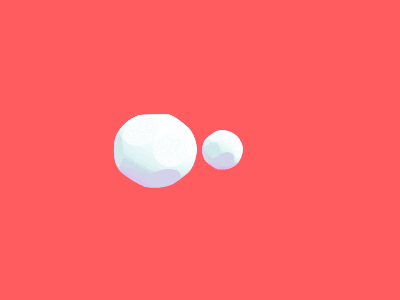
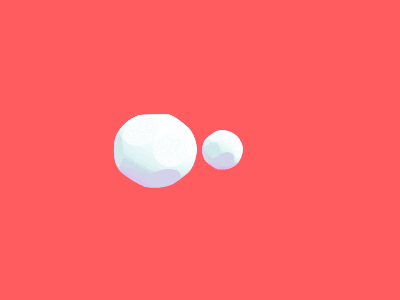