2023-04-13 更新
Modality-Invariant Representation for Infrared and Visible Image Registration
Authors:Zhiying Jiang, Zengxi Zhang, Jinyuan Liu, Xin Fan, Risheng Liu
Since the differences in viewing range, resolution and relative position, the multi-modality sensing module composed of infrared and visible cameras needs to be registered so as to have more accurate scene perception. In practice, manual calibration-based registration is the most widely used process, and it is regularly calibrated to maintain accuracy, which is time-consuming and labor-intensive. To cope with these problems, we propose a scene-adaptive infrared and visible image registration. Specifically, in regard of the discrepancy between multi-modality images, an invertible translation process is developed to establish a modality-invariant domain, which comprehensively embraces the feature intensity and distribution of both infrared and visible modalities. We employ homography to simulate the deformation between different planes and develop a hierarchical framework to rectify the deformation inferred from the proposed latent representation in a coarse-to-fine manner. For that, the advanced perception ability coupled with the residual estimation conducive to the regression of sparse offsets, and the alternate correlation search facilitates a more accurate correspondence matching. Moreover, we propose the first ground truth available misaligned infrared and visible image dataset, involving three synthetic sets and one real-world set. Extensive experiments validate the effectiveness of the proposed method against the state-of-the-arts, advancing the subsequent applications.
PDF 10 pages, 11 figures
点此查看论文截图
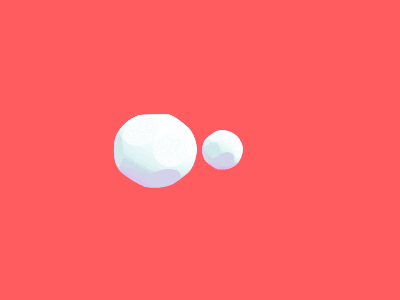
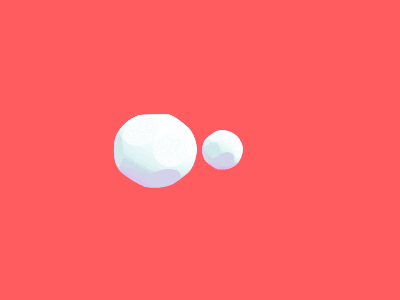
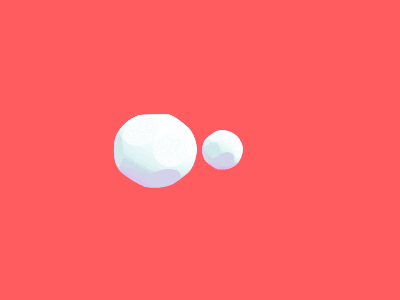
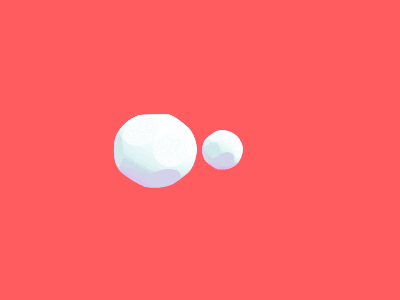
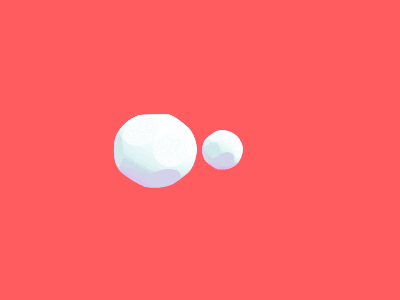
Scale-Equivariant Deep Learning for 3D Data
Authors:Thomas Wimmer, Vladimir Golkov, Hoai Nam Dang, Moritz Zaiss, Andreas Maier, Daniel Cremers
The ability of convolutional neural networks (CNNs) to recognize objects regardless of their position in the image is due to the translation-equivariance of the convolutional operation. Group-equivariant CNNs transfer this equivariance to other transformations of the input. Dealing appropriately with objects and object parts of different scale is challenging, and scale can vary for multiple reasons such as the underlying object size or the resolution of the imaging modality. In this paper, we propose a scale-equivariant convolutional network layer for three-dimensional data that guarantees scale-equivariance in 3D CNNs. Scale-equivariance lifts the burden of having to learn each possible scale separately, allowing the neural network to focus on higher-level learning goals, which leads to better results and better data-efficiency. We provide an overview of the theoretical foundations and scientific work on scale-equivariant neural networks in the two-dimensional domain. We then transfer the concepts from 2D to the three-dimensional space and create a scale-equivariant convolutional layer for 3D data. Using the proposed scale-equivariant layer, we create a scale-equivariant U-Net for medical image segmentation and compare it with a non-scale-equivariant baseline method. Our experiments demonstrate the effectiveness of the proposed method in achieving scale-equivariance for 3D medical image analysis. We publish our code at https://github.com/wimmerth/scale-equivariant-3d-convnet for further research and application.
PDF 12 pages, 4 figures
点此查看论文截图
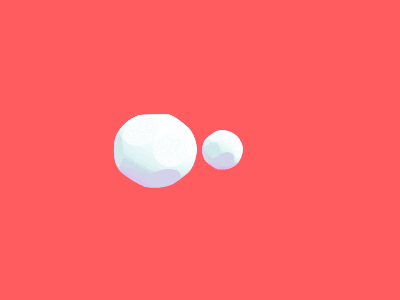
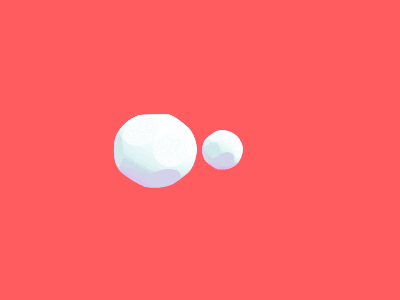
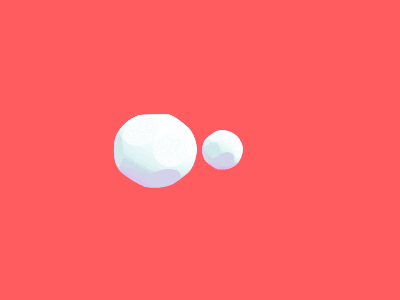