2023-03-29 更新
Depth- and Semantics-aware Multi-modal Domain Translation: Generating 3D Panoramic Color Images from LiDAR Point Clouds
Authors:Tiago Cortinhal, Eren Erdal Aksoy
This work presents a new depth- and semantics-aware conditional generative model, named TITAN-Next, for cross-domain image-to-image translation in a multi-modal setup between LiDAR and camera sensors. The proposed model leverages scene semantics as a mid-level representation and is able to translate raw LiDAR point clouds to RGB-D camera images by solely relying on semantic scene segments. We claim that this is the first framework of its kind and it has practical applications in autonomous vehicles such as providing a fail-safe mechanism and augmenting available data in the target image domain. The proposed model is evaluated on the large-scale and challenging Semantic-KITTI dataset, and experimental findings show that it considerably outperforms the original TITAN-Net and other strong baselines by 23.7$\%$ margin in terms of IoU.
PDF
点此查看论文截图
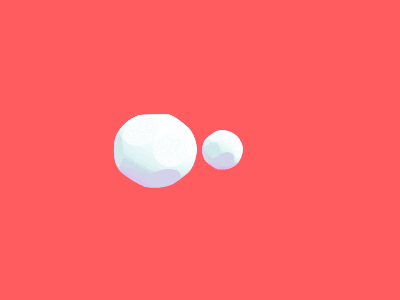
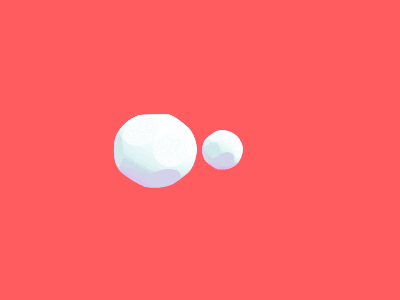
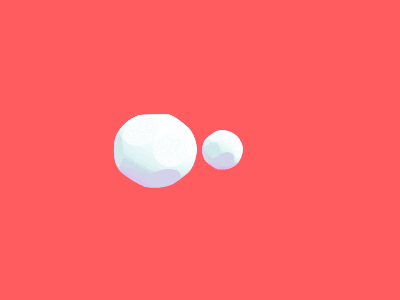
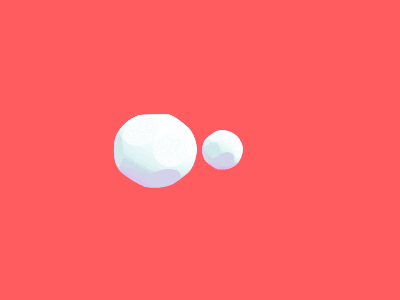
Indonesian Text-to-Image Synthesis with Sentence-BERT and FastGAN
Authors:Made Raharja Surya Mahadi, Nugraha Priya Utama
Currently, text-to-image synthesis uses text encoder and image generator architecture. Research on this topic is challenging. This is because of the domain gap between natural language and vision. Nowadays, most research on this topic only focuses on producing a photo-realistic image, but the other domain, in this case, is the language, which is less concentrated. A lot of the current research uses English as the input text. Besides, there are many languages around the world. Bahasa Indonesia, as the official language of Indonesia, is quite popular. This language has been taught in Philipines, Australia, and Japan. Translating or recreating a new dataset into another language with good quality will cost a lot. Research on this domain is necessary because we need to examine how the image generator performs in other languages besides generating photo-realistic images. To achieve this, we translate the CUB dataset into Bahasa using google translate and manually by humans. We use Sentence BERT as the text encoder and FastGAN as the image generator. FastGAN uses lots of skip excitation modules and auto-encoder to generate an image with resolution 512x512x3, which is twice as bigger as the current state-of-the-art model (Zhang, Xu, Li, Zhang, Wang, Huang and Metaxas, 2019). We also get 4.76 +- 0.43 and 46.401 on Inception Score and Fr\’echet inception distance, respectively, and comparable with the current English text-to-image generation models. The mean opinion score also gives as 3.22 out of 5, which means the generated image is acceptable by humans. Link to source code: https://github.com/share424/Indonesian-Text-to-Image-synthesis-with-Sentence-BERT-and-FastGAN
PDF 11 pages, 3 figures
点此查看论文截图
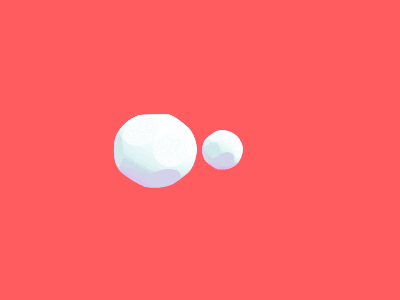
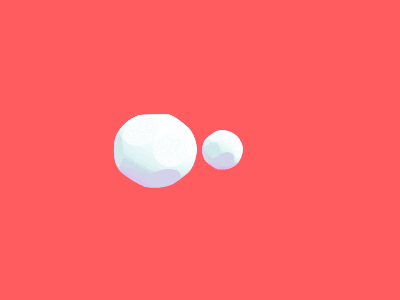
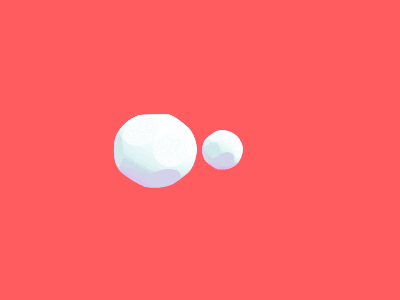
Lightweight Estimation of Hand Mesh and Biomechanically Feasible Kinematic Parameters
Authors:Zhipeng Fan, Yao Wang
3D hand pose estimation is a long-standing challenge in both robotics and computer vision communities due to its implicit depth ambiguity and often strong self-occlusion. Recently, in addition to the hand skeleton, jointly estimating hand pose and shape has gained more attraction. State-of-the-art methods adopt a model-free approach, estimating the vertices of the hand mesh directly and providing superior accuracy compared to traditional model-based methods directly regressing the parameters of the parametric hand mesh. However, with the large number of mesh vertices to estimate, these methods are often slow in inference. We propose an efficient variation of the previously proposed image-to-lixel approach to efficiently estimate hand meshes from the images. Leveraging recent developments in efficient neural architectures, we significantly reduce the computation complexity without sacrificing the estimation accuracy. Furthermore, we introduce an inverted kinematic(IK) network to translate the estimated hand mesh to a biomechanically feasible set of joint rotation parameters, which is necessary for applications that leverage pose estimation for controlling robotic hands. Finally, an optional post-processing module is proposed to refine the rotation and shape parameters to compensate for the error introduced by the IK net. Our Lite I2L Mesh Net achieves state-of-the-art joint and mesh estimation accuracy with less than $13\%$ of the total computational complexity of the original I2L hand mesh estimator. Adding the IK net and post-optimization modules can improve the accuracy slightly at a small computation cost, but more importantly, provide the kinematic parameters required for robotic applications.
PDF
点此查看论文截图
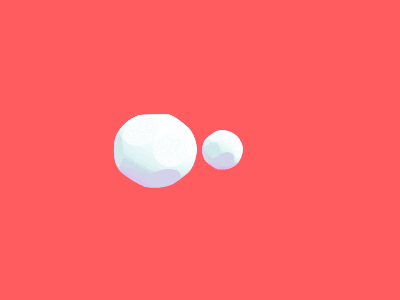
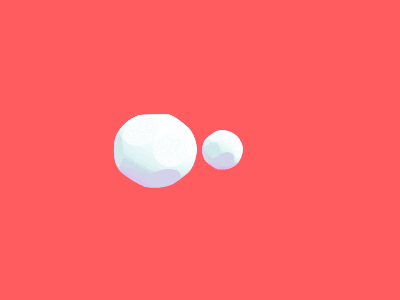
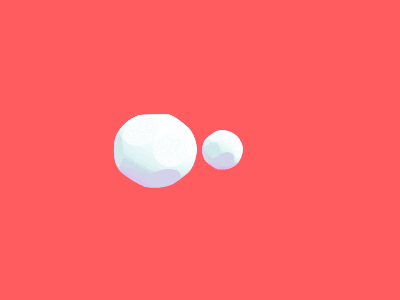
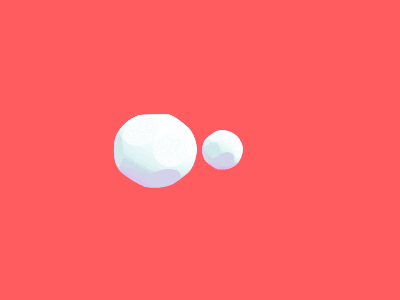
3D-Aware Multi-Class Image-to-Image Translation with NeRFs
Authors:Senmao Li, Joost van de Weijer, Yaxing Wang, Fahad Shahbaz Khan, Meiqin Liu, Jian Yang
Recent advances in 3D-aware generative models (3D-aware GANs) combined with Neural Radiance Fields (NeRF) have achieved impressive results. However no prior works investigate 3D-aware GANs for 3D consistent multi-class image-to-image (3D-aware I2I) translation. Naively using 2D-I2I translation methods suffers from unrealistic shape/identity change. To perform 3D-aware multi-class I2I translation, we decouple this learning process into a multi-class 3D-aware GAN step and a 3D-aware I2I translation step. In the first step, we propose two novel techniques: a new conditional architecture and an effective training strategy. In the second step, based on the well-trained multi-class 3D-aware GAN architecture, that preserves view-consistency, we construct a 3D-aware I2I translation system. To further reduce the view-consistency problems, we propose several new techniques, including a U-net-like adaptor network design, a hierarchical representation constrain and a relative regularization loss. In extensive experiments on two datasets, quantitative and qualitative results demonstrate that we successfully perform 3D-aware I2I translation with multi-view consistency.
PDF Accepted by CVPR2023
点此查看论文截图
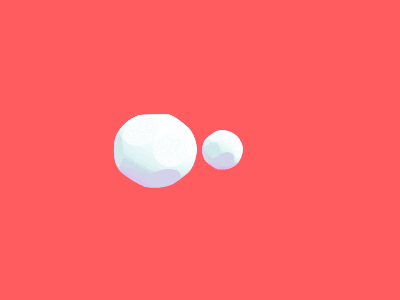
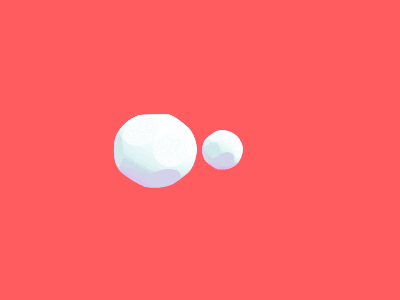
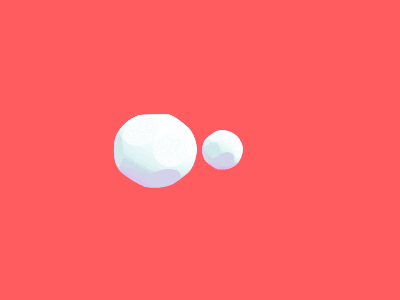
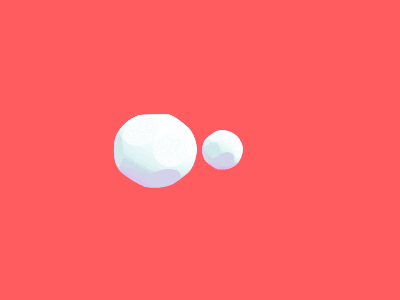
Training-free Style Transfer Emerges from h-space in Diffusion models
Authors:Jaeseok Jeong, Mingi Kwon, Youngjung Uh
Diffusion models (DMs) synthesize high-quality images in various domains. However, controlling their generative process is still hazy because the intermediate variables in the process are not rigorously studied. Recently, StyleCLIP-like editing of DMs is found in the bottleneck of the U-Net, named $h$-space. In this paper, we discover that DMs inherently have disentangled representations for content and style of the resulting images: $h$-space contains the content and the skip connections convey the style. Furthermore, we introduce a principled way to inject content of one image to another considering progressive nature of the generative process. Briefly, given the original generative process, 1) the feature of the source content should be gradually blended, 2) the blended feature should be normalized to preserve the distribution, 3) the change of skip connections due to content injection should be calibrated. Then, the resulting image has the source content with the style of the original image just like image-to-image translation. Interestingly, injecting contents to styles of unseen domains produces harmonization-like style transfer. To the best of our knowledge, our method introduces the first training-free feed-forward style transfer only with an unconditional pretrained frozen generative network. The code is available at https://curryjung.github.io/DiffStyle/.
PDF
点此查看论文截图
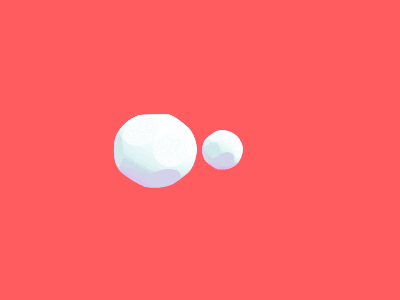
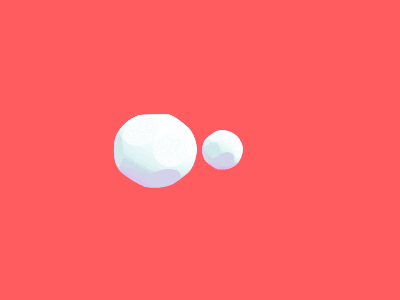
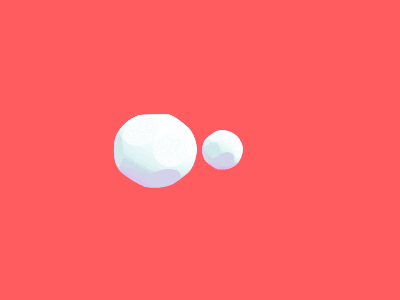
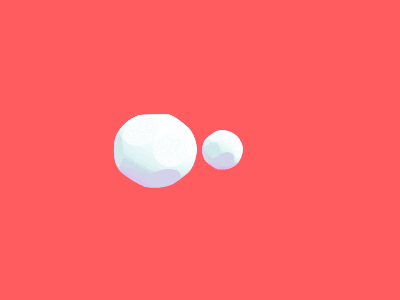
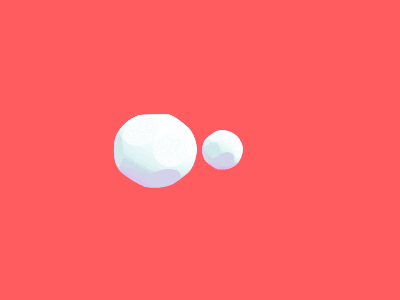