2023-03-16 更新
Real Face Foundation Representation Learning for Generalized Deepfake Detection
Authors:Liang Shi, Jie Zhang, Shiguang Shan
The emergence of deepfake technologies has become a matter of social concern as they pose threats to individual privacy and public security. It is now of great significance to develop reliable deepfake detectors. However, with numerous face manipulation algorithms present, it is almost impossible to collect sufficient representative fake faces, and it is hard for existing detectors to generalize to all types of manipulation. Therefore, we turn to learn the distribution of real faces, and indirectly identify fake images that deviate from the real face distribution. In this study, we propose Real Face Foundation Representation Learning (RFFR), which aims to learn a general representation from large-scale real face datasets and detect potential artifacts outside the distribution of RFFR. Specifically, we train a model on real face datasets by masked image modeling (MIM), which results in a discrepancy between input faces and the reconstructed ones when applying the model on fake samples. This discrepancy reveals the low-level artifacts not contained in RFFR, making it easier to build a deepfake detector sensitive to all kinds of potential artifacts outside the distribution of RFFR. Extensive experiments demonstrate that our method brings about better generalization performance, as it significantly outperforms the state-of-the-art methods in cross-manipulation evaluations, and has the potential to further improve by introducing extra real faces for training RFFR.
PDF 12 pages, 5 figures, 9 tables
点此查看论文截图
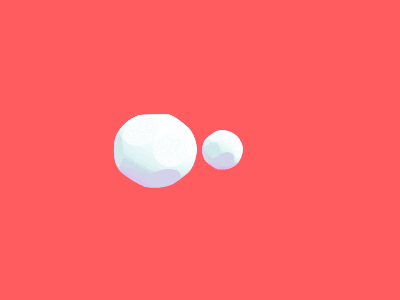
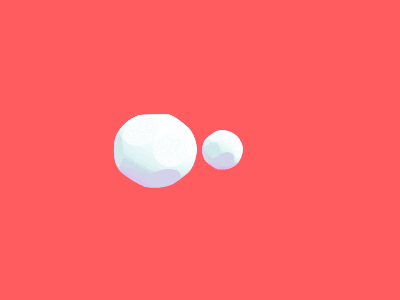
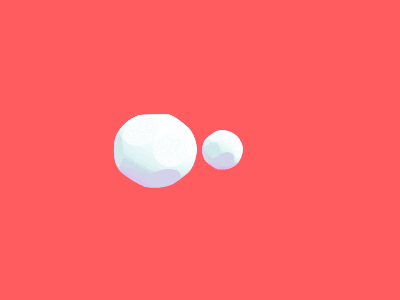
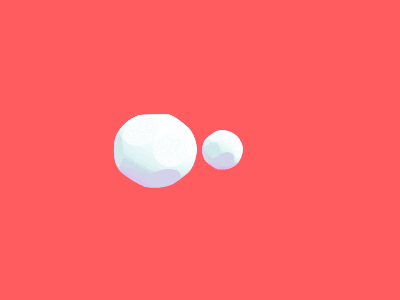
Identity-Preserving Knowledge Distillation for Low-resolution Face Recognition
Authors:Yuhang Lu, Touradj Ebrahimi
Low-resolution face recognition (LRFR) has become a challenging problem for modern deep face recognition systems. Existing methods mainly leverage prior information from high-resolution (HR) images by either reconstructing facial details with super-resolution techniques or learning a unified feature space. To address this issue, this paper proposes a novel approach which enforces the network to focus on the discriminative information stored in the low-frequency components of a low-resolution (LR) image. A cross-resolution knowledge distillation paradigm is first employed as the learning framework. An identity-preserving network, WaveResNet, and a wavelet similarity loss are then designed to capture low-frequency details and boost performance. Finally, an image degradation model is conceived to simulate more realistic LR training data. Consequently, extensive experimental results show that the proposed method consistently outperforms the baseline model and other state-of-the-art methods across a variety of image resolutions.
PDF
点此查看论文截图
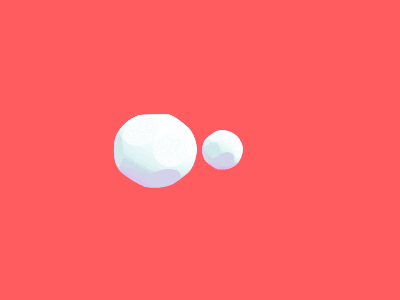
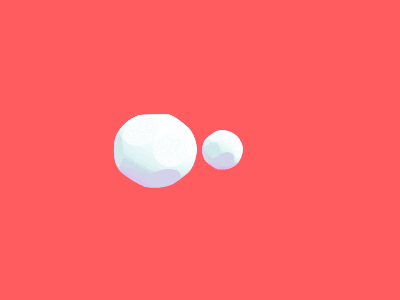
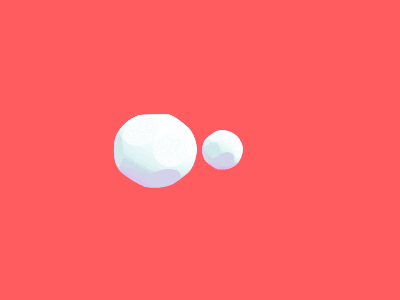
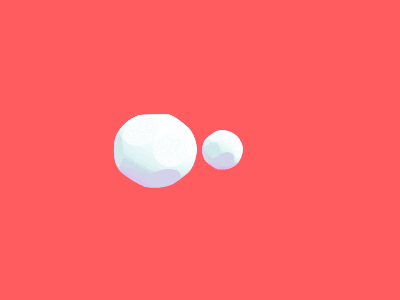
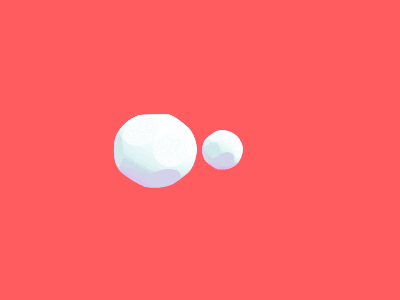