2022-12-29 更新
Spacecraft Pose Estimation Based on Unsupervised Domain Adaptation and on a 3D-Guided Loss Combination
Authors:Juan Ignacio Bravo Pérez-Villar, Álvaro García-Martín, Jesús Bescós
Spacecraft pose estimation is a key task to enable space missions in which two spacecrafts must navigate around each other. Current state-of-the-art algorithms for pose estimation employ data-driven techniques. However, there is an absence of real training data for spacecraft imaged in space conditions due to the costs and difficulties associated with the space environment. This has motivated the introduction of 3D data simulators, solving the issue of data availability but introducing a large gap between the training (source) and test (target) domains. We explore a method that incorporates 3D structure into the spacecraft pose estimation pipeline to provide robustness to intensity domain shift and we present an algorithm for unsupervised domain adaptation with robust pseudo-labelling. Our solution has ranked second in the two categories of the 2021 Pose Estimation Challenge organised by the European Space Agency and the Stanford University, achieving the lowest average error over the two categories.
PDF Accepted at ECCV 2022 AI4SPACE Workshop (https://aiforspace.github.io/2022/)
点此查看论文截图
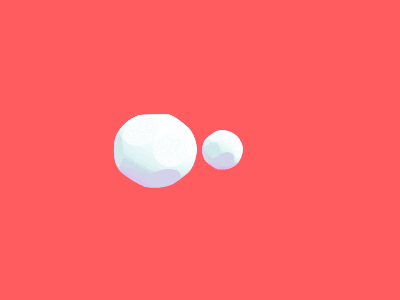
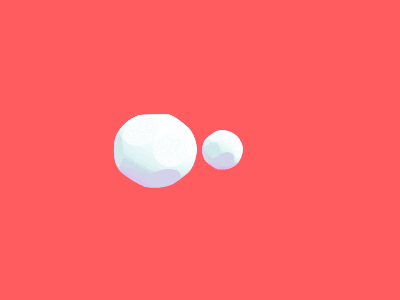
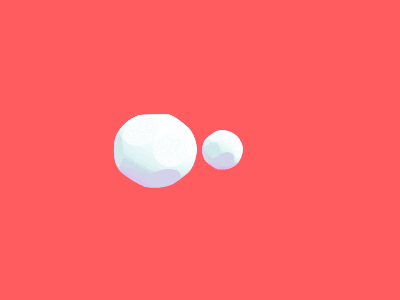