2022-12-14 更新
Computer Vision for Transit Travel Time Prediction: An End-to-End Framework Using Roadside Urban Imagery
Authors:Awad Abdelhalim, Jinhua Zhao
Accurate travel time estimation is paramount for providing transit users with reliable schedules and dependable real-time information. This paper is the first to utilize roadside urban imagery for direct transit travel time prediction. We propose and evaluate an end-to-end framework integrating traditional transit data sources with a roadside camera for automated roadside image data acquisition, labeling, and model training to predict transit travel times across a segment of interest. First, we show how the GTFS real-time data can be utilized as an efficient activation mechanism for a roadside camera unit monitoring a segment of interest. Second, AVL data is utilized to generate ground truth labels for the acquired images based on the observed transit travel time percentiles across the camera-monitored segment during the time of image acquisition. Finally, the generated labeled image dataset is used to train and thoroughly evaluate a Vision Transformer (ViT) model to predict a discrete transit travel time range (band). The results illustrate that the ViT model is able to learn image features and contents that best help it deduce the expected travel time range with an average validation accuracy ranging between 80%-85%. We assess the interpretability of the ViT model’s predictions and showcase how this discrete travel time band prediction can subsequently improve continuous transit travel time estimation. The workflow and results presented in this study provide an end-to-end, scalable, automated, and highly efficient approach for integrating traditional transit data sources and roadside imagery to improve the estimation of transit travel duration. This work also demonstrates the value of incorporating real-time information from computer-vision sources, which are becoming increasingly accessible and can have major implications for improving operations and passenger real-time information.
PDF Final revised preprint
点此查看论文截图
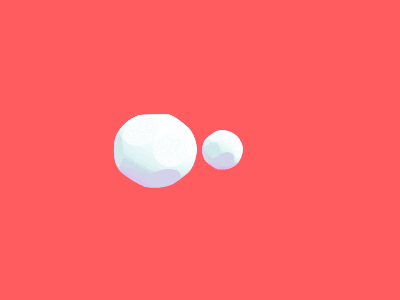
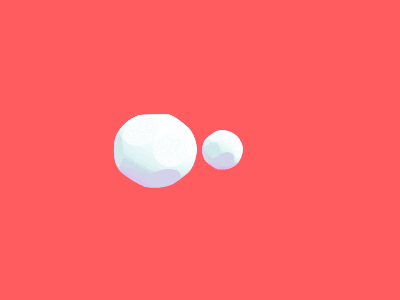
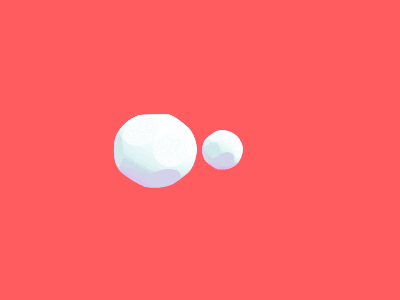
Masked autoencoders are effective solution to transformer data-hungry
Authors:Jiawei Mao, Honggu Zhou, Xuesong Yin, Yuanqi Chang. Binling Nie. Rui Xu
Vision Transformers (ViTs) outperforms convolutional neural networks (CNNs) in several vision tasks with its global modeling capabilities. However, ViT lacks the inductive bias inherent to convolution making it require a large amount of data for training. This results in ViT not performing as well as CNNs on small datasets like medicine and science. We experimentally found that masked autoencoders (MAE) can make the transformer focus more on the image itself, thus alleviating the data-hungry issue of ViT to some extent. Yet the current MAE model is too complex resulting in over-fitting problems on small datasets. This leads to a gap between MAEs trained on small datasets and advanced CNNs models still. Therefore, we investigated how to reduce the decoder complexity in MAE and found a more suitable architectural configuration for it with small datasets. Besides, we additionally designed a location prediction task and a contrastive learning task to introduce localization and invariance characteristics for MAE. Our contrastive learning task not only enables the model to learn high-level visual information but also allows the training of MAE’s class token. This is something that most MAE improvement efforts do not consider. Extensive experiments have shown that our method shows state-of-the-art performance on standard small datasets as well as medical datasets with few samples compared to the current popular masked image modeling (MIM) and vision transformers for small datasets.The code and models are available at https://github.com/Talented-Q/SDMAE.
PDF
点此查看论文截图
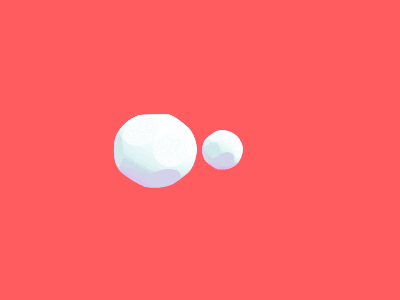
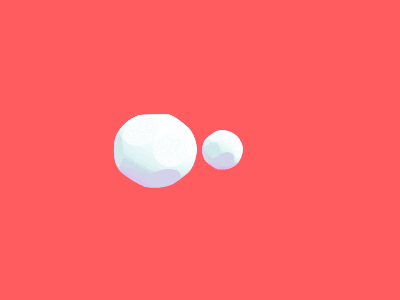
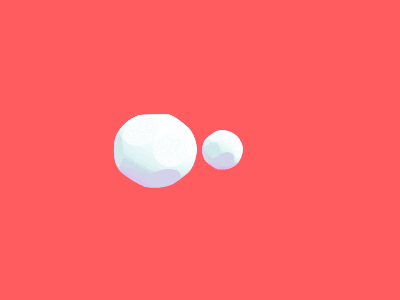
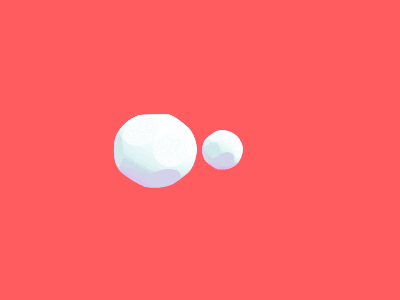
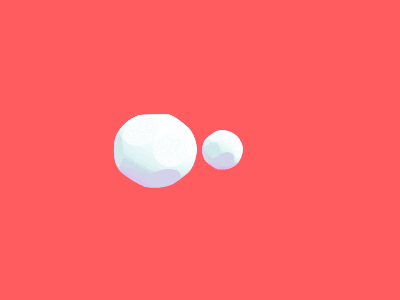
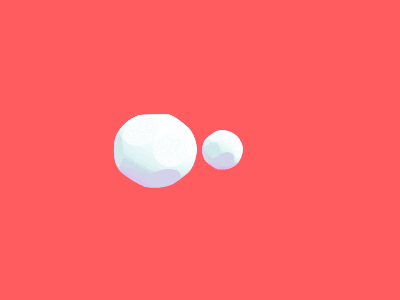