2022-11-30 更新
Scene Text Image Super-Resolution via Content Perceptual Loss and Criss-Cross Transformer Blocks
Authors:Rui Qin, Bin Wang, Yu-Wing Tai
Text image super-resolution is a unique and important task to enhance readability of text images to humans. It is widely used as pre-processing in scene text recognition. However, due to the complex degradation in natural scenes, recovering high-resolution texts from the low-resolution inputs is ambiguous and challenging. Existing methods mainly leverage deep neural networks trained with pixel-wise losses designed for natural image reconstruction, which ignore the unique character characteristics of texts. A few works proposed content-based losses. However, they only focus on text recognizers’ accuracy, while the reconstructed images may still be ambiguous to humans. Further, they often have weak generalizability to handle cross languages. To this end, we present TATSR, a Text-Aware Text Super-Resolution framework, which effectively learns the unique text characteristics using Criss-Cross Transformer Blocks (CCTBs) and a novel Content Perceptual (CP) Loss. The CCTB extracts vertical and horizontal content information from text images by two orthogonal transformers, respectively. The CP Loss supervises the text reconstruction with content semantics by multi-scale text recognition features, which effectively incorporates content awareness into the framework. Extensive experiments on various language datasets demonstrate that TATSR outperforms state-of-the-art methods in terms of both recognition accuracy and human perception.
PDF
点此查看论文截图
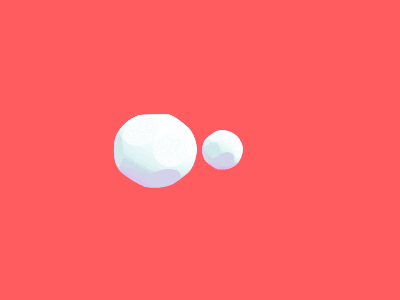
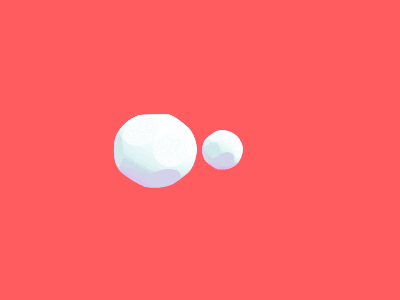
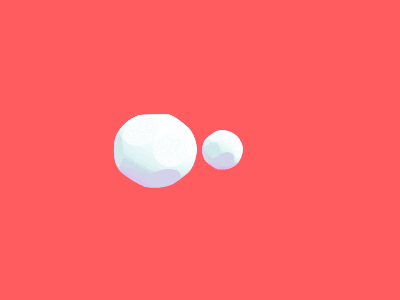
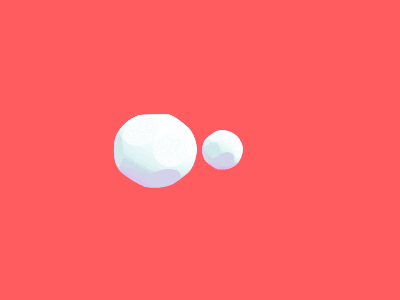
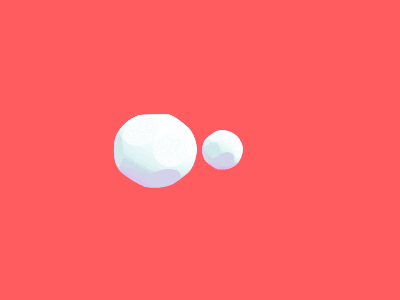
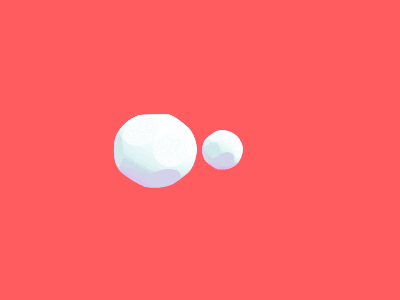
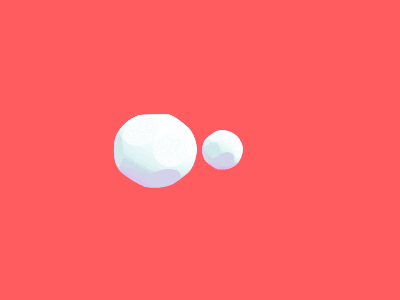
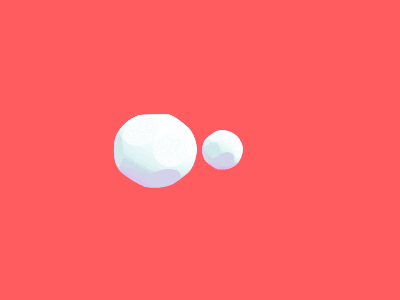
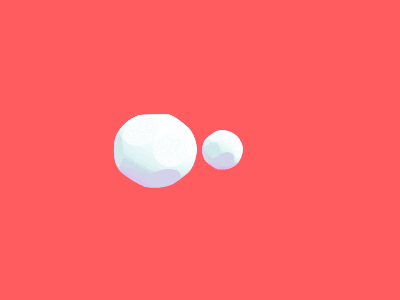
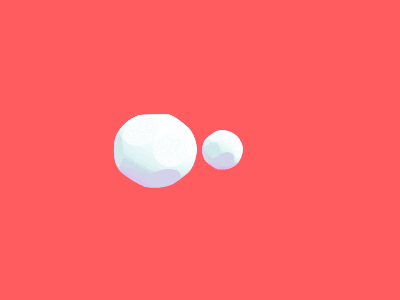
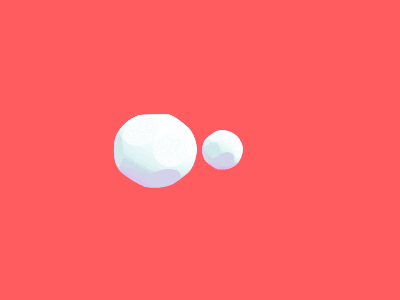
Multi-Granularity Prediction for Scene Text Recognition
Authors:Peng Wang, Cheng Da, Cong Yao
Scene text recognition (STR) has been an active research topic in computer vision for years. To tackle this challenging problem, numerous innovative methods have been successively proposed and incorporating linguistic knowledge into STR models has recently become a prominent trend. In this work, we first draw inspiration from the recent progress in Vision Transformer (ViT) to construct a conceptually simple yet powerful vision STR model, which is built upon ViT and outperforms previous state-of-the-art models for scene text recognition, including both pure vision models and language-augmented methods. To integrate linguistic knowledge, we further propose a Multi-Granularity Prediction strategy to inject information from the language modality into the model in an implicit way, i.e. , subword representations (BPE and WordPiece) widely-used in NLP are introduced into the output space, in addition to the conventional character level representation, while no independent language model (LM) is adopted. The resultant algorithm (termed MGP-STR) is able to push the performance envelop of STR to an even higher level. Specifically, it achieves an average recognition accuracy of 93.35% on standard benchmarks. Code is available at https://github.com/AlibabaResearch/AdvancedLiterateMachinery/tree/main/OCR/MGP-STR.
PDF Accepted by ECCV2022
点此查看论文截图
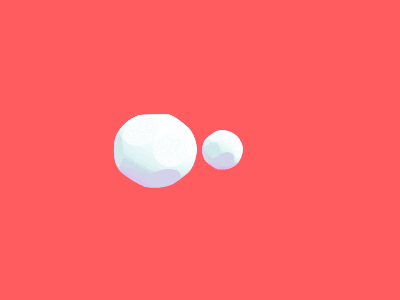
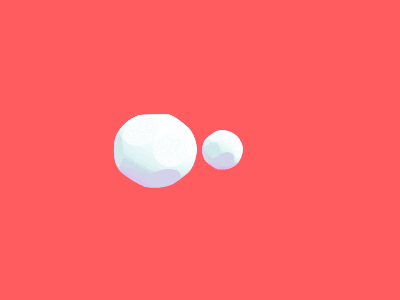
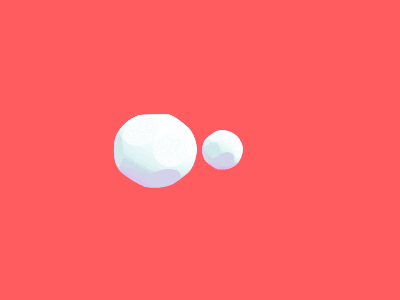
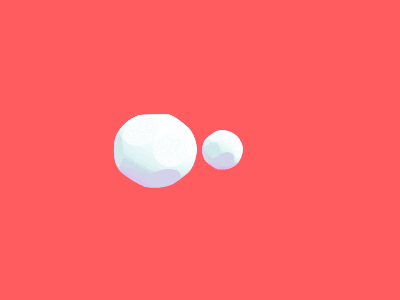
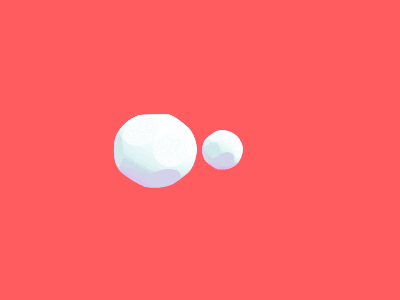
TPSNet: Reverse Thinking of Thin Plate Splines for Arbitrary Shape Scene Text Representation
Authors:Wei Wang, Yu Zhou, Jiahao Lv, Dayan Wu, Guoqing Zhao, Ning Jiang, Weiping Wang
The research focus of scene text detection and recognition has shifted to arbitrary shape text in recent years, where the text shape representation is a fundamental problem. An ideal representation should be compact, complete, efficient, and reusable for subsequent recognition in our opinion. However, previous representations have flaws in one or more aspects. Thin-Plate-Spline (TPS) transformation has achieved great success in scene text recognition. Inspired by this, we reversely think of its usage and sophisticatedly take TPS as an exquisite representation for arbitrary shape text representation. The TPS representation is compact, complete, and efficient. With the predicted TPS parameters, the detected text region can be directly rectified to a near-horizontal one to assist the subsequent recognition. To further exploit the potential of the TPS representation, the Border Alignment Loss is proposed. Based on these designs, we implement the text detector TPSNet, which can be extended to a text spotter conveniently. Extensive evaluation and ablation of several public benchmarks demonstrate the effectiveness and superiority of the proposed method for text representation and spotting. Particularly, TPSNet achieves the detection F-Measure improvement of 4.4\% (78.4\% vs. 74.0\%) on Art dataset and the end-to-end spotting F-Measure improvement of 5.0\% (78.5\% vs. 73.5\%) on Total-Text, which are large margins with no bells and whistles.
PDF
点此查看论文截图
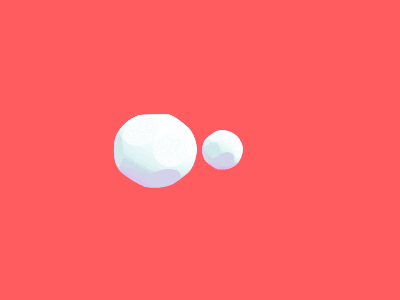
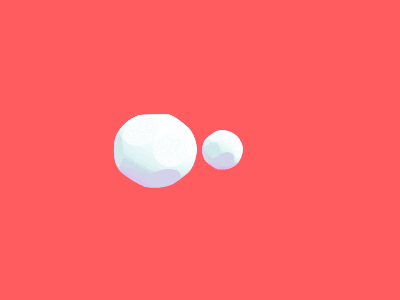
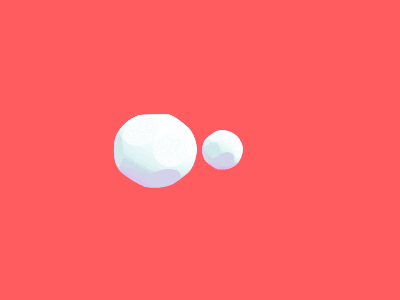
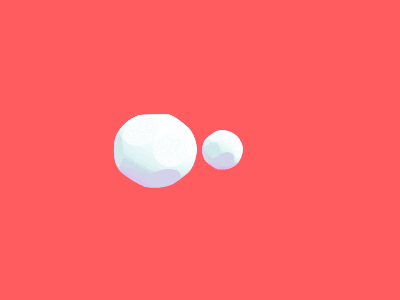
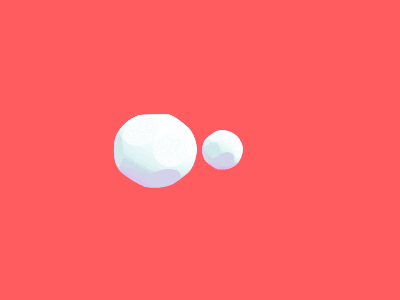
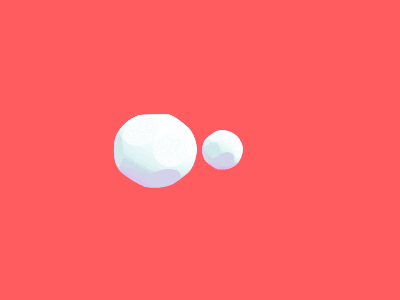
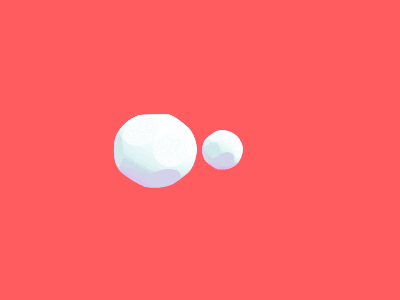
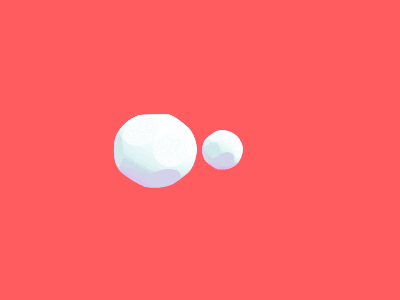