2022-09-05 更新
Object Discovery via Contrastive Learning for Weakly Supervised Object Detection
Authors:Jinhwan Seo, Wonho Bae, Danica J. Sutherland, Junhyug Noh, Daijin Kim
Weakly Supervised Object Detection (WSOD) is a task that detects objects in an image using a model trained only on image-level annotations. Current state-of-the-art models benefit from self-supervised instance-level supervision, but since weak supervision does not include count or location information, the most common ``argmax’’ labeling method often ignores many instances of objects. To alleviate this issue, we propose a novel multiple instance labeling method called object discovery. We further introduce a new contrastive loss under weak supervision where no instance-level information is available for sampling, called weakly supervised contrastive loss (WSCL). WSCL aims to construct a credible similarity threshold for object discovery by leveraging consistent features for embedding vectors in the same class. As a result, we achieve new state-of-the-art results on MS-COCO 2014 and 2017 as well as PASCAL VOC 2012, and competitive results on PASCAL VOC 2007.
PDF Accepted at ECCV 2022. For project page, see https://jinhseo.github.io/research/wsod.html For code, see https://github.com/jinhseo/OD-WSCL
点此查看论文截图
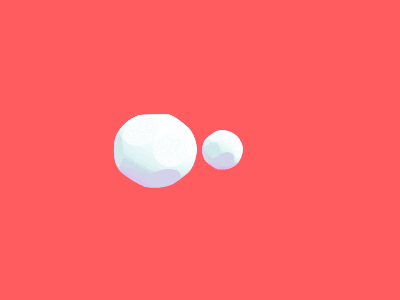
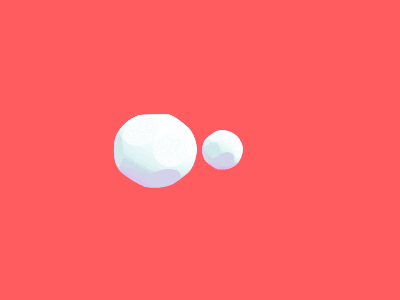
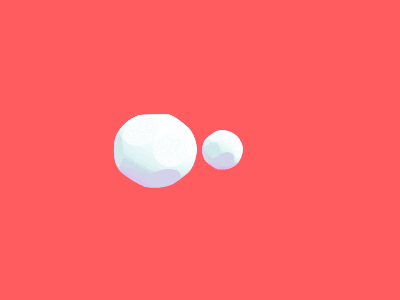
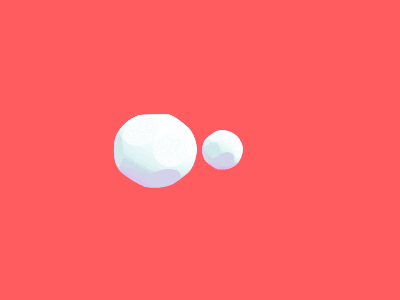