2022-09-05 更新
Unsupervised Joint Image Transfer and Uncertainty Quantification Using Patch Invariant Networks
Authors:Christoph Angermann, Markus Haltmeier, Ahsan Raza Siyal
Unsupervised image transfer enables intra- and inter-modality image translation in applications where a large amount of paired training data is not abundant. To ensure a structure-preserving mapping from the input to the target domain, existing methods for unpaired image transfer are commonly based on cycle-consistency, causing additional computational resources and instability due to the learning of an inverse mapping. This paper presents a novel method for uni-directional domain mapping that does not rely on any paired training data. A proper transfer is achieved by using a GAN architecture and a novel generator loss based on patch invariance. To be more specific, the generator outputs are evaluated and compared at different scales, also leading to an increased focus on high-frequency details as well as an implicit data augmentation. This novel patch loss also offers the possibility to accurately predict aleatoric uncertainty by modeling an input-dependent scale map for the patch residuals. The proposed method is comprehensively evaluated on three well-established medical databases. As compared to four state-of-the-art methods, we observe significantly higher accuracy on these datasets, indicating great potential of the proposed method for unpaired image transfer with uncertainty taken into account. Implementation of the proposed framework is released here: \url{https://github.com/anger-man/unsupervised-image-transfer-and-uq}.
PDF Accepted to ECCV 2022 Workshop on Uncertainty Quantification for Computer Vision (UNCV 2022)
点此查看论文截图
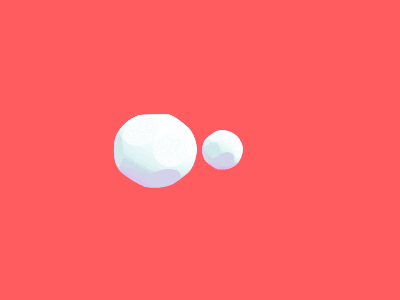
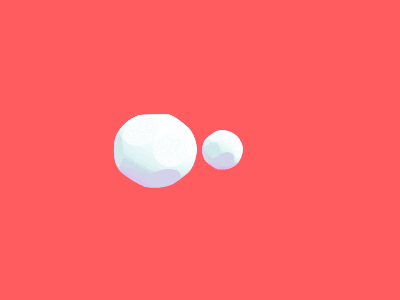
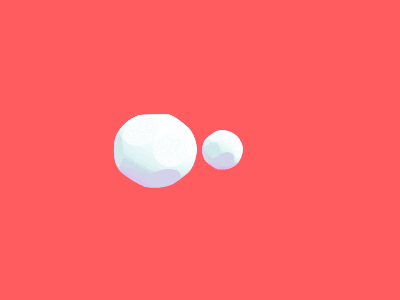
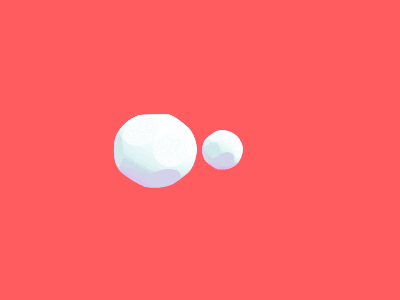
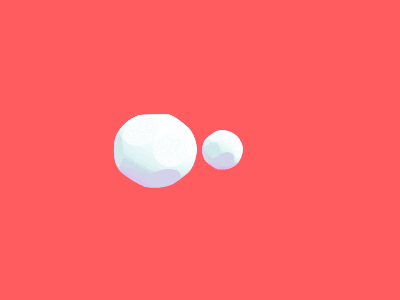