2022-08-27 更新
Dual Diffusion Implicit Bridges for Image-to-Image Translation
Authors:Xuan Su, Jiaming Song, Chenlin Meng, Stefano Ermon
Common image-to-image translation methods rely on joint training over data from both source and target domains. This prevents the training process from preserving privacy of domain data (e.g., in a federated setting), and often means that a new model has to be trained for a new pair of domains. We present Dual Diffusion Implicit Bridges (DDIBs), an image translation method based on diffusion models, that circumvents training on domain pairs. Image translation with DDIBs relies on two diffusion models trained independently on each domain, and is a two-step process: DDIBs first obtain latent encodings for source images with the source diffusion model, and then decode such encodings using the target model to construct target images. Both steps are defined via an ODE, thus the process is cycle consistent only up to discretization errors of the ODE solvers. Theoretically, we interpret DDIBs as concatenation of source to latent, and latent to target Schr\”odinger Bridges, a form of entropy-regularized optimal transport, to explain the efficacy of the method. Experimentally, we apply DDIBs on both synthetic and high-resolution image datasets, to demonstrate their utility in a wide variety of translation tasks and their connections to existing optimal transport methods.
PDF 16 pages, 12 figures, in review
点此查看论文截图
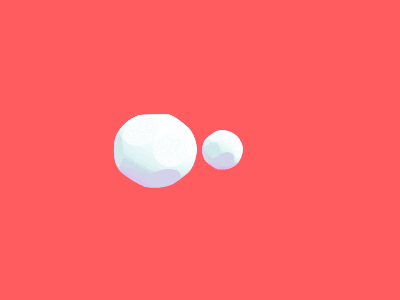
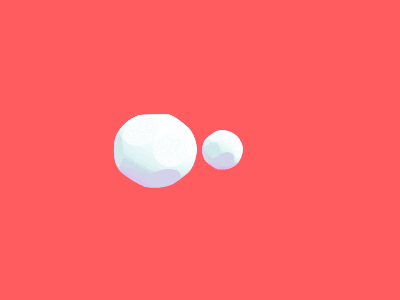
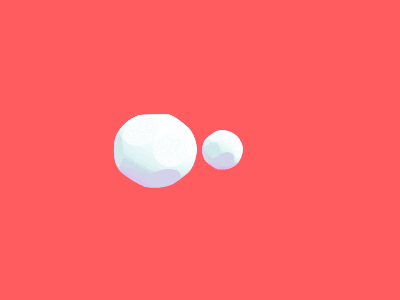
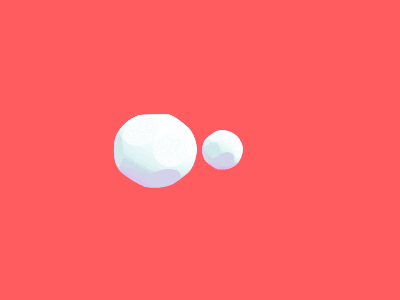
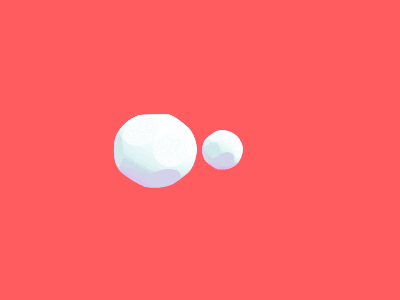
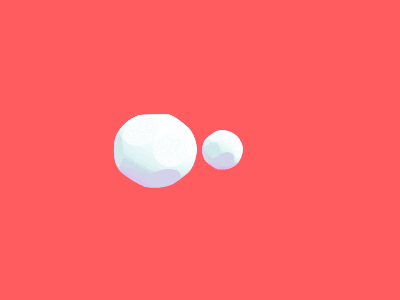
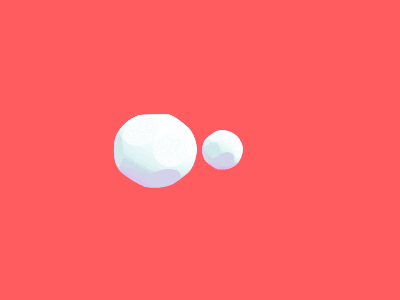
Generative Adversarial Network (GAN) based Image-Deblurring
Authors:Yuhong Lu, Nicholas Polydorides
This thesis analyzes the challenging problem of Image Deblurring based on classical theorems and state-of-art methods proposed in recent years. By spectral analysis we mathematically show the effective of spectral regularization methods, and point out the linking between the spectral filtering result and the solution of the regularization optimization objective. For ill-posed problems like image deblurring, the optimization objective contains a regularization term (also called the regularization functional) that encodes our prior knowledge into the solution. We demonstrate how to craft a regularization term by hand using the idea of maximum a posterior estimation. Then, we point out the limitations of such regularization-based methods, and step into the neural-network based methods. Based on the idea of Wasserstein generative adversarial models, we can train a CNN to learn the regularization functional. Such data-driven approaches are able to capture the complexity, which may not be analytically modellable. Besides, in recent years with the improvement of architectures, the network has been able to output an image closely approximating the ground truth given the blurry observation. The Generative Adversarial Network (GAN) works on this Image-to-Image translation idea. We analyze the DeblurGAN-v2 method proposed by Orest Kupyn et al. [14] in 2019 based on numerical tests. And, based on the experimental results and our knowledge, we put forward some suggestions for improvement on this method.
PDF 90 pages, 35 figures, MS Thesis at the University of Edinburgh
点此查看论文截图
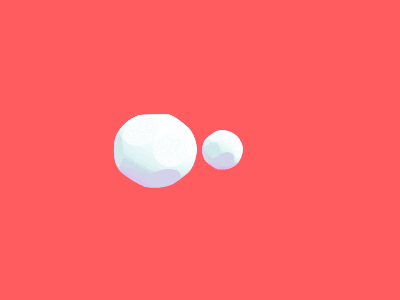
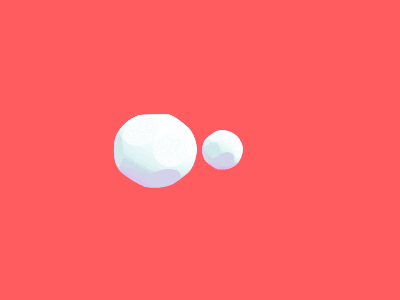
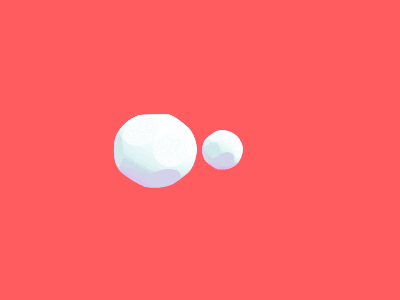