2022-06-28 更新
Woodscape Fisheye Object Detection for Autonomous Driving — CVPR 2022 OmniCV Workshop Challenge
Authors:Saravanabalagi Ramachandran, Ganesh Sistu, Varun Ravi Kumar, John McDonald, Senthil Yogamani
Object detection is a comprehensively studied problem in autonomous driving. However, it has been relatively less explored in the case of fisheye cameras. The strong radial distortion breaks the translation invariance inductive bias of Convolutional Neural Networks. Thus, we present the WoodScape fisheye object detection challenge for autonomous driving which was held as part of the CVPR 2022 Workshop on Omnidirectional Computer Vision (OmniCV). This is one of the first competitions focused on fisheye camera object detection. We encouraged the participants to design models which work natively on fisheye images without rectification. We used CodaLab to host the competition based on the publicly available WoodScape fisheye dataset. In this paper, we provide a detailed analysis on the competition which attracted the participation of 120 global teams and a total of 1492 submissions. We briefly discuss the details of the winning methods and analyze their qualitative and quantitative results.
PDF Workshop on Omnidirectional Computer Vision (OmniCV) at Conference on Computer Vision and Pattern Recognition (CVPR) 2021
点此查看论文截图
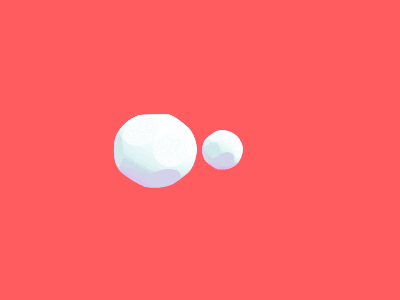
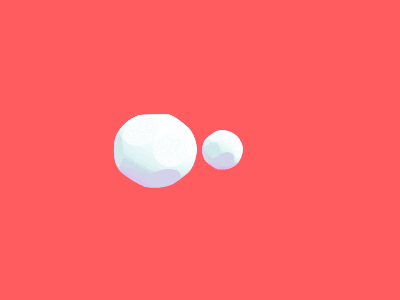
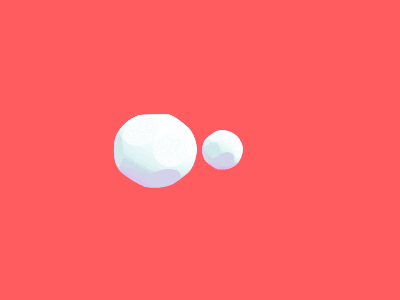
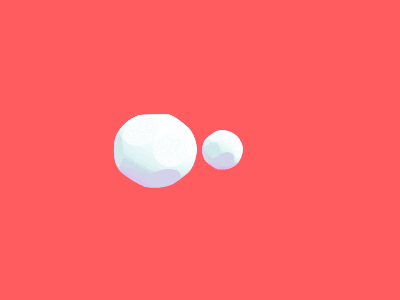
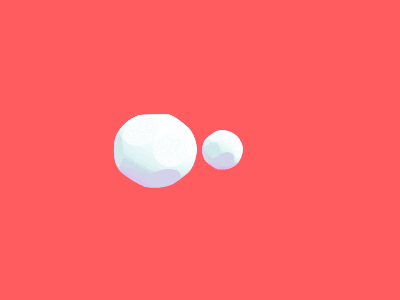
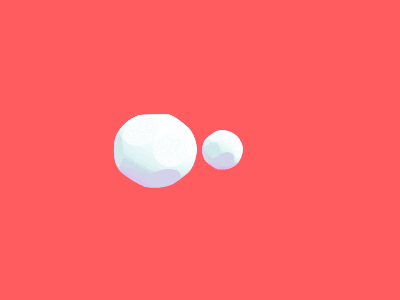
A Strategy Optimized Pix2pix Approach for SAR-to-Optical Image Translation Task
Authors:Fujian Cheng, Yashu Kang, Chunlei Chen, Kezhao Jiang
This paper presented a state-of-the-art framework, Time Gated Convolutional Neural Network (TGCNN) that takes advantage of temporal information and gating mechanisms for the crop classification problem. Besides, several vegetation indices were constructed to expand dimensions of input data to take advantage of spectral information. Both spatial (channel-wise) and temporal (step-wise) correlation are considered in TGCNN. Specifically, our preliminary analysis indicates that step-wise information is of greater importance in this data set. Lastly, the gating mechanism helps capture high-order relationship. Our TGCNN solution achieves $0.973$ F1 score, $0.977$ AUC ROC and $0.948$ IoU, respectively. In addition, it outperforms three other benchmarks in different local tasks (Kenya, Brazil and Togo). Overall, our experiments demonstrate that TGCNN is advantageous in this earth observation time series classification task.
PDF
点此查看论文截图
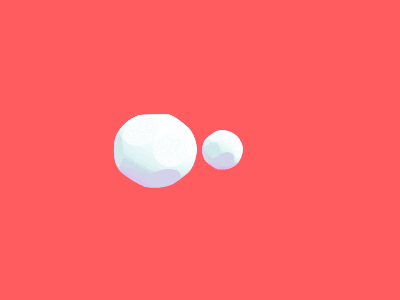
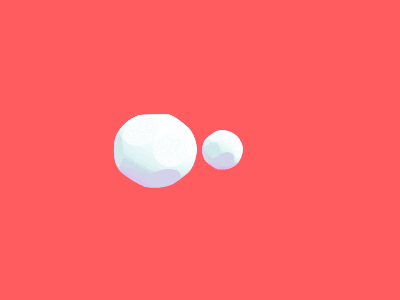
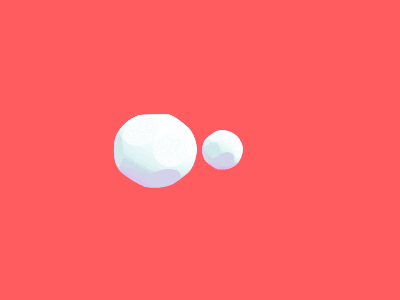
Neural Global Shutter: Learn to Restore Video from a Rolling Shutter Camera with Global Reset Feature
Authors:Zhixiang Wang, Xiang Ji, Jia-Bin Huang, Shin’ichi Satoh, Xiao Zhou, Yinqiang Zheng
Most computer vision systems assume distortion-free images as inputs. The widely used rolling-shutter (RS) image sensors, however, suffer from geometric distortion when the camera and object undergo motion during capture. Extensive researches have been conducted on correcting RS distortions. However, most of the existing work relies heavily on the prior assumptions of scenes or motions. Besides, the motion estimation steps are either oversimplified or computationally inefficient due to the heavy flow warping, limiting their applicability. In this paper, we investigate using rolling shutter with a global reset feature (RSGR) to restore clean global shutter (GS) videos. This feature enables us to turn the rectification problem into a deblur-like one, getting rid of inaccurate and costly explicit motion estimation. First, we build an optic system that captures paired RSGR/GS videos. Second, we develop a novel algorithm incorporating spatial and temporal designs to correct the spatial-varying RSGR distortion. Third, we demonstrate that existing image-to-image translation algorithms can recover clean GS videos from distorted RSGR inputs, yet our algorithm achieves the best performance with the specific designs. Our rendered results are not only visually appealing but also beneficial to downstream tasks. Compared to the state-of-the-art RS solution, our RSGR solution is superior in both effectiveness and efficiency. Considering it is easy to realize without changing the hardware, we believe our RSGR solution can potentially replace the RS solution in taking distortion-free videos with low noise and low budget.
PDF CVPR2022, https://github.com/lightChaserX/neural-global-shutter
点此查看论文截图
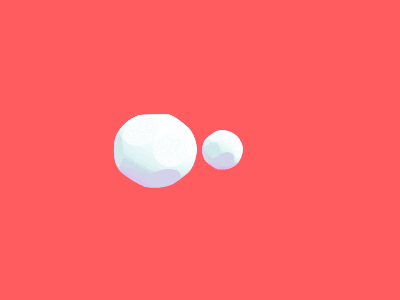
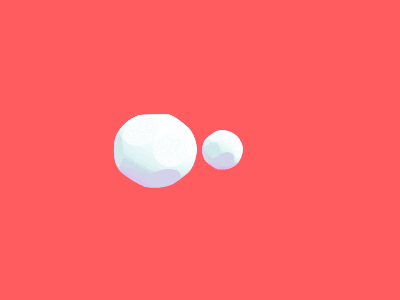
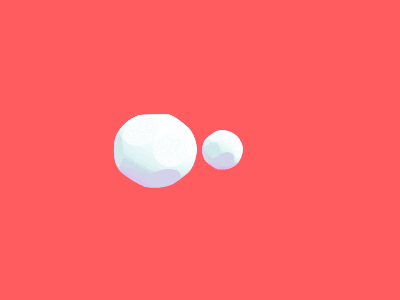
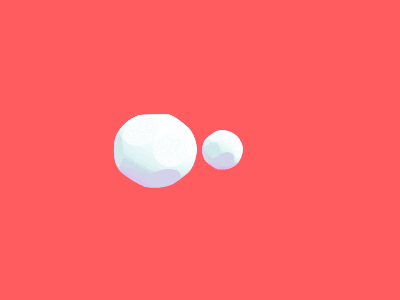
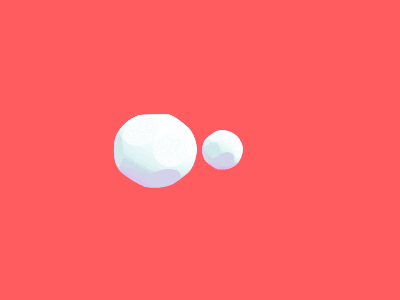
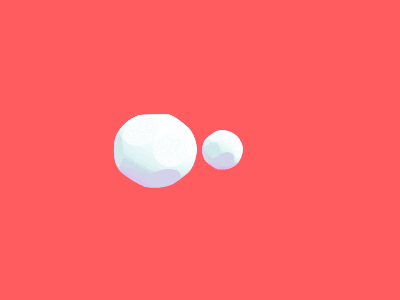
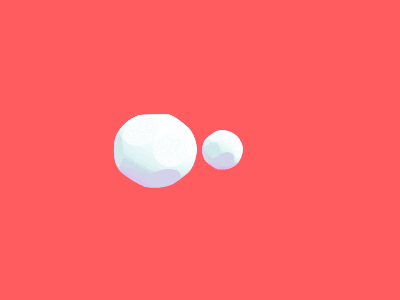
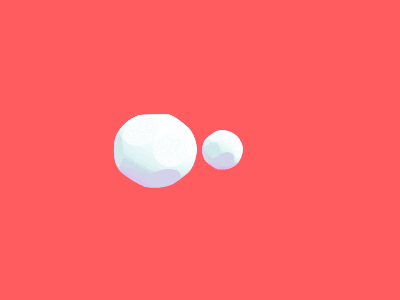
2022-06-28 更新
Test-time image-to-image translation ensembling improves out-of-distribution generalization in histopathology
Authors:Marin Scalbert, Maria Vakalopoulou, Florent Couzinié-Devy
Histopathology whole slide images (WSIs) can reveal significant inter-hospital variability such as illumination, color or optical artifacts. These variations, caused by the use of different scanning protocols across medical centers (staining, scanner), can strongly harm algorithms generalization on unseen protocols. This motivates development of new methods to limit such drop of performances. In this paper, to enhance robustness on unseen target protocols, we propose a new test-time data augmentation based on multi domain image-to-image translation. It allows to project images from unseen protocol into each source domain before classifying them and ensembling the predictions. This test-time augmentation method results in a significant boost of performances for domain generalization. To demonstrate its effectiveness, our method has been evaluated on 2 different histopathology tasks where it outperforms conventional domain generalization, standard H&E specific color augmentation/normalization and standard test-time augmentation techniques. Our code is publicly available at https://gitlab.com/vitadx/articles/test-time-i2i-translation-ensembling.
PDF Accepted on MICCAI2022 Conference
点此查看论文截图
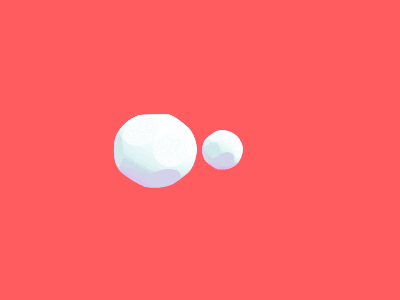
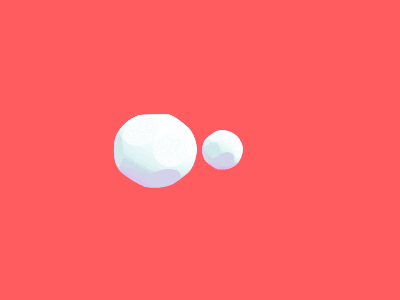
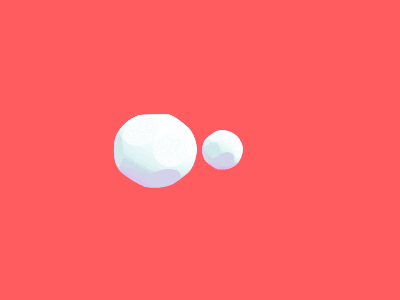
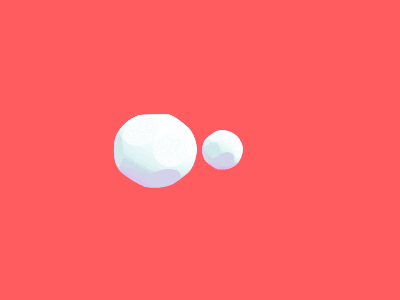
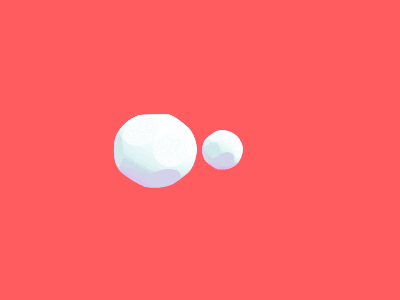