2022-06-25 更新
What makes you, you? Analyzing Recognition by Swapping Face Parts
Authors:Claudio Ferrari, Matteo Serpentoni, Stefano Berretti, Alberto Del Bimbo
Deep learning advanced face recognition to an unprecedented accuracy. However, understanding how local parts of the face affect the overall recognition performance is still mostly unclear. Among others, face swap has been experimented to this end, but just for the entire face. In this paper, we propose to swap facial parts as a way to disentangle the recognition relevance of different face parts, like eyes, nose and mouth. In our method, swapping parts from a source face to a target one is performed by fitting a 3D prior, which establishes dense pixels correspondence between parts, while also handling pose differences. Seamless cloning is then used to obtain smooth transitions between the mapped source regions and the shape and skin tone of the target face. We devised an experimental protocol that allowed us to draw some preliminary conclusions when the swapped images are classified by deep networks, indicating a prominence of the eyes and eyebrows region. Code available at https://github.com/clferrari/FacePartsSwap
PDF Accepted for publication at 26TH International Conference on Pattern Recognition (ICPR), 2022
论文截图
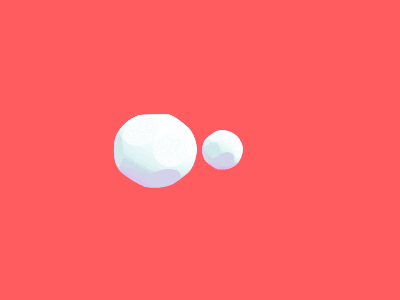
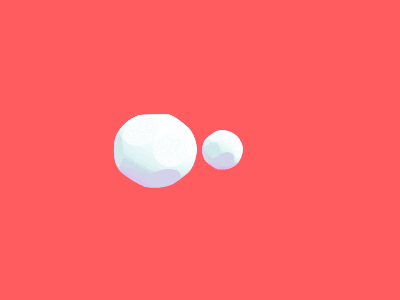
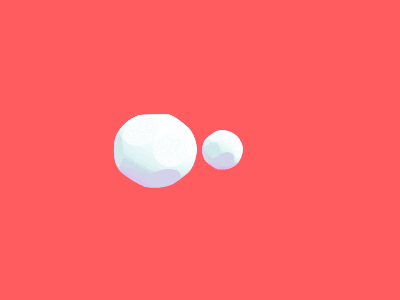
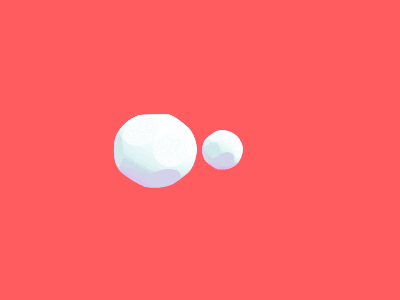
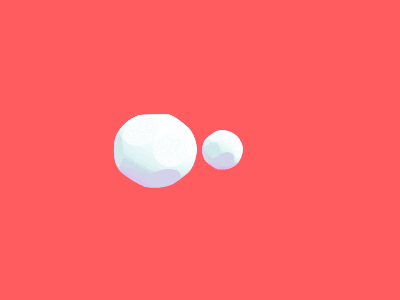
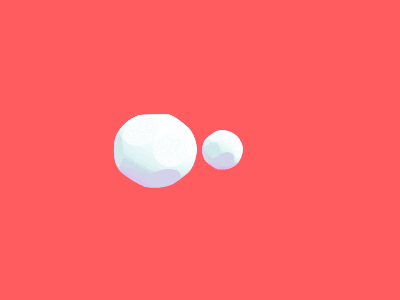
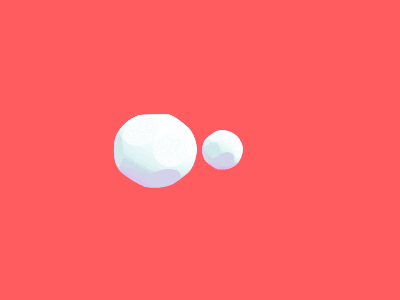
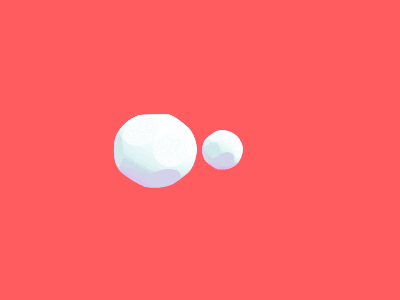
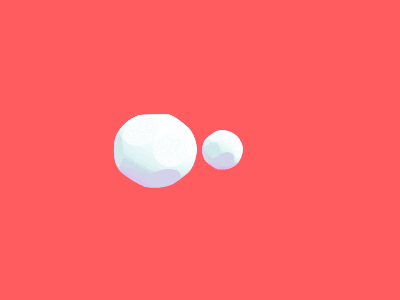
SFace: Privacy-friendly and Accurate Face Recognition using Synthetic Data
Authors:Fadi Boutros, Marco Huber, Patrick Siebke, Tim Rieber, Naser Damer
Recent deep face recognition models proposed in the literature utilized large-scale public datasets such as MS-Celeb-1M and VGGFace2 for training very deep neural networks, achieving state-of-the-art performance on mainstream benchmarks. Recently, many of these datasets, e.g., MS-Celeb-1M and VGGFace2, are retracted due to credible privacy and ethical concerns. This motivates this work to propose and investigate the feasibility of using a privacy-friendly synthetically generated face dataset to train face recognition models. Towards this end, we utilize a class-conditional generative adversarial network to generate class-labeled synthetic face images, namely SFace. To address the privacy aspect of using such data to train a face recognition model, we provide extensive evaluation experiments on the identity relation between the synthetic dataset and the original authentic dataset used to train the generative model. Our reported evaluation proved that associating an identity of the authentic dataset to one with the same class label in the synthetic dataset is hardly possible. We also propose to train face recognition on our privacy-friendly dataset, SFace, using three different learning strategies, multi-class classification, label-free knowledge transfer, and combined learning of multi-class classification and knowledge transfer. The reported evaluation results on five authentic face benchmarks demonstrated that the privacy-friendly synthetic dataset has high potential to be used for training face recognition models, achieving, for example, a verification accuracy of 91.87\% on LFW using multi-class classification and 99.13\% using the combined learning strategy.
PDF
论文截图
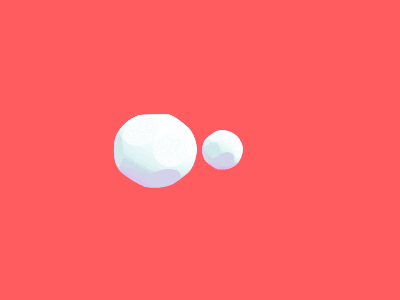
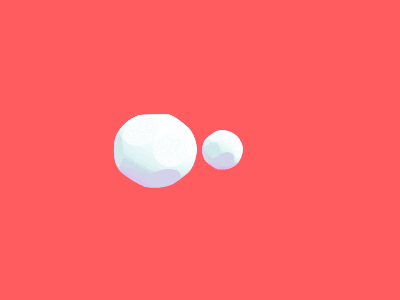
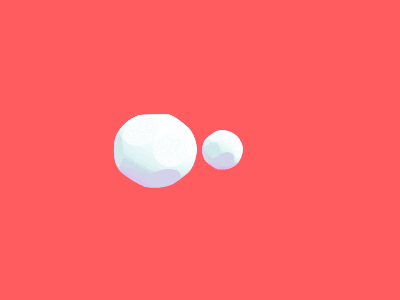
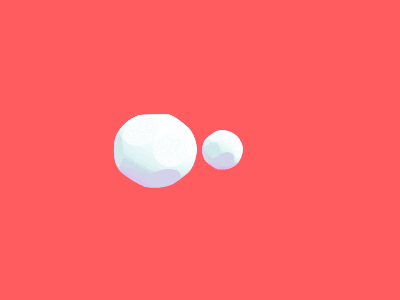
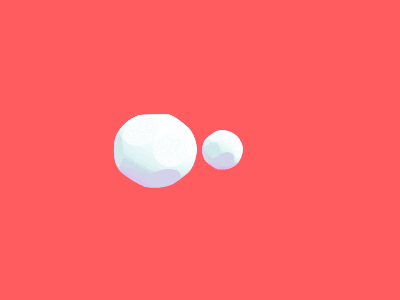
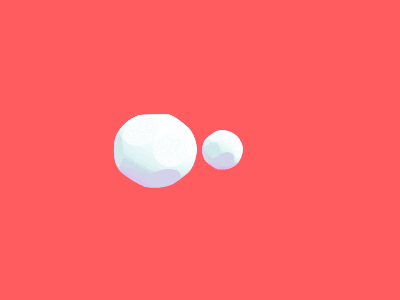
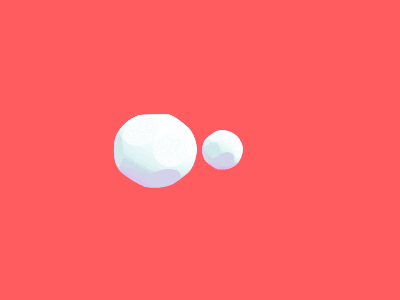
3D Face Parsing via Surface Parameterization and 2D Semantic Segmentation Network
Authors:Wenyuan Sun, Ping Zhou, Yangang Wang, Zongpu Yu, Jing Jin, Guangquan Zhou
Face parsing assigns pixel-wise semantic labels as the face representation for computers, which is the fundamental part of many advanced face technologies. Compared with 2D face parsing, 3D face parsing shows more potential to achieve better performance and further application, but it is still challenging due to 3D mesh data computation. Recent works introduced different methods for 3D surface segmentation, while the performance is still limited. In this paper, we propose a method based on the “3D-2D-3D” strategy to accomplish 3D face parsing. The topological disk-like 2D face image containing spatial and textural information is transformed from the sampled 3D face data through the face parameterization algorithm, and a specific 2D network called CPFNet is proposed to achieve the semantic segmentation of the 2D parameterized face data with multi-scale technologies and feature aggregation. The 2D semantic result is then inversely re-mapped to 3D face data, which finally achieves the 3D face parsing. Experimental results show that both CPFNet and the “3D-2D-3D” strategy accomplish high-quality 3D face parsing and outperform state-of-the-art 2D networks as well as 3D methods in both qualitative and quantitative comparisons.
PDF
论文截图
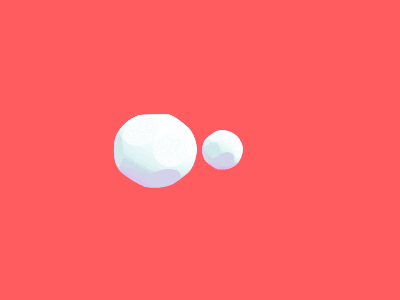
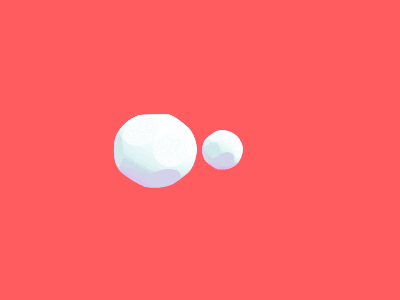
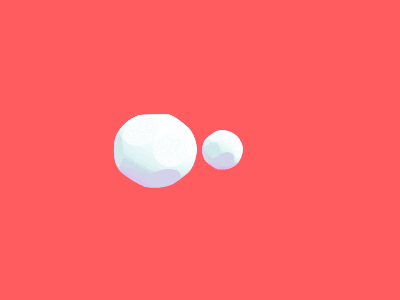
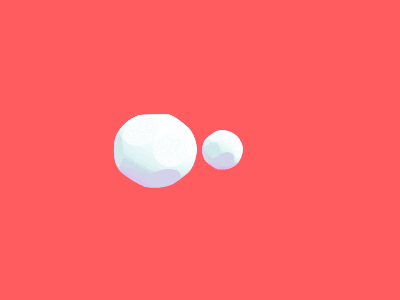
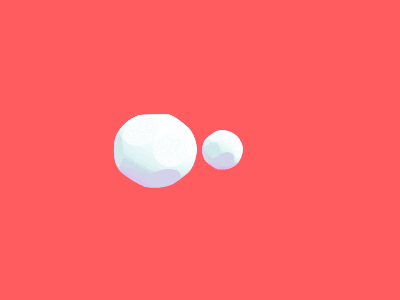
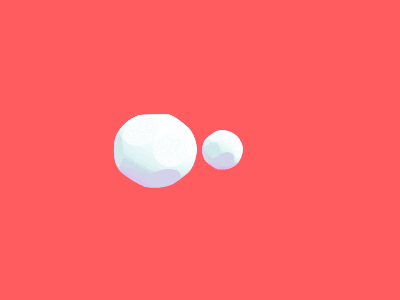
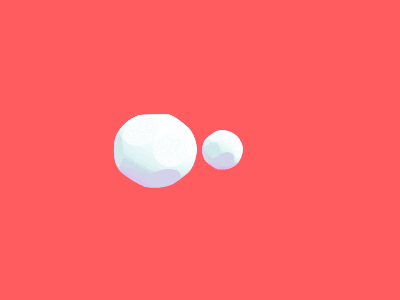
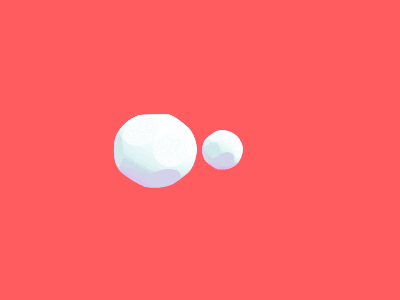
A Novel Face-Anti Spoofing Neural Network Model For Face Recognition And Detection
Authors:Soham S. Sarpotdar
Face Recognition (FR) systems are being used in a variety of applications, including road crossings, banking, and mobile banking. The widespread use of FR systems has raised concerns about the safety of face biometrics against spoofing attacks, which use the use of a photo or video of a legitimate user’s face to gain illegal access to the resources or activities. Despite the development of several FAS or liveness detection methods (which determine whether a face is live or spoofed at the time of acquisition), the problem remains unsolved due to the difficulty of identifying discrimination and operationally reasonably priced spoof characteristics but also approaches. Additionally, certain facial portions are frequently repeated or correlate to image clutter, resulting in poor performance overall. This research proposes a face-anti-spoofing neural network model that outperforms existing models and has an efficiency of 0.89 percent.
PDF 9 Pages
论文截图
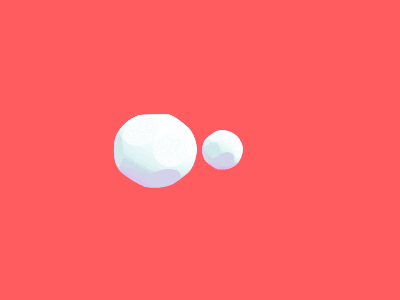
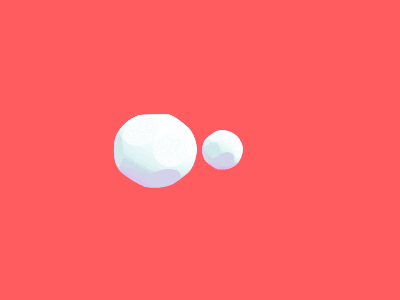
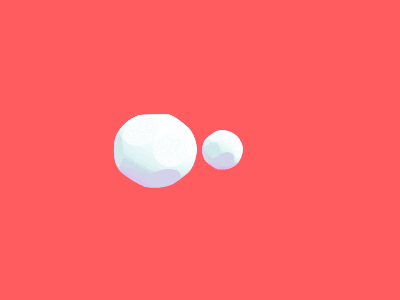
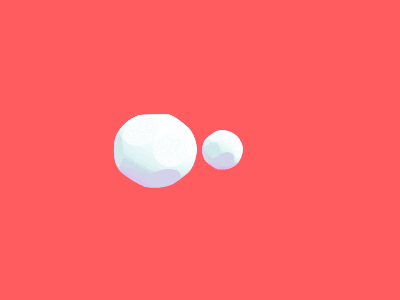
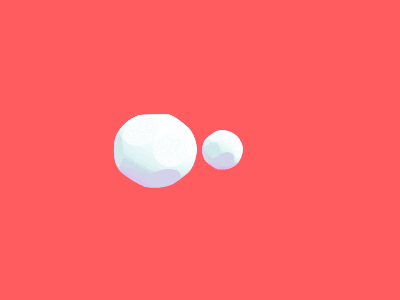
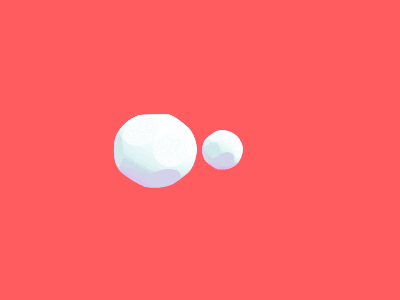