2022-06-25 更新
Making Generated Images Hard To Spot: A Transferable Attack On Synthetic Image Detectors
Authors:Xinwei Zhao, Matthew C. Stamm
Visually realistic GAN-generated images have recently emerged as an important misinformation threat. Research has shown that these synthetic images contain forensic traces that are readily identifiable by forensic detectors. Unfortunately, these detectors are built upon neural networks, which are vulnerable to recently developed adversarial attacks. In this paper, we propose a new anti-forensic attack capable of fooling GAN-generated image detectors. Our attack uses an adversarially trained generator to synthesize traces that these detectors associate with real images. Furthermore, we propose a technique to train our attack so that it can achieve transferability, i.e. it can fool unknown CNNs that it was not explicitly trained against. We evaluate our attack through an extensive set of experiments, where we show that our attack can fool eight state-of-the-art detection CNNs with synthetic images created using seven different GANs, and outperform other alternative attacks.
PDF
论文截图
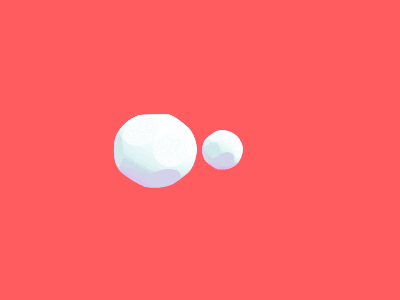
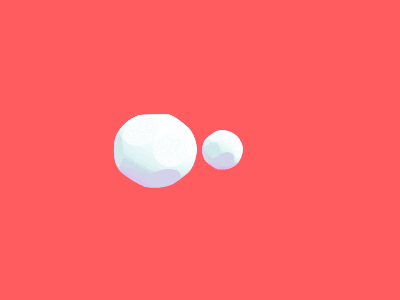
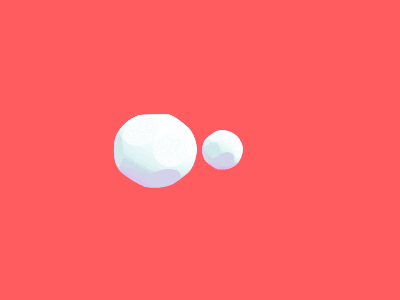
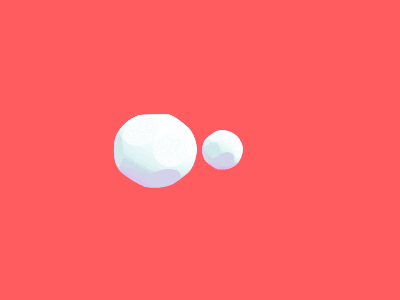
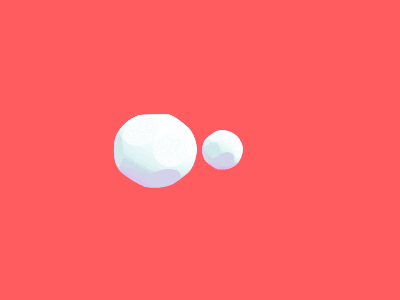
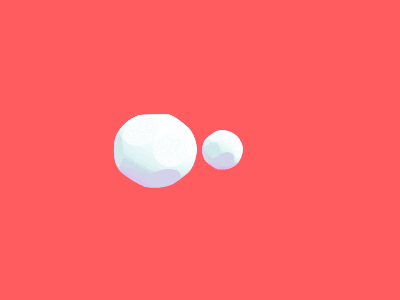
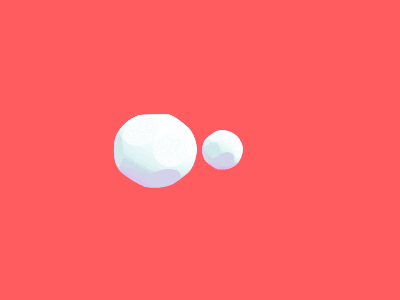
Person Re-identification Method Based on Color Attack and Joint Defence
Authors:Yunpeng Gong, Liqing Huang, Lifei Chen
The main challenges of ReID is the intra-class variations caused by color deviation under different camera conditions. Simultaneously, we find that most of the existing adversarial metric attacks are realized by interfering with the color characteristics of the sample. Based on this observation, we first propose a local transformation attack (LTA) based on color variation. It uses more obvious color variation to randomly disturb the color of the retrieved image, rather than adding random noise. Experiments show that the performance of the proposed LTA method is better than the advanced attack methods. Furthermore, considering that the contour feature is the main factor of the robustness of adversarial training, and the color feature will directly affect the success rate of attack. Therefore, we further propose joint adversarial defense (JAD) method, which include proactive defense and passive defense. Proactive defense fuse multi-modality images to enhance the contour feature and color feature, and considers local homomorphic transformation to solve the over-fitting problem. Passive defense exploits the invariance of contour feature during image scaling to mitigate the adversarial disturbance on contour feature. Finally, a series of experimental results show that the proposed joint adversarial defense method is more competitive than a state-of-the-art methods.
PDF Accepted by CVPR2022 Workshops (https://openaccess.thecvf.com/content/CVPR2022W/HCIS/html/Gong_Person_Re-Identification_Method_Based_on_Color_Attack_and_Joint_Defence_CVPRW_2022_paper.html)
论文截图
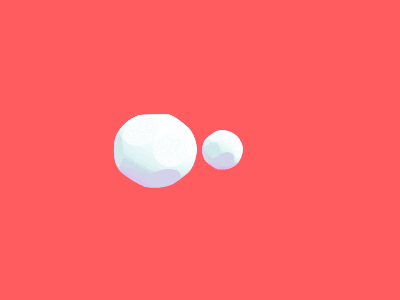
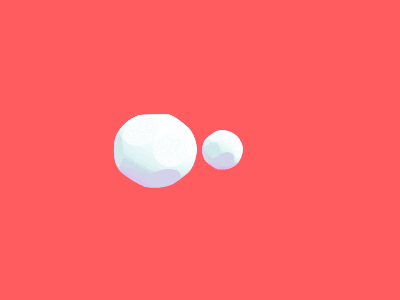
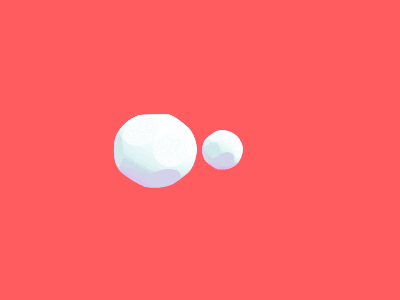
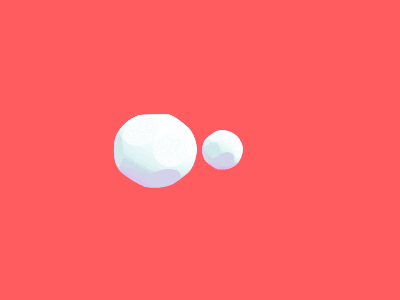
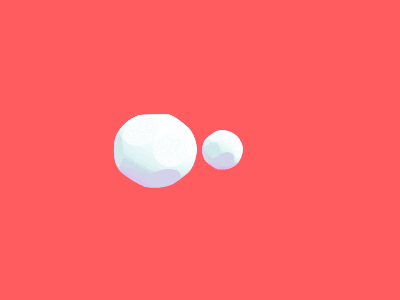
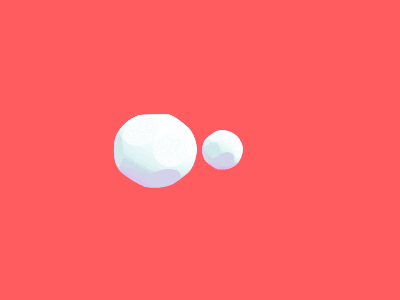
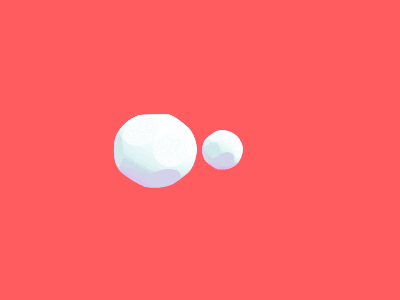
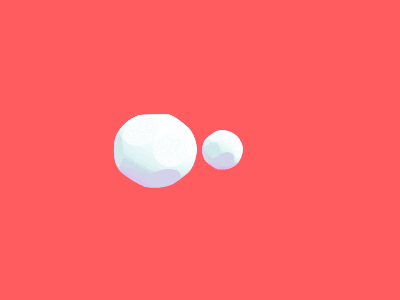
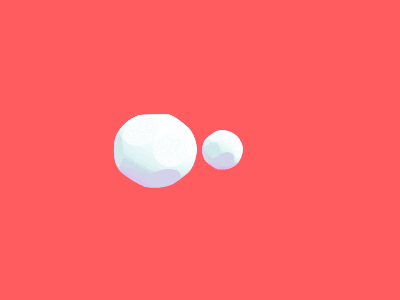