2024-08-27 更新
Enhancing Cross-Modal Medical Image Segmentation through Compositionality
Authors:Aniek Eijpe, Valentina Corbetta, Kalina Chupetlovska, Regina Beets-Tan, Wilson Silva
Cross-modal medical image segmentation presents a significant challenge, as different imaging modalities produce images with varying resolutions, contrasts, and appearances of anatomical structures. We introduce compositionality as an inductive bias in a cross-modal segmentation network to improve segmentation performance and interpretability while reducing complexity. The proposed network is an end-to-end cross-modal segmentation framework that enforces compositionality on the learned representations using learnable von Mises-Fisher kernels. These kernels facilitate content-style disentanglement in the learned representations, resulting in compositional content representations that are inherently interpretable and effectively disentangle different anatomical structures. The experimental results demonstrate enhanced segmentation performance and reduced computational costs on multiple medical datasets. Additionally, we demonstrate the interpretability of the learned compositional features. Code and checkpoints will be publicly available at: https://github.com/Trustworthy-AI-UU-NKI/Cross-Modal-Segmentation.
PDF 11 pages, 3 figures, 2 tables. Accepted at Deep Generative Models workshop @ MICCAI 2024 (DGM4MICCAI). This is the submitted manuscript with added link to github repo, funding acknowledgements and authors’ names and affiliations. No further post submission improvements or corrections were integrated. Final version not published yet
点此查看论文截图
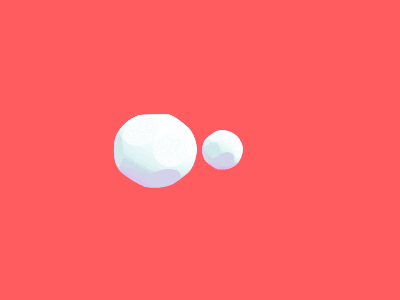
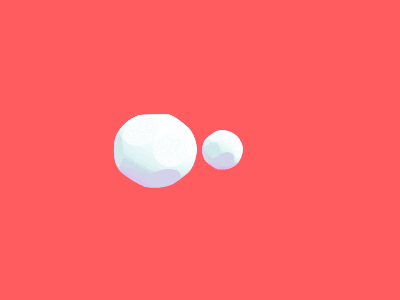
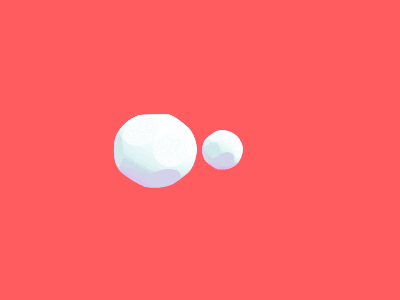
Multi-Style Facial Sketch Synthesis through Masked Generative Modeling
Authors:Bowen Sun, Guo Lu, Shibao Zheng
The facial sketch synthesis (FSS) model, capable of generating sketch portraits from given facial photographs, holds profound implications across multiple domains, encompassing cross-modal face recognition, entertainment, art, media, among others. However, the production of high-quality sketches remains a formidable task, primarily due to the challenges and flaws associated with three key factors: (1) the scarcity of artist-drawn data, (2) the constraints imposed by limited style types, and (3) the deficiencies of processing input information in existing models. To address these difficulties, we propose a lightweight end-to-end synthesis model that efficiently converts images to corresponding multi-stylized sketches, obviating the necessity for any supplementary inputs (\eg, 3D geometry). In this study, we overcome the issue of data insufficiency by incorporating semi-supervised learning into the training process. Additionally, we employ a feature extraction module and style embeddings to proficiently steer the generative transformer during the iterative prediction of masked image tokens, thus achieving a continuous stylized output that retains facial features accurately in sketches. The extensive experiments demonstrate that our method consistently outperforms previous algorithms across multiple benchmarks, exhibiting a discernible disparity.
PDF
点此查看论文截图
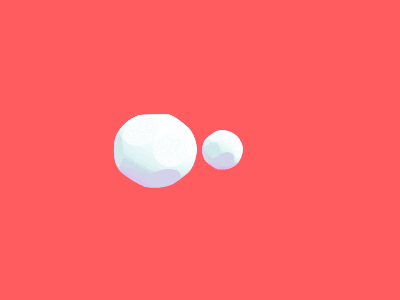
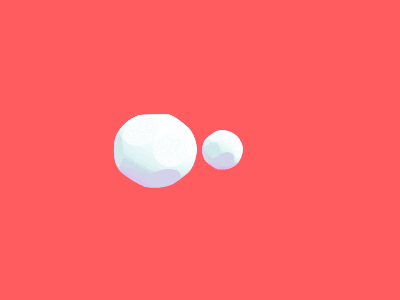
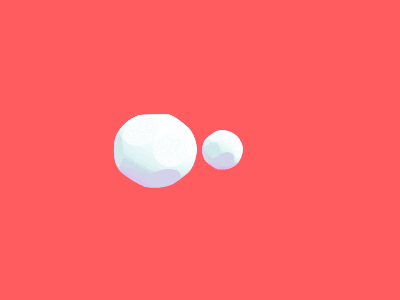
Show-o: One Single Transformer to Unify Multimodal Understanding and Generation
Authors:Jinheng Xie, Weijia Mao, Zechen Bai, David Junhao Zhang, Weihao Wang, Kevin Qinghong Lin, Yuchao Gu, Zhijie Chen, Zhenheng Yang, Mike Zheng Shou
We present a unified transformer, i.e., Show-o, that unifies multimodal understanding and generation. Unlike fully autoregressive models, Show-o unifies autoregressive and (discrete) diffusion modeling to adaptively handle inputs and outputs of various and mixed modalities. The unified model flexibly supports a wide range of vision-language tasks including visual question-answering, text-to-image generation, text-guided inpainting/extrapolation, and mixed-modality generation. Across various benchmarks, it demonstrates comparable or superior performance to existing individual models with an equivalent or larger number of parameters tailored for understanding or generation. This significantly highlights its potential as a next-generation foundation model. Code and models are released at https://github.com/showlab/Show-o.
PDF Technical Report
点此查看论文截图
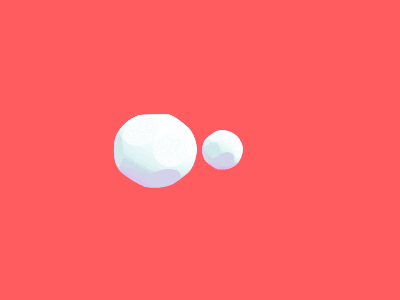
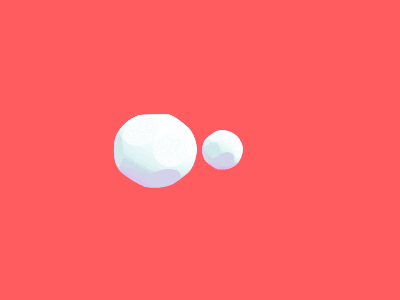
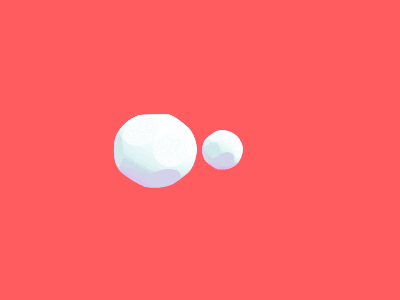
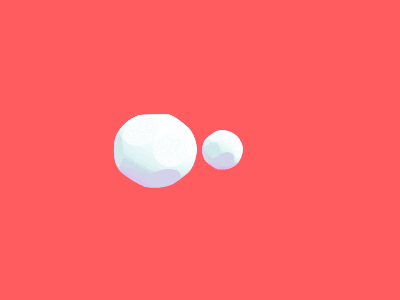
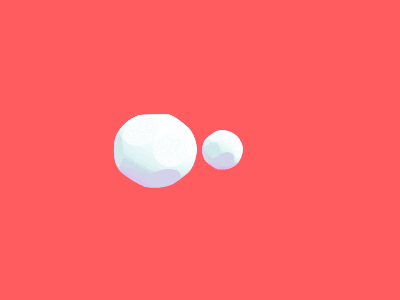
TVG: A Training-free Transition Video Generation Method with Diffusion Models
Authors:Rui Zhang, Yaosen Chen, Yuegen Liu, Wei Wang, Xuming Wen, Hongxia Wang
Transition videos play a crucial role in media production, enhancing the flow and coherence of visual narratives. Traditional methods like morphing often lack artistic appeal and require specialized skills, limiting their effectiveness. Recent advances in diffusion model-based video generation offer new possibilities for creating transitions but face challenges such as poor inter-frame relationship modeling and abrupt content changes. We propose a novel training-free Transition Video Generation (TVG) approach using video-level diffusion models that addresses these limitations without additional training. Our method leverages Gaussian Process Regression ($\mathcal{GPR}$) to model latent representations, ensuring smooth and dynamic transitions between frames. Additionally, we introduce interpolation-based conditional controls and a Frequency-aware Bidirectional Fusion (FBiF) architecture to enhance temporal control and transition reliability. Evaluations of benchmark datasets and custom image pairs demonstrate the effectiveness of our approach in generating high-quality smooth transition videos. The code are provided in https://sobeymil.github.io/tvg.com.
PDF
点此查看论文截图
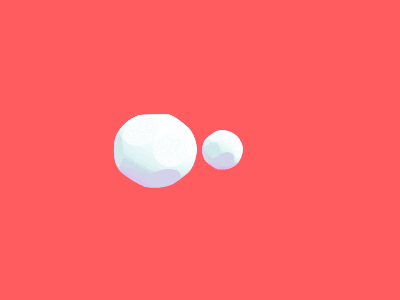
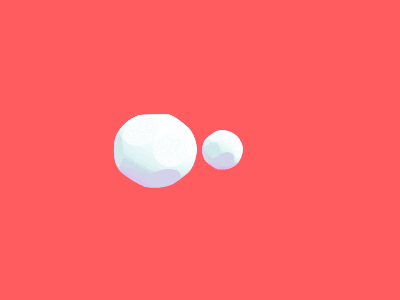
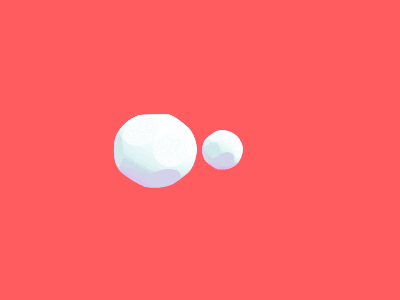
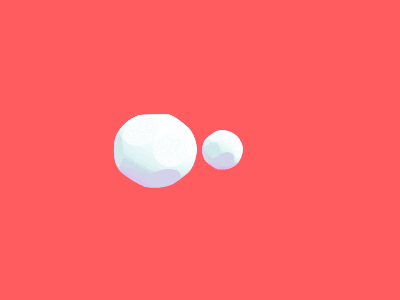
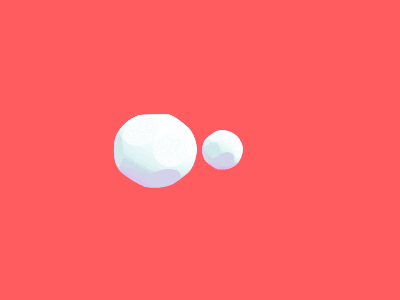
Rethinking Video Deblurring with Wavelet-Aware Dynamic Transformer and Diffusion Model
Authors:Chen Rao, Guangyuan Li, Zehua Lan, Jiakai Sun, Junsheng Luan, Wei Xing, Lei Zhao, Huaizhong Lin, Jianfeng Dong, Dalong Zhang
Current video deblurring methods have limitations in recovering high-frequency information since the regression losses are conservative with high-frequency details. Since Diffusion Models (DMs) have strong capabilities in generating high-frequency details, we consider introducing DMs into the video deblurring task. However, we found that directly applying DMs to the video deblurring task has the following problems: (1) DMs require many iteration steps to generate videos from Gaussian noise, which consumes many computational resources. (2) DMs are easily misled by the blurry artifacts in the video, resulting in irrational content and distortion of the deblurred video. To address the above issues, we propose a novel video deblurring framework VD-Diff that integrates the diffusion model into the Wavelet-Aware Dynamic Transformer (WADT). Specifically, we perform the diffusion model in a highly compact latent space to generate prior features containing high-frequency information that conforms to the ground truth distribution. We design the WADT to preserve and recover the low-frequency information in the video while utilizing the high-frequency information generated by the diffusion model. Extensive experiments show that our proposed VD-Diff outperforms SOTA methods on GoPro, DVD, BSD, and Real-World Video datasets.
PDF accepted by ECCV2024
点此查看论文截图
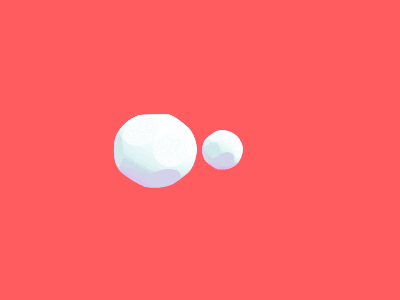
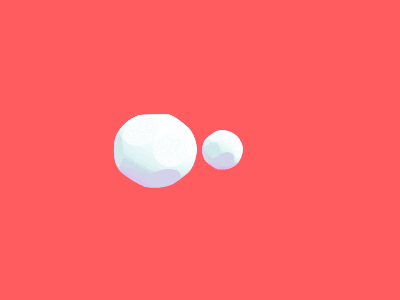
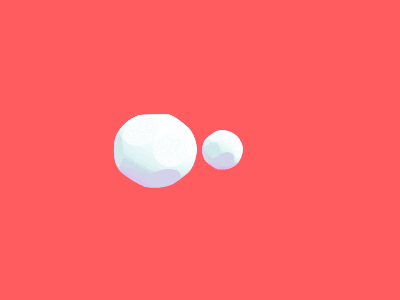
DualAnoDiff: Dual-Interrelated Diffusion Model for Few-Shot Anomaly Image Generation
Authors:Ying Jin, Jinlong Peng, Qingdong He, Teng Hu, Hao Chen, Jiafu Wu, Wenbing Zhu, Mingmin Chi, Jun Liu, Yabiao Wang, Chengjie Wang
The performance of anomaly inspection in industrial manufacturing is constrained by the scarcity of anomaly data. To overcome this challenge, researchers have started employing anomaly generation approaches to augment the anomaly dataset. However, existing anomaly generation methods suffer from limited diversity in the generated anomalies and struggle to achieve a seamless blending of this anomaly with the original image. In this paper, we overcome these challenges from a new perspective, simultaneously generating a pair of the overall image and the corresponding anomaly part. We propose DualAnoDiff, a novel diffusion-based few-shot anomaly image generation model, which can generate diverse and realistic anomaly images by using a dual-interrelated diffusion model, where one of them is employed to generate the whole image while the other one generates the anomaly part. Moreover, we extract background and shape information to mitigate the distortion and blurriness phenomenon in few-shot image generation. Extensive experiments demonstrate the superiority of our proposed model over state-of-the-art methods in terms of both realism and diversity. Overall, our approach significantly improves the performance of downstream anomaly detection tasks, including anomaly detection, anomaly localization, and anomaly classification tasks.
PDF
点此查看论文截图
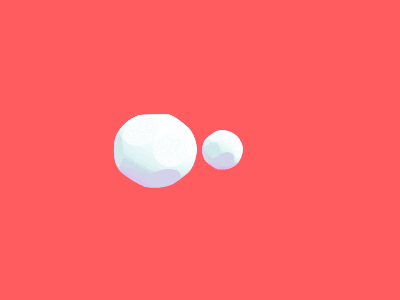
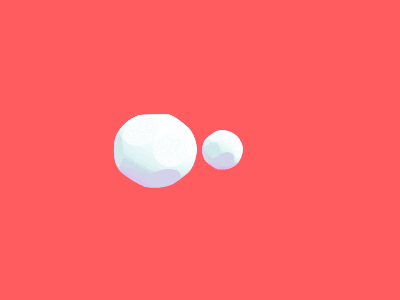
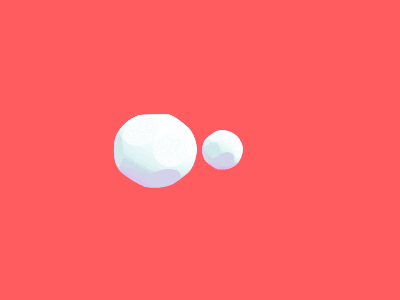
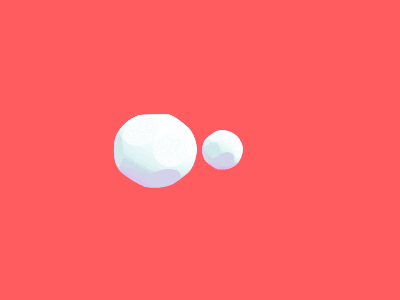
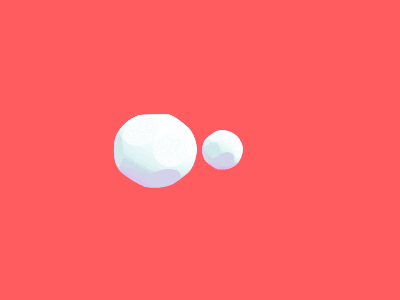
Guided and Fused: Efficient Frozen CLIP-ViT with Feature Guidance and Multi-Stage Feature Fusion for Generalizable Deepfake Detection
Authors:Yingjian Chen, Lei Zhang, Yakun Niu, Pei Chen, Lei Tan, Jing Zhou
The rise of generative models has sparked concerns about image authenticity online, highlighting the urgent need for an effective and general detector. Recent methods leveraging the frozen pre-trained CLIP-ViT model have made great progress in deepfake detection. However, these models often rely on visual-general features directly extracted by the frozen network, which contain excessive information irrelevant to the task, resulting in limited detection performance. To address this limitation, in this paper, we propose an efficient Guided and Fused Frozen CLIP-ViT (GFF), which integrates two simple yet effective modules. The Deepfake-Specific Feature Guidance Module (DFGM) guides the frozen pre-trained model in extracting features specifically for deepfake detection, reducing irrelevant information while preserving its generalization capabilities. The Multi-Stage Fusion Module (FuseFormer) captures low-level and high-level information by fusing features extracted from each stage of the ViT. This dual-module approach significantly improves deepfake detection by fully leveraging CLIP-ViT’s inherent advantages. Extensive experiments demonstrate the effectiveness and generalization ability of GFF, which achieves state-of-the-art performance with optimal results in only 5 training epochs. Even when trained on only 4 classes of ProGAN, GFF achieves nearly 99% accuracy on unseen GANs and maintains an impressive 97% accuracy on unseen diffusion models.
PDF
点此查看论文截图
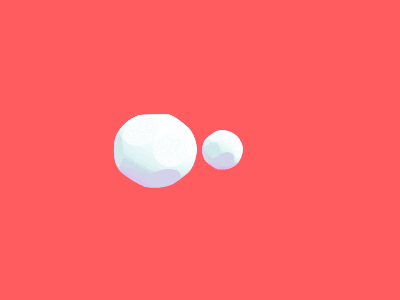
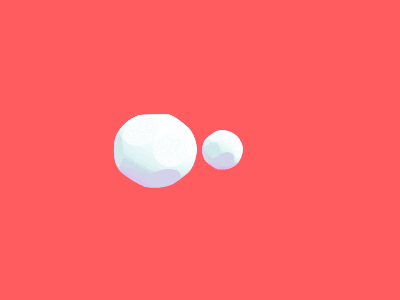
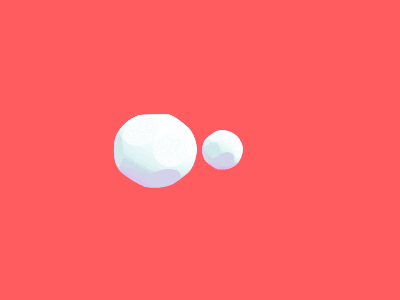
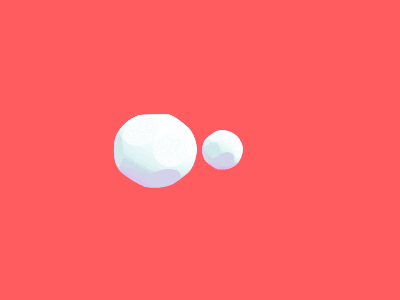
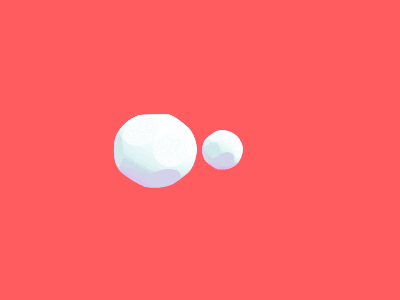
SceneDreamer360: Text-Driven 3D-Consistent Scene Generation with Panoramic Gaussian Splatting
Authors:Wenrui Li, Yapeng Mi, Fucheng Cai, Zhe Yang, Wangmeng Zuo, Xingtao Wang, Xiaopeng Fan
Text-driven 3D scene generation has seen significant advancements recently. However, most existing methods generate single-view images using generative models and then stitch them together in 3D space. This independent generation for each view often results in spatial inconsistency and implausibility in the 3D scenes. To address this challenge, we proposed a novel text-driven 3D-consistent scene generation model: SceneDreamer360. Our proposed method leverages a text-driven panoramic image generation model as a prior for 3D scene generation and employs 3D Gaussian Splatting (3DGS) to ensure consistency across multi-view panoramic images. Specifically, SceneDreamer360 enhances the fine-tuned Panfusion generator with a three-stage panoramic enhancement, enabling the generation of high-resolution, detail-rich panoramic images. During the 3D scene construction, a novel point cloud fusion initialization method is used, producing higher quality and spatially consistent point clouds. Our extensive experiments demonstrate that compared to other methods, SceneDreamer360 with its panoramic image generation and 3DGS can produce higher quality, spatially consistent, and visually appealing 3D scenes from any text prompt. Our codes are available at \url{https://github.com/liwrui/SceneDreamer360}.
PDF
点此查看论文截图
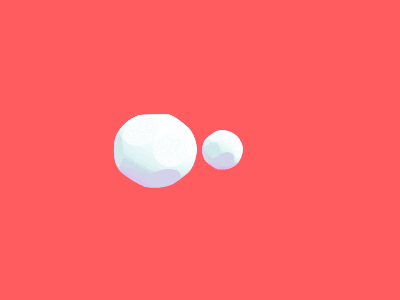
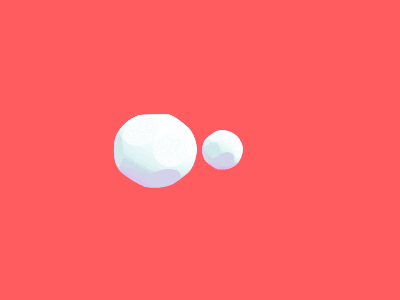
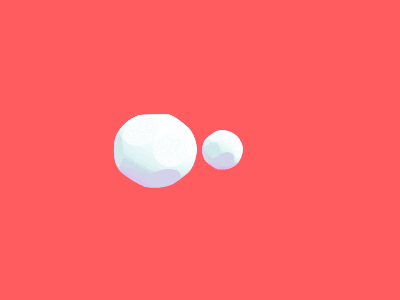
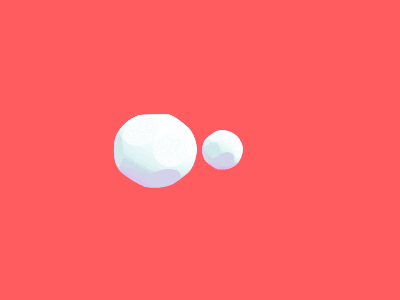
Draw Like an Artist: Complex Scene Generation with Diffusion Model via Composition, Painting, and Retouching
Authors:Minghao Liu, Le Zhang, Yingjie Tian, Xiaochao Qu, Luoqi Liu, Ting Liu
Recent advances in text-to-image diffusion models have demonstrated impressive capabilities in image quality. However, complex scene generation remains relatively unexplored, and even the definition of `complex scene’ itself remains unclear. In this paper, we address this gap by providing a precise definition of complex scenes and introducing a set of Complex Decomposition Criteria (CDC) based on this definition. Inspired by the artists painting process, we propose a training-free diffusion framework called Complex Diffusion (CxD), which divides the process into three stages: composition, painting, and retouching. Our method leverages the powerful chain-of-thought capabilities of large language models (LLMs) to decompose complex prompts based on CDC and to manage composition and layout. We then develop an attention modulation method that guides simple prompts to specific regions to complete the complex scene painting. Finally, we inject the detailed output of the LLM into a retouching model to enhance the image details, thus implementing the retouching stage. Extensive experiments demonstrate that our method outperforms previous SOTA approaches, significantly improving the generation of high-quality, semantically consistent, and visually diverse images for complex scenes, even with intricate prompts.
PDF
点此查看论文截图
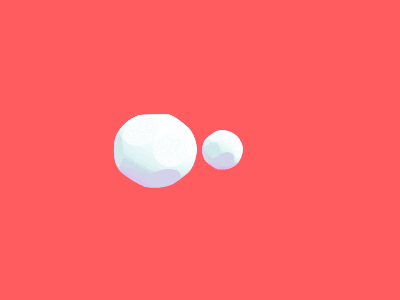
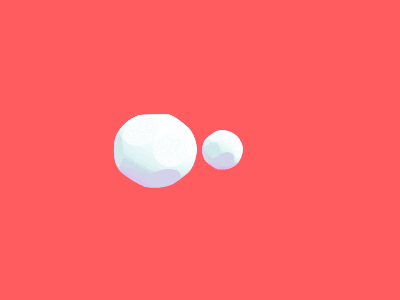
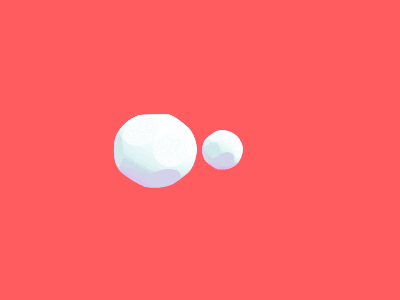
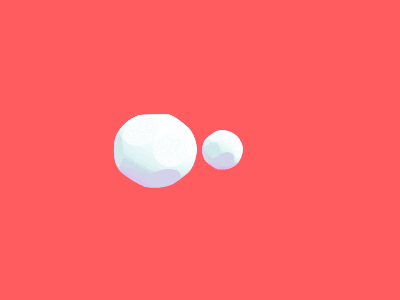
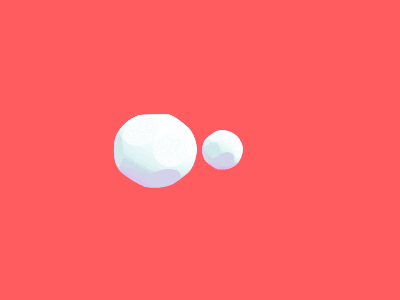
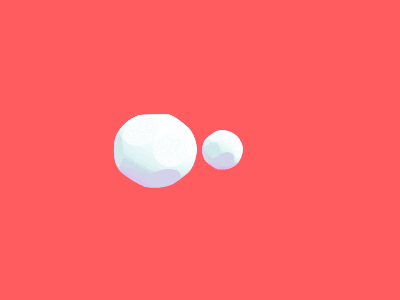
Particle-Filtering-based Latent Diffusion for Inverse Problems
Authors:Amir Nazemi, Mohammad Hadi Sepanj, Nicholas Pellegrino, Chris Czarnecki, Paul Fieguth
Current strategies for solving image-based inverse problems apply latent diffusion models to perform posterior sampling.However, almost all approaches make no explicit attempt to explore the solution space, instead drawing only a single sample from a Gaussian distribution from which to generate their solution. In this paper, we introduce a particle-filtering-based framework for a nonlinear exploration of the solution space in the initial stages of reverse SDE methods. Our proposed particle-filtering-based latent diffusion (PFLD) method and proposed problem formulation and framework can be applied to any diffusion-based solution for linear or nonlinear inverse problems. Our experimental results show that PFLD outperforms the SoTA solver PSLD on the FFHQ-1K and ImageNet-1K datasets on inverse problem tasks of super resolution, Gaussian debluring and inpainting.
PDF Mohammad Hadi Sepanj, Nicholas Pellegrino, and Chris Czarnecki contributed equally
点此查看论文截图
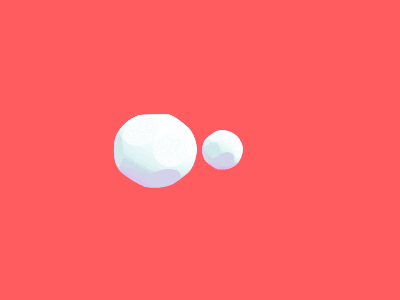
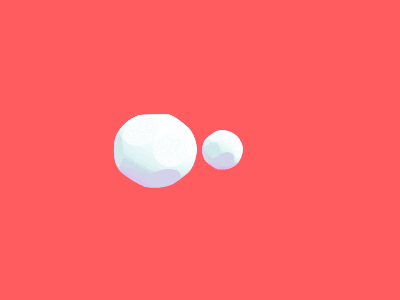
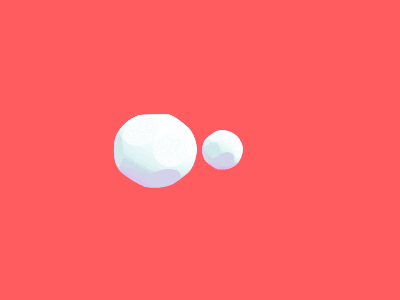
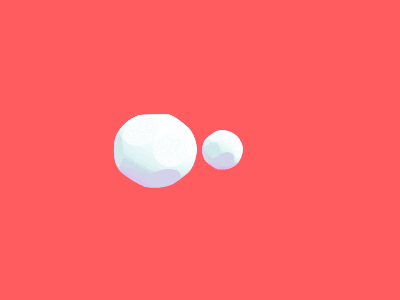
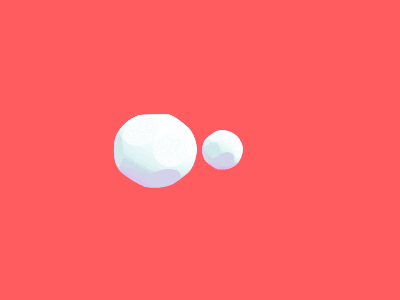
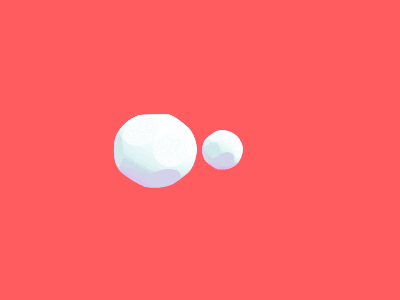
SurGen: Text-Guided Diffusion Model for Surgical Video Generation
Authors:Joseph Cho, Samuel Schmidgall, Cyril Zakka, Mrudang Mathur, Rohan Shad, William Hiesinger
Diffusion-based video generation models have made significant strides, producing outputs with improved visual fidelity, temporal coherence, and user control. These advancements hold great promise for improving surgical education by enabling more realistic, diverse, and interactive simulation environments. In this study, we introduce SurGen, a text-guided diffusion model tailored for surgical video synthesis, producing the highest resolution and longest duration videos among existing surgical video generation models. We validate the visual and temporal quality of the outputs using standard image and video generation metrics. Additionally, we assess their alignment to the corresponding text prompts through a deep learning classifier trained on surgical data. Our results demonstrate the potential of diffusion models to serve as valuable educational tools for surgical trainees.
PDF
点此查看论文截图
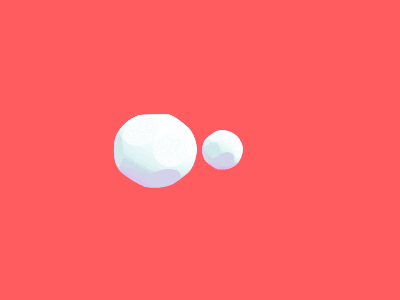
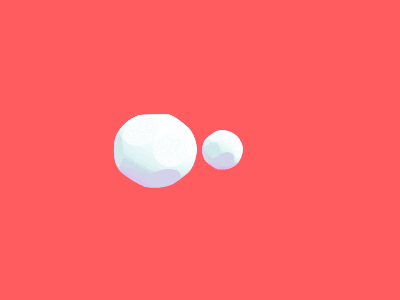
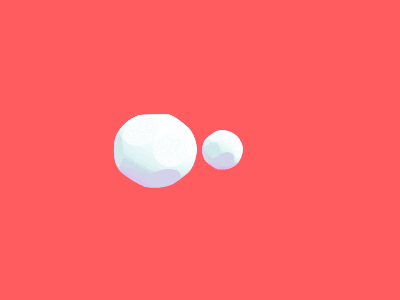
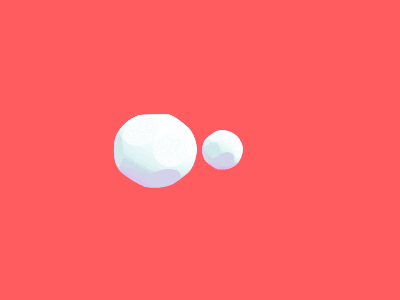
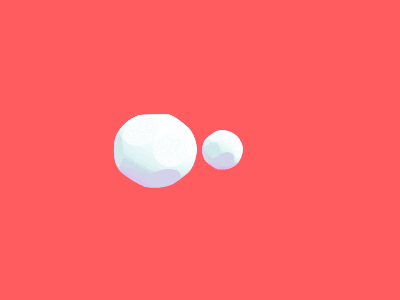
SwiftBrush v2: Make Your One-step Diffusion Model Better Than Its Teacher
Authors:Trung Dao, Thuan Hoang Nguyen, Thanh Le, Duc Vu, Khoi Nguyen, Cuong Pham, Anh Tran
In this paper, we aim to enhance the performance of SwiftBrush, a prominent one-step text-to-image diffusion model, to be competitive with its multi-step Stable Diffusion counterpart. Initially, we explore the quality-diversity trade-off between SwiftBrush and SD Turbo: the former excels in image diversity, while the latter excels in image quality. This observation motivates our proposed modifications in the training methodology, including better weight initialization and efficient LoRA training. Moreover, our introduction of a novel clamped CLIP loss enhances image-text alignment and results in improved image quality. Remarkably, by combining the weights of models trained with efficient LoRA and full training, we achieve a new state-of-the-art one-step diffusion model, achieving an FID of 8.14 and surpassing all GAN-based and multi-step Stable Diffusion models. The evaluation code is available at: https://github.com/vinairesearch/swiftbrushv2.
PDF Accepted to ECCV’24
点此查看论文截图
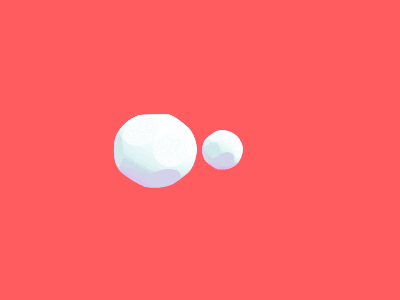
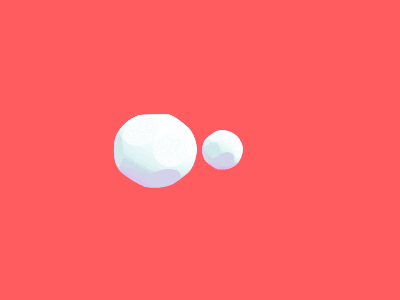
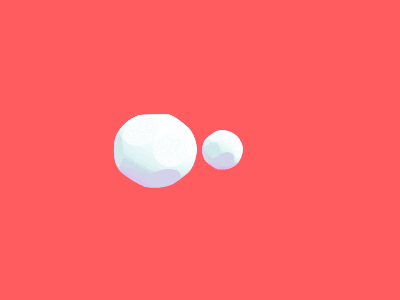
MagicMan: Generative Novel View Synthesis of Humans with 3D-Aware Diffusion and Iterative Refinement
Authors:Xu He, Xiaoyu Li, Di Kang, Jiangnan Ye, Chaopeng Zhang, Liyang Chen, Xiangjun Gao, Han Zhang, Zhiyong Wu, Haolin Zhuang
Existing works in single-image human reconstruction suffer from weak generalizability due to insufficient training data or 3D inconsistencies for a lack of comprehensive multi-view knowledge. In this paper, we introduce MagicMan, a human-specific multi-view diffusion model designed to generate high-quality novel view images from a single reference image. As its core, we leverage a pre-trained 2D diffusion model as the generative prior for generalizability, with the parametric SMPL-X model as the 3D body prior to promote 3D awareness. To tackle the critical challenge of maintaining consistency while achieving dense multi-view generation for improved 3D human reconstruction, we first introduce hybrid multi-view attention to facilitate both efficient and thorough information interchange across different views. Additionally, we present a geometry-aware dual branch to perform concurrent generation in both RGB and normal domains, further enhancing consistency via geometry cues. Last but not least, to address ill-shaped issues arising from inaccurate SMPL-X estimation that conflicts with the reference image, we propose a novel iterative refinement strategy, which progressively optimizes SMPL-X accuracy while enhancing the quality and consistency of the generated multi-views. Extensive experimental results demonstrate that our method significantly outperforms existing approaches in both novel view synthesis and subsequent 3D human reconstruction tasks.
PDF Project Page: https://thuhcsi.github.io/MagicMan
点此查看论文截图
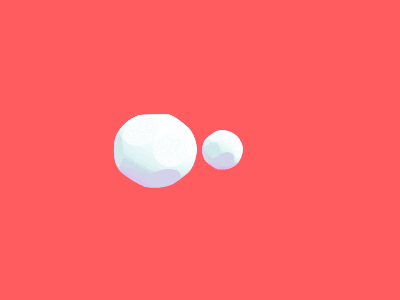
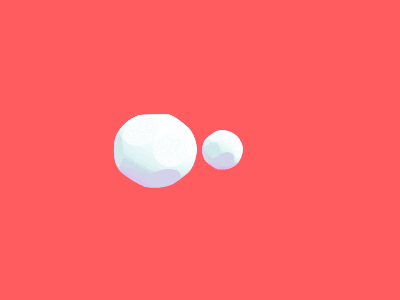
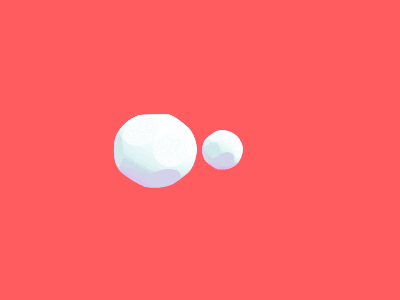
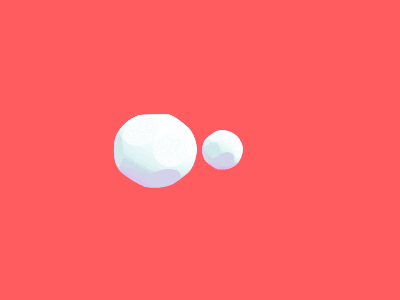
TC-PDM: Temporally Consistent Patch Diffusion Models for Infrared-to-Visible Video Translation
Authors:Anh-Dzung Doan, Vu Minh Hieu Phan, Surabhi Gupta, Markus Wagner, Tat-Jun Chin, Ian Reid
Infrared imaging offers resilience against changing lighting conditions by capturing object temperatures. Yet, in few scenarios, its lack of visual details compared to daytime visible images, poses a significant challenge for human and machine interpretation. This paper proposes a novel diffusion method, dubbed Temporally Consistent Patch Diffusion Models (TC-DPM), for infrared-to-visible video translation. Our method, extending the Patch Diffusion Model, consists of two key components. Firstly, we propose a semantic-guided denoising, leveraging the strong representations of foundational models. As such, our method faithfully preserves the semantic structure of generated visible images. Secondly, we propose a novel temporal blending module to guide the denoising trajectory, ensuring the temporal consistency between consecutive frames. Experiment shows that TC-PDM outperforms state-of-the-art methods by 35.3% in FVD for infrared-to-visible video translation and by 6.1% in AP50 for day-to-night object detection. Our code is publicly available at https://github.com/dzungdoan6/tc-pdm
PDF Technical report
点此查看论文截图
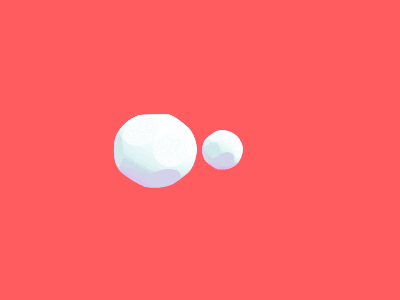
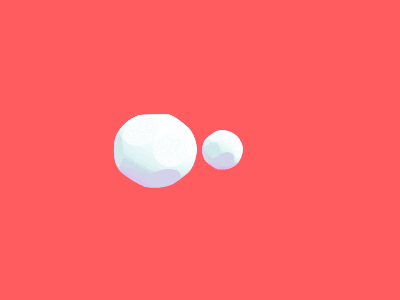
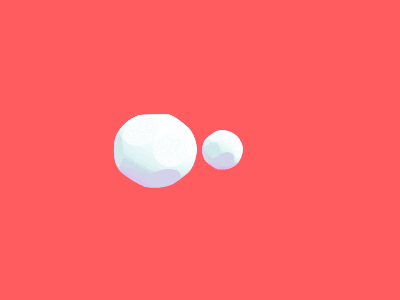
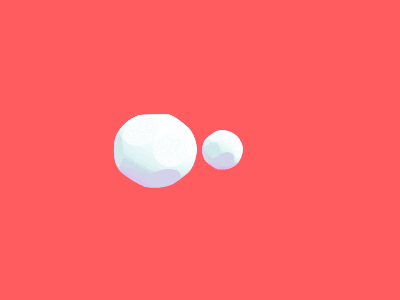
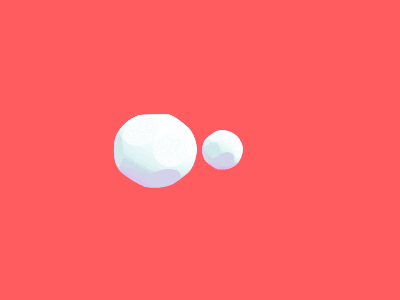
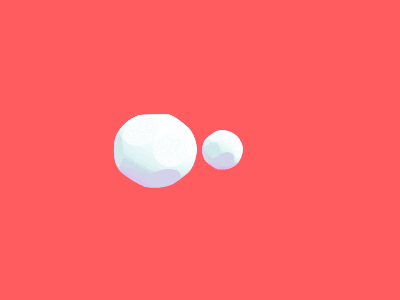
K-Sort Arena: Efficient and Reliable Benchmarking for Generative Models via K-wise Human Preferences
Authors:Zhikai Li, Xuewen Liu, Dongrong Fu, Jianquan Li, Qingyi Gu, Kurt Keutzer, Zhen Dong
The rapid advancement of visual generative models necessitates efficient and reliable evaluation methods. Arena platform, which gathers user votes on model comparisons, can rank models with human preferences. However, traditional Arena methods, while established, require an excessive number of comparisons for ranking to converge and are vulnerable to preference noise in voting, suggesting the need for better approaches tailored to contemporary evaluation challenges. In this paper, we introduce K-Sort Arena, an efficient and reliable platform based on a key insight: images and videos possess higher perceptual intuitiveness than texts, enabling rapid evaluation of multiple samples simultaneously. Consequently, K-Sort Arena employs K-wise comparisons, allowing K models to engage in free-for-all competitions, which yield much richer information than pairwise comparisons. To enhance the robustness of the system, we leverage probabilistic modeling and Bayesian updating techniques. We propose an exploration-exploitation-based matchmaking strategy to facilitate more informative comparisons. In our experiments, K-Sort Arena exhibits 16.3x faster convergence compared to the widely used ELO algorithm. To further validate the superiority and obtain a comprehensive leaderboard, we collect human feedback via crowdsourced evaluations of numerous cutting-edge text-to-image and text-to-video models. Thanks to its high efficiency, K-Sort Arena can continuously incorporate emerging models and update the leaderboard with minimal votes. Our project has undergone several months of internal testing and is now available at https://huggingface.co/spaces/ksort/K-Sort-Arena
PDF Project page: https://huggingface.co/spaces/ksort/K-Sort-Arena
点此查看论文截图
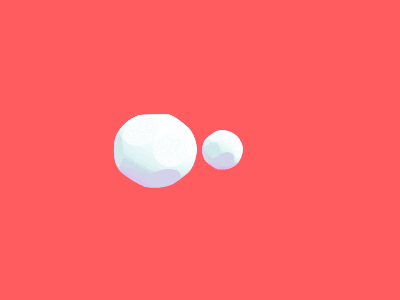
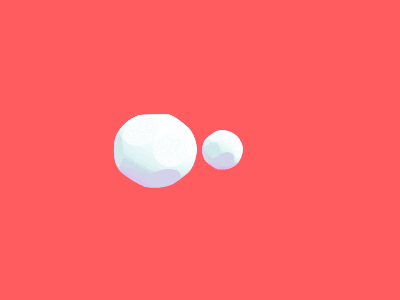
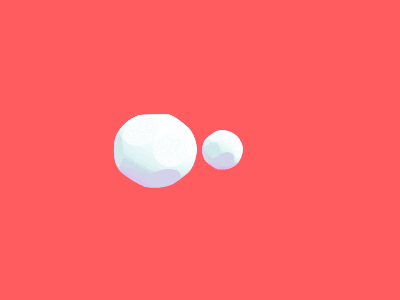
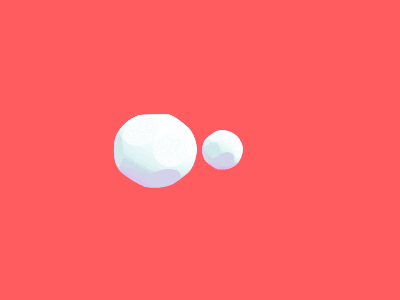