2024-08-27 更新
UNetMamba: An Efficient UNet-Like Mamba for Semantic Segmentation of High-Resolution Remote Sensing Images
Authors:Enze Zhu, Zhan Chen, Dingkai Wang, Hanru Shi, Xiaoxuan Liu, Lei Wang
Semantic segmentation of high-resolution remote sensing images is vital in downstream applications such as land-cover mapping, urban planning and disaster assessment.Existing Transformer-based methods suffer from the constraint between accuracy and efficiency, while the recently proposed Mamba is renowned for being efficient. Therefore, to overcome the dilemma, we propose UNetMamba, a UNet-like semantic segmentation model based on Mamba. It incorporates a mamba segmentation decoder (MSD) that can efficiently decode the complex information within high-resolution images, and a local supervision module (LSM), which is train-only but can significantly enhance the perception of local contents. Extensive experiments demonstrate that UNetMamba outperforms the state-of-the-art methods with mIoU increased by 0.87% on LoveDA and 0.36% on ISPRS Vaihingen, while achieving high efficiency through the lightweight design, less memory footprint and reduced computational cost. The source code is available at https://github.com/EnzeZhu2001/UNetMamba.
PDF 5 pages, 3 figures
点此查看论文截图
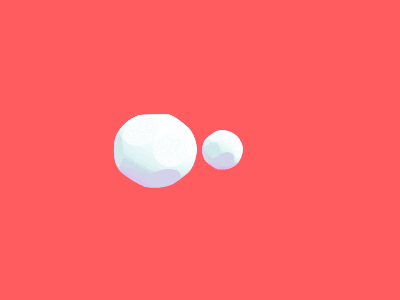
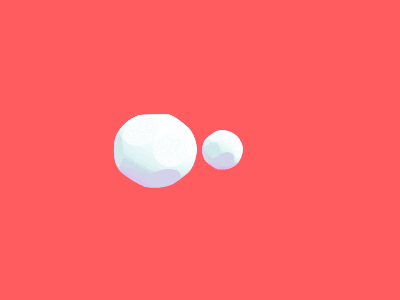
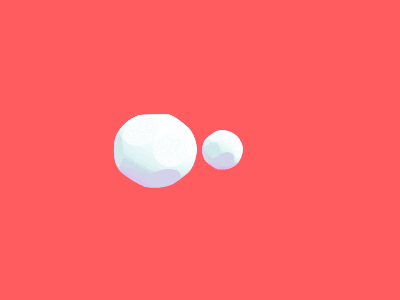
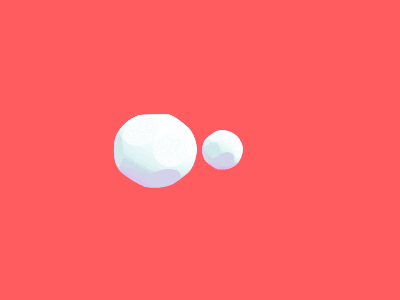
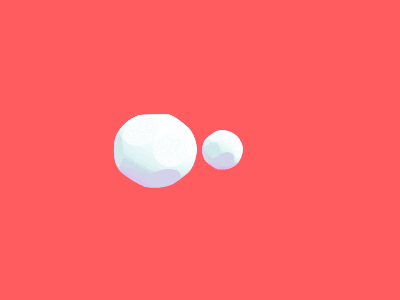
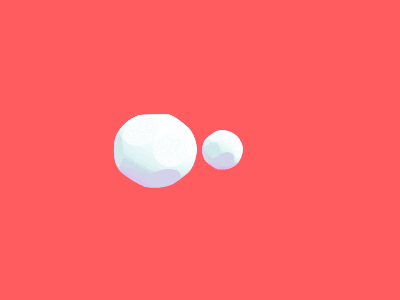
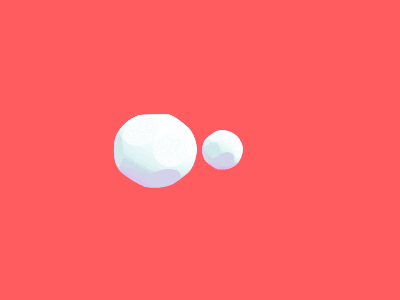
ESA: Annotation-Efficient Active Learning for Semantic Segmentation
Authors:Jinchao Ge, Zeyu Zhang, Minh Hieu Phan, Bowen Zhang, Akide Liu, Yang Zhao
Active learning enhances annotation efficiency by selecting the most revealing samples for labeling, thereby reducing reliance on extensive human input. Previous methods in semantic segmentation have centered on individual pixels or small areas, neglecting the rich patterns in natural images and the power of advanced pre-trained models. To address these challenges, we propose three key contributions: Firstly, we introduce Entity-Superpixel Annotation (ESA), an innovative and efficient active learning strategy which utilizes a class-agnostic mask proposal network coupled with super-pixel grouping to capture local structural cues. Additionally, our method selects a subset of entities within each image of the target domain, prioritizing superpixels with high entropy to ensure comprehensive representation. Simultaneously, it focuses on a limited number of key entities, thereby optimizing for efficiency. By utilizing an annotator-friendly design that capitalizes on the inherent structure of images, our approach significantly outperforms existing pixel-based methods, achieving superior results with minimal queries, specifically reducing click cost by 98% and enhancing performance by 1.71%. For instance, our technique requires a mere 40 clicks for annotation, a stark contrast to the 5000 clicks demanded by conventional methods.
PDF
点此查看论文截图
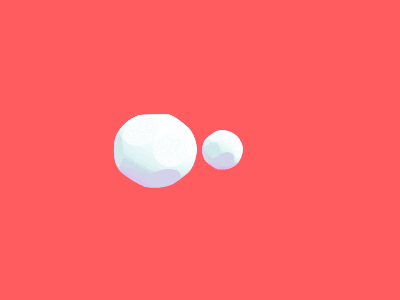
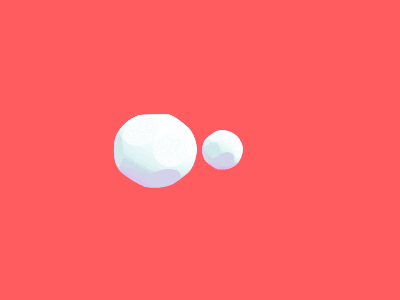
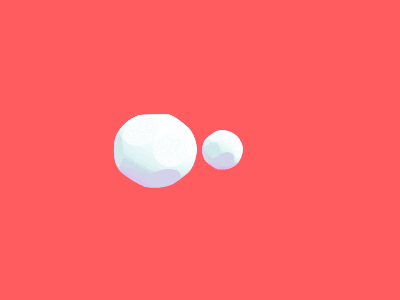
Exploring Reliable Matching with Phase Enhancement for Night-time Semantic Segmentation
Authors:Yuwen Pan, Rui Sun, Naisong Luo, Tianzhu Zhang, Yongdong Zhang
Semantic segmentation of night-time images holds significant importance in computer vision, particularly for applications like night environment perception in autonomous driving systems. However, existing methods tend to parse night-time images from a day-time perspective, leaving the inherent challenges in low-light conditions (such as compromised texture and deceiving matching errors) unexplored. To address these issues, we propose a novel end-to-end optimized approach, named NightFormer, tailored for night-time semantic segmentation, avoiding the conventional practice of forcibly fitting night-time images into day-time distributions. Specifically, we design a pixel-level texture enhancement module to acquire texture-aware features hierarchically with phase enhancement and amplified attention, and an object-level reliable matching module to realize accurate association matching via reliable attention in low-light environments. Extensive experimental results on various challenging benchmarks including NightCity, BDD and Cityscapes demonstrate that our proposed method performs favorably against state-of-the-art night-time semantic segmentation methods.
PDF ECCV 2024
点此查看论文截图
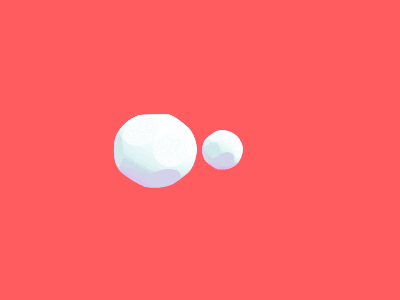
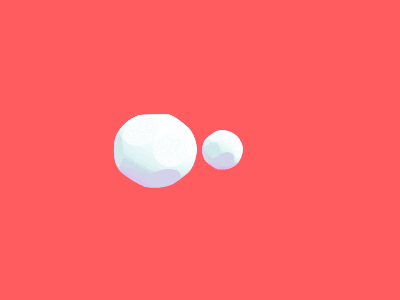
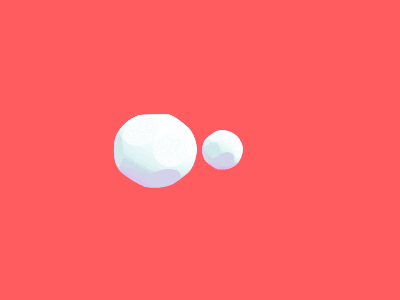
OpenNav: Efficient Open Vocabulary 3D Object Detection for Smart Wheelchair Navigation
Authors:Muhammad Rameez ur Rahman, Piero Simonetto, Anna Polato, Francesco Pasti, Luca Tonin, Sebastiano Vascon
Open vocabulary 3D object detection (OV3D) allows precise and extensible object recognition crucial for adapting to diverse environments encountered in assistive robotics. This paper presents OpenNav, a zero-shot 3D object detection pipeline based on RGB-D images for smart wheelchairs. Our pipeline integrates an open-vocabulary 2D object detector with a mask generator for semantic segmentation, followed by depth isolation and point cloud construction to create 3D bounding boxes. The smart wheelchair exploits these 3D bounding boxes to identify potential targets and navigate safely. We demonstrate OpenNav’s performance through experiments on the Replica dataset and we report preliminary results with a real wheelchair. OpenNav improves state-of-the-art significantly on the Replica dataset at mAP25 (+9pts) and mAP50 (+5pts) with marginal improvement at mAP. The code is publicly available at this link: https://github.com/EasyWalk-PRIN/OpenNav.
PDF ECCVW
点此查看论文截图
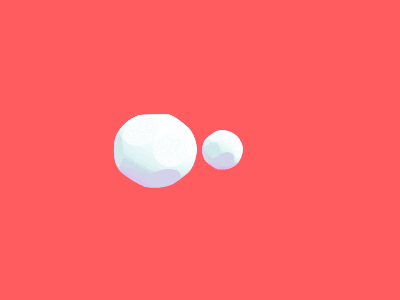
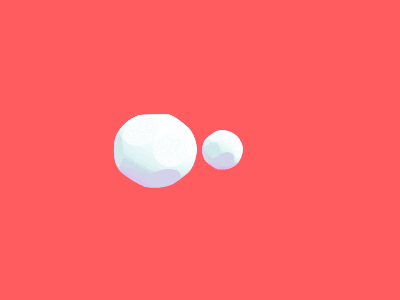
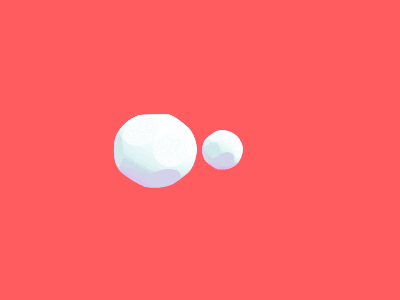
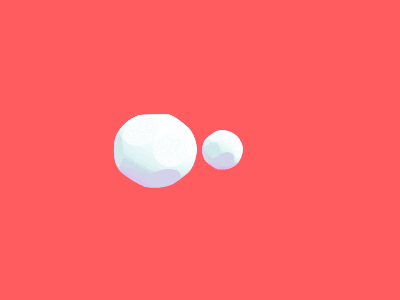
InterTrack: Tracking Human Object Interaction without Object Templates
Authors:Xianghui Xie, Jan Eric Lenssen, Gerard Pons-Moll
Tracking human object interaction from videos is important to understand human behavior from the rapidly growing stream of video data. Previous video-based methods require predefined object templates while single-image-based methods are template-free but lack temporal consistency. In this paper, we present a method to track human object interaction without any object shape templates. We decompose the 4D tracking problem into per-frame pose tracking and canonical shape optimization. We first apply a single-view reconstruction method to obtain temporally-inconsistent per-frame interaction reconstructions. Then, for the human, we propose an efficient autoencoder to predict SMPL vertices directly from the per-frame reconstructions, introducing temporally consistent correspondence. For the object, we introduce a pose estimator that leverages temporal information to predict smooth object rotations under occlusions. To train our model, we propose a method to generate synthetic interaction videos and synthesize in total 10 hour videos of 8.5k sequences with full 3D ground truth. Experiments on BEHAVE and InterCap show that our method significantly outperforms previous template-based video tracking and single-frame reconstruction methods. Our proposed synthetic video dataset also allows training video-based methods that generalize to real-world videos. Our code and dataset will be publicly released.
PDF 17 pages, 13 figures and 6 tables. Project page: https://virtualhumans.mpi-inf.mpg.de/InterTrack/
点此查看论文截图
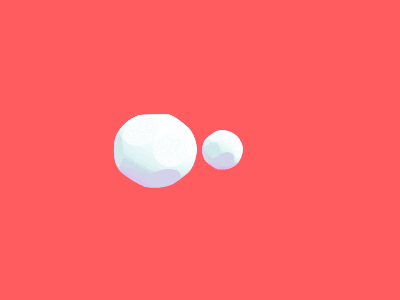
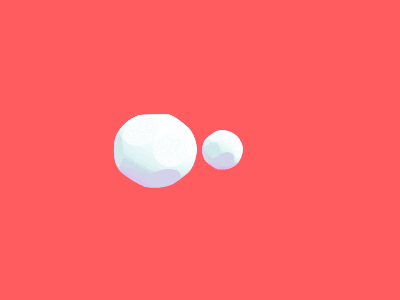
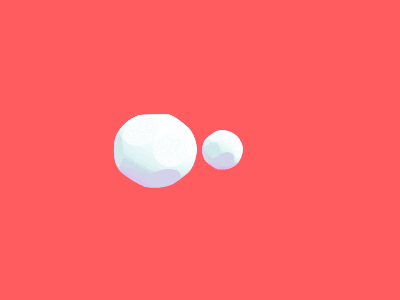
Beyond Few-shot Object Detection: A Detailed Survey
Authors:Vishal Chudasama, Hiran Sarkar, Pankaj Wasnik, Vineeth N Balasubramanian, Jayateja Kalla
Object detection is a critical field in computer vision focusing on accurately identifying and locating specific objects in images or videos. Traditional methods for object detection rely on large labeled training datasets for each object category, which can be time-consuming and expensive to collect and annotate. To address this issue, researchers have introduced few-shot object detection (FSOD) approaches that merge few-shot learning and object detection principles. These approaches allow models to quickly adapt to new object categories with only a few annotated samples. While traditional FSOD methods have been studied before, this survey paper comprehensively reviews FSOD research with a specific focus on covering different FSOD settings such as standard FSOD, generalized FSOD, incremental FSOD, open-set FSOD, and domain adaptive FSOD. These approaches play a vital role in reducing the reliance on extensive labeled datasets, particularly as the need for efficient machine learning models continues to rise. This survey paper aims to provide a comprehensive understanding of the above-mentioned few-shot settings and explore the methodologies for each FSOD task. It thoroughly compares state-of-the-art methods across different FSOD settings, analyzing them in detail based on their evaluation protocols. Additionally, it offers insights into their applications, challenges, and potential future directions in the evolving field of object detection with limited data.
PDF 43 pages, 8 figures
点此查看论文截图
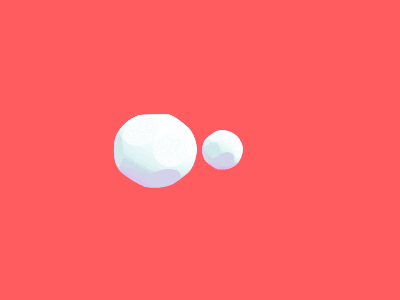
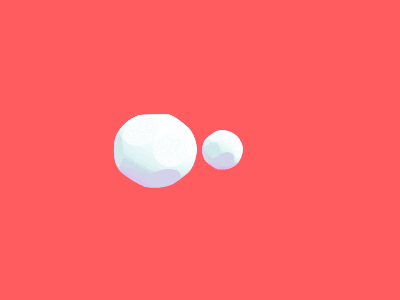
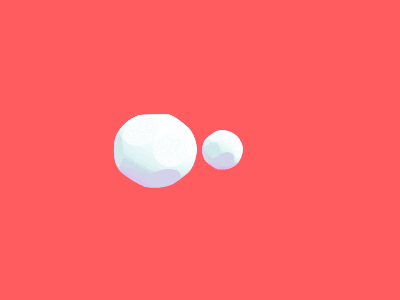
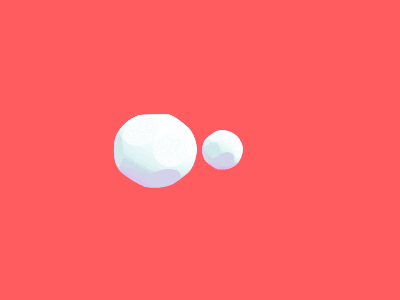
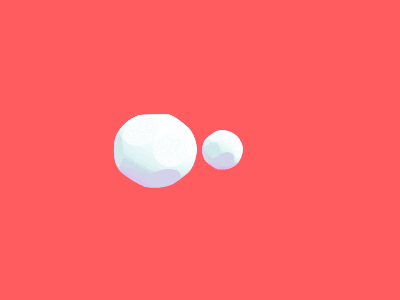