2024-04-20 更新
Multimodal 3D Object Detection on Unseen Domains
Authors:Deepti Hegde, Suhas Lohit, Kuan-Chuan Peng, Michael J. Jones, Vishal M. Patel
LiDAR datasets for autonomous driving exhibit biases in properties such as point cloud density, range, and object dimensions. As a result, object detection networks trained and evaluated in different environments often experience performance degradation. Domain adaptation approaches assume access to unannotated samples from the test distribution to address this problem. However, in the real world, the exact conditions of deployment and access to samples representative of the test dataset may be unavailable while training. We argue that the more realistic and challenging formulation is to require robustness in performance to unseen target domains. We propose to address this problem in a two-pronged manner. First, we leverage paired LiDAR-image data present in most autonomous driving datasets to perform multimodal object detection. We suggest that working with multimodal features by leveraging both images and LiDAR point clouds for scene understanding tasks results in object detectors more robust to unseen domain shifts. Second, we train a 3D object detector to learn multimodal object features across different distributions and promote feature invariance across these source domains to improve generalizability to unseen target domains. To this end, we propose CLIX$^\text{3D}$, a multimodal fusion and supervised contrastive learning framework for 3D object detection that performs alignment of object features from same-class samples of different domains while pushing the features from different classes apart. We show that CLIX$^\text{3D}$ yields state-of-the-art domain generalization performance under multiple dataset shifts.
PDF technical report
点此查看论文截图
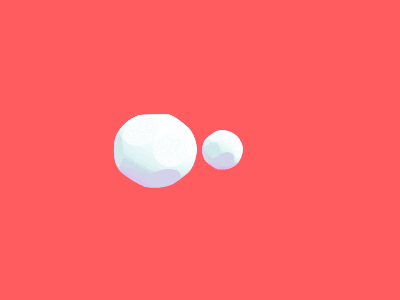
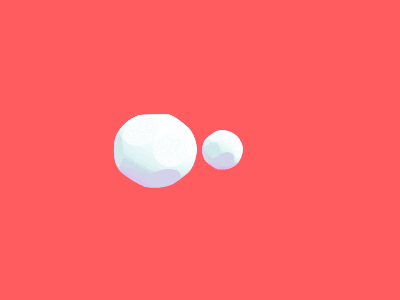
Mushroom Segmentation and 3D Pose Estimation from Point Clouds using Fully Convolutional Geometric Features and Implicit Pose Encoding
Authors:George Retsinas, Niki Efthymiou, Petros Maragos
Modern agricultural applications rely more and more on deep learning solutions. However, training well-performing deep networks requires a large amount of annotated data that may not be available and in the case of 3D annotation may not even be feasible for human annotators. In this work, we develop a deep learning approach to segment mushrooms and estimate their pose on 3D data, in the form of point clouds acquired by depth sensors. To circumvent the annotation problem, we create a synthetic dataset of mushroom scenes, where we are fully aware of 3D information, such as the pose of each mushroom. The proposed network has a fully convolutional backbone, that parses sparse 3D data, and predicts pose information that implicitly defines both instance segmentation and pose estimation task. We have validated the effectiveness of the proposed implicit-based approach for a synthetic test set, as well as provided qualitative results for a small set of real acquired point clouds with depth sensors. Code is publicly available at https://github.com/georgeretsi/mushroom-pose.
PDF
点此查看论文截图
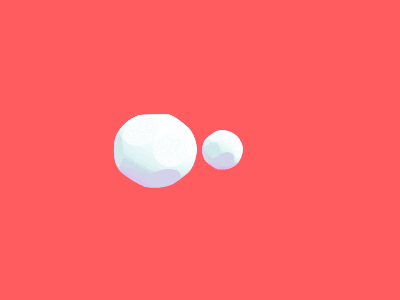
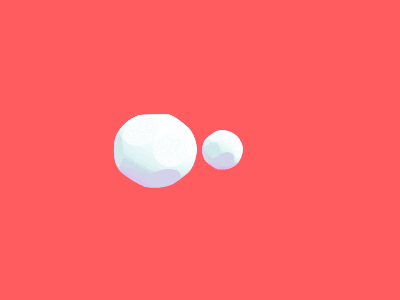
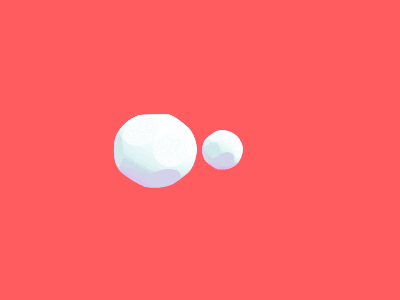
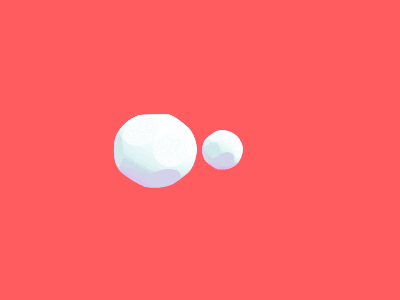
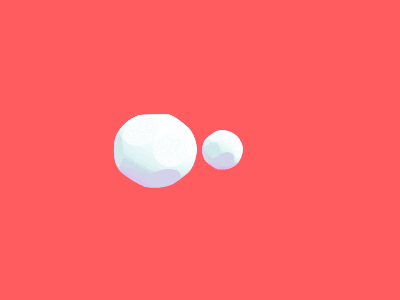
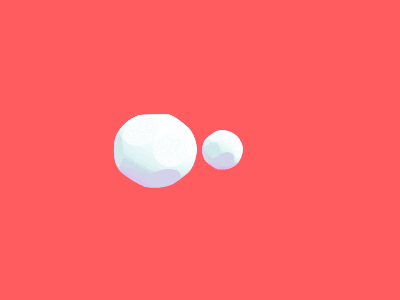
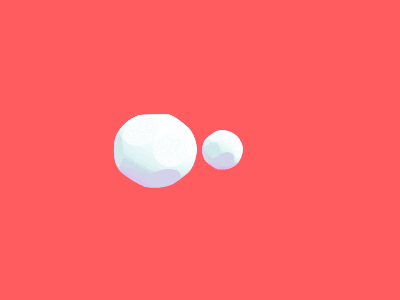