2024-04-16 更新
An inclusive review on deep learning techniques and their scope in handwriting recognition
Authors:Sukhdeep Singh, Sudhir Rohilla, Anuj Sharma
Deep learning expresses a category of machine learning algorithms that have the capability to combine raw inputs into intermediate features layers. These deep learning algorithms have demonstrated great results in different fields. Deep learning has particularly witnessed for a great achievement of human level performance across a number of domains in computer vision and pattern recognition. For the achievement of state-of-the-art performances in diverse domains, the deep learning used different architectures and these architectures used activation functions to perform various computations between hidden and output layers of any architecture. This paper presents a survey on the existing studies of deep learning in handwriting recognition field. Even though the recent progress indicates that the deep learning methods has provided valuable means for speeding up or proving accurate results in handwriting recognition, but following from the extensive literature survey, the present study finds that the deep learning has yet to revolutionize more and has to resolve many of the most pressing challenges in this field, but promising advances have been made on the prior state of the art. Additionally, an inadequate availability of labelled data to train presents problems in this domain. Nevertheless, the present handwriting recognition survey foresees deep learning enabling changes at both bench and bedside with the potential to transform several domains as image processing, speech recognition, computer vision, machine translation, robotics and control, medical imaging, medical information processing, bio-informatics, natural language processing, cyber security, and many others.
PDF
点此查看论文截图
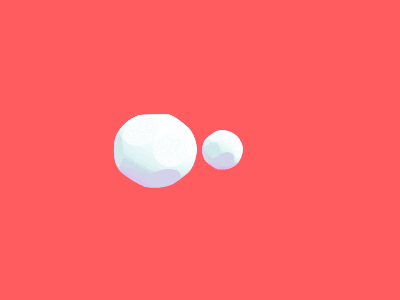
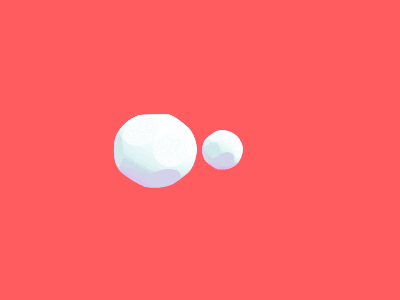
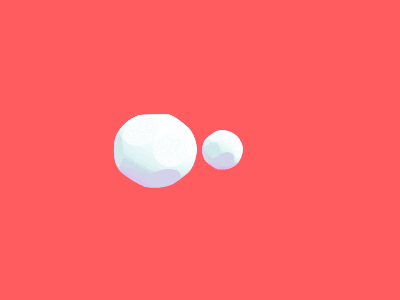
ASR advancements for indigenous languages: Quechua, Guarani, Bribri, Kotiria, and Wa’ikhana
Authors:Monica Romero, Sandra Gomez, Iván G. Torre
Indigenous languages are a fundamental legacy in the development of human communication, embodying the unique identity and culture of local communities of America. The Second AmericasNLP Competition Track 1 of NeurIPS 2022 proposed developing automatic speech recognition (ASR) systems for five indigenous languages: Quechua, Guarani, Bribri, Kotiria, and Wa’ikhana. In this paper, we propose a reliable ASR model for each target language by crawling speech corpora spanning diverse sources and applying data augmentation methods that resulted in the winning approach in this competition. To achieve this, we systematically investigated the impact of different hyperparameters by a Bayesian search on the performance of the language models, specifically focusing on the variants of the Wav2vec2.0 XLS-R model: 300M and 1B parameters. Moreover, we performed a global sensitivity analysis to assess the contribution of various hyperparametric configurations to the performances of our best models. Importantly, our results show that freeze fine-tuning updates and dropout rate are more vital parameters than the total number of epochs of lr. Additionally, we liberate our best models — with no other ASR model reported until now for two Wa’ikhana and Kotiria — and the many experiments performed to pave the way to other researchers to continue improving ASR in minority languages. This insight opens up interesting avenues for future work, allowing for the advancement of ASR techniques in the preservation of minority indigenous and acknowledging the complexities involved in this important endeavour.
PDF
点此查看论文截图
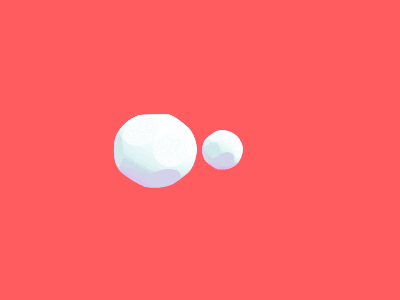
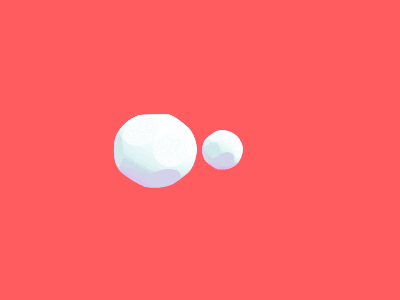
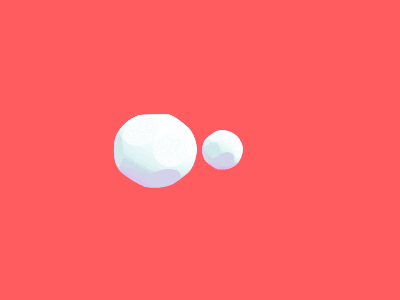