2024-04-16 更新
Balanced Mixed-Type Tabular Data Synthesis with Diffusion Models
Authors:Zeyu Yang, Peikun Guo, Khadija Zanna, Akane Sano
Diffusion models have emerged as a robust framework for various generative tasks, such as image and audio synthesis, and have also demonstrated a remarkable ability to generate mixed-type tabular data comprising both continuous and discrete variables. However, current approaches to training diffusion models on mixed-type tabular data tend to inherit the imbalanced distributions of features present in the training dataset, which can result in biased sampling. In this research, we introduce a fair diffusion model designed to generate balanced data on sensitive attributes. We present empirical evidence demonstrating that our method effectively mitigates the class imbalance in training data while maintaining the quality of the generated samples. Furthermore, we provide evidence that our approach outperforms existing methods for synthesizing tabular data in terms of performance and fairness.
PDF
点此查看论文截图
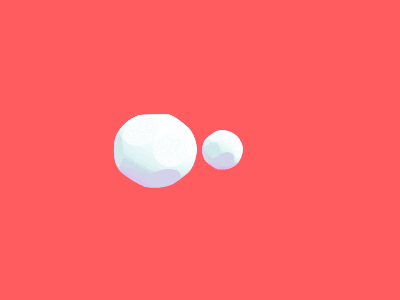
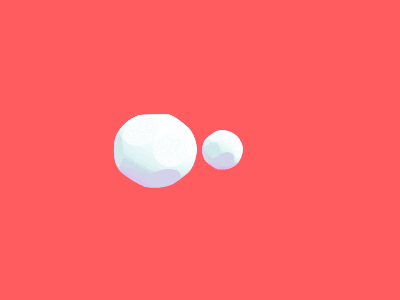
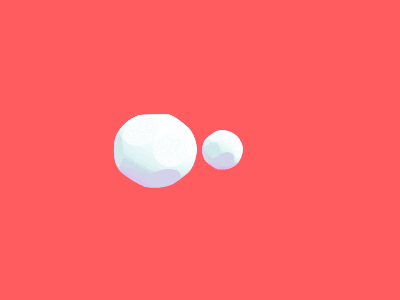
Struggle with Adversarial Defense? Try Diffusion
Authors:Yujie Li, Yanbin Wang, Haitao xu, Bin Liu, Jianguo Sun, Zhenhao Guo, Wenrui Ma
Adversarial attacks induce misclassification by introducing subtle perturbations. Recently, diffusion models are applied to the image classifiers to improve adversarial robustness through adversarial training or by purifying adversarial noise. However, diffusion-based adversarial training often encounters convergence challenges and high computational expenses. Additionally, diffusion-based purification inevitably causes data shift and is deemed susceptible to stronger adaptive attacks. To tackle these issues, we propose the Truth Maximization Diffusion Classifier (TMDC), a generative Bayesian classifier that builds upon pre-trained diffusion models and the Bayesian theorem. Unlike data-driven classifiers, TMDC, guided by Bayesian principles, utilizes the conditional likelihood from diffusion models to determine the class probabilities of input images, thereby insulating against the influences of data shift and the limitations of adversarial training. Moreover, to enhance TMDC’s resilience against more potent adversarial attacks, we propose an optimization strategy for diffusion classifiers. This strategy involves post-training the diffusion model on perturbed datasets with ground-truth labels as conditions, guiding the diffusion model to learn the data distribution and maximizing the likelihood under the ground-truth labels. The proposed method achieves state-of-the-art performance on the CIFAR10 dataset against heavy white-box attacks and strong adaptive attacks. Specifically, TMDC achieves robust accuracies of 82.81% against $l{\infty}$ norm-bounded perturbations and 86.05% against $l{2}$ norm-bounded perturbations, respectively, with $\epsilon=0.05$.
PDF
点此查看论文截图
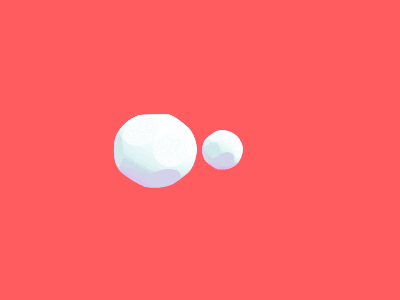
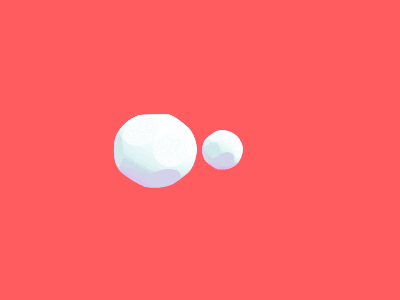
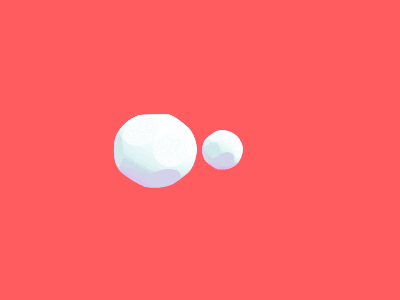
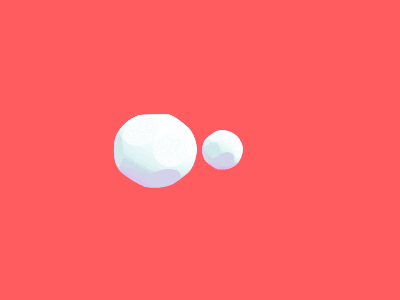
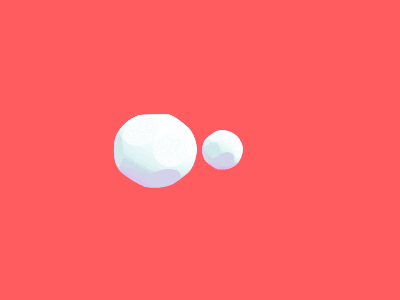