2024-04-03 更新
Disentangling Hippocampal Shape Variations: A Study of Neurological Disorders Using Graph Variational Autoencoder with Contrastive Learning
Authors:Jakaria Rabbi, Johannes Kiechle, Christian Beaulieu, Nilanjan Ray, Dana Cobzas
This paper presents a comprehensive study focused on disentangling hippocampal shape variations from diffusion tensor imaging (DTI) datasets within the context of neurological disorders. Leveraging a Graph Variational Autoencoder (VAE) enhanced with Supervised Contrastive Learning, our approach aims to improve interpretability by disentangling two distinct latent variables corresponding to age and the presence of diseases. In our ablation study, we investigate a range of VAE architectures and contrastive loss functions, showcasing the enhanced disentanglement capabilities of our approach. This evaluation uses synthetic 3D torus mesh data and real 3D hippocampal mesh datasets derived from the DTI hippocampal dataset. Our supervised disentanglement model outperforms several state-of-the-art (SOTA) methods like attribute and guided VAEs in terms of disentanglement scores. Our model distinguishes between age groups and disease status in patients with Multiple Sclerosis (MS) using the hippocampus data. Our Graph VAE with Supervised Contrastive Learning shows the volume changes of the hippocampus of MS populations at different ages, and the result is consistent with the current neuroimaging literature. This research provides valuable insights into the relationship between neurological disorder and hippocampal shape changes in different age groups of MS populations using a Graph VAE with Supervised Contrastive loss.
PDF Length: 23 pages and submitted to the journal: MELBA (Machine Learning for Biomedical Imaging)
点此查看论文截图
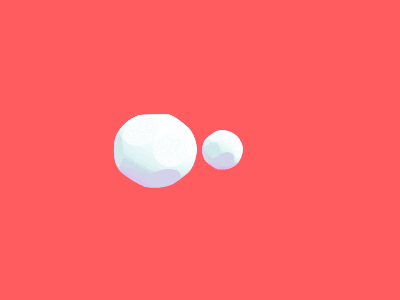
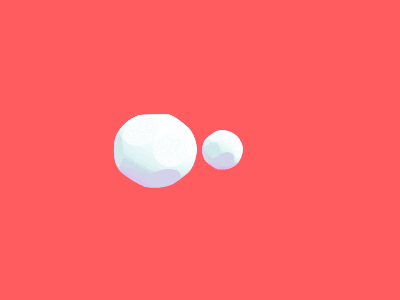
A Universal Knowledge Embedded Contrastive Learning Framework for Hyperspectral Image Classification
Authors:Quanwei Liu, Yanni Dong, Tao Huang, Lefei Zhang, Bo Do
Hyperspectral image (HSI) classification techniques have been intensively studied and a variety of models have been developed. However, these HSI classification models are confined to pocket models and unrealistic ways of datasets partitioning. The former limits the generalization performance of the model and the latter is partitioned leads to inflated model evaluation metrics, which results in plummeting model performance in the real world. Therefore, we propose a universal knowledge embedded contrastive learning framework (KnowCL) for supervised, unsupervised, and semisupervised HSI classification, which largely closes the gap of HSI classification models between pocket models and standard vision backbones. We present a new HSI processing pipeline in conjunction with a range of data transformation and augmentation techniques that provide diverse data representations and realistic data partitioning. The proposed framework based on this pipeline is compatible with all kinds of backbones and can fully exploit labeled and unlabeled samples with expected training time. Furthermore, we design a new loss function, which can adaptively fuse the supervised loss and unsupervised loss, enhancing the learning performance. This proposed new classification paradigm shows great potentials in exploring for HSI classification technology. The code can be accessed at https://github.com/quanweiliu/KnowCL.
PDF
点此查看论文截图
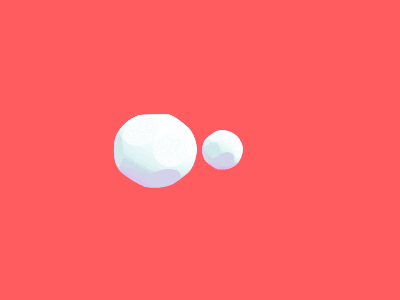
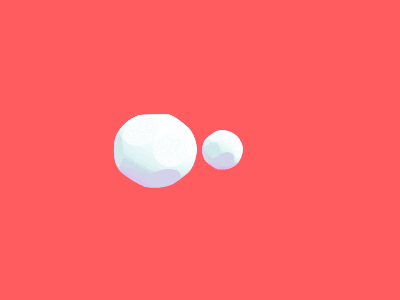
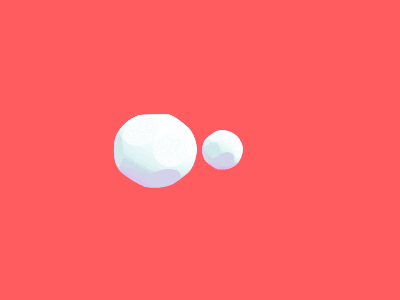
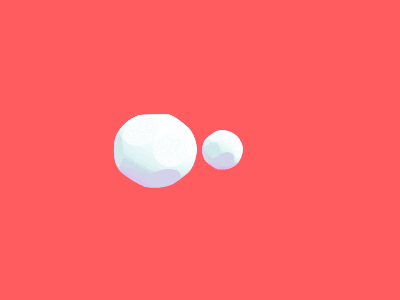
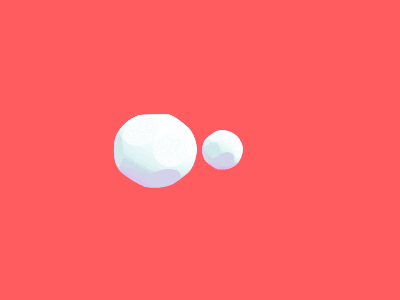
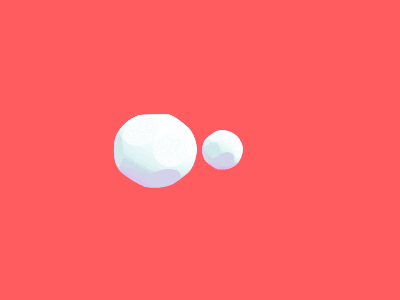