2024-04-03 更新
Utilizing Maximum Mean Discrepancy Barycenter for Propagating the Uncertainty of Value Functions in Reinforcement Learning
Authors:Srinjoy Roy, Swagatam Das
Accounting for the uncertainty of value functions boosts exploration in Reinforcement Learning (RL). Our work introduces Maximum Mean Discrepancy Q-Learning (MMD-QL) to improve Wasserstein Q-Learning (WQL) for uncertainty propagation during Temporal Difference (TD) updates. MMD-QL uses the MMD barycenter for this purpose, as MMD provides a tighter estimate of closeness between probability measures than the Wasserstein distance. Firstly, we establish that MMD-QL is Probably Approximately Correct in MDP (PAC-MDP) under the average loss metric. Concerning the accumulated rewards, experiments on tabular environments show that MMD-QL outperforms WQL and other algorithms. Secondly, we incorporate deep networks into MMD-QL to create MMD Q-Network (MMD-QN). Making reasonable assumptions, we analyze the convergence rates of MMD-QN using function approximation. Empirical results on challenging Atari games demonstrate that MMD-QN performs well compared to benchmark deep RL algorithms, highlighting its effectiveness in handling large state-action spaces.
PDF
点此查看论文截图
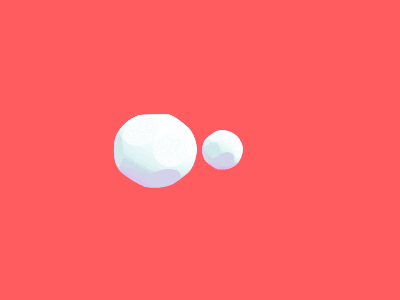
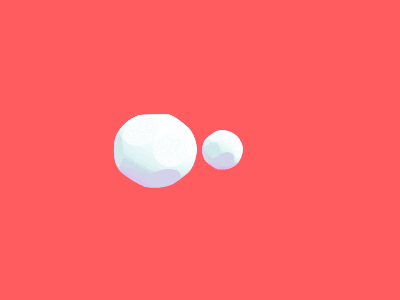
CAMO: Correlation-Aware Mask Optimization with Modulated Reinforcement Learning
Authors:Xiaoxiao Liang, Haoyu Yang, Kang Liu, Bei Yu, Yuzhe Ma
Optical proximity correction (OPC) is a vital step to ensure printability in modern VLSI manufacturing. Various OPC approaches based on machine learning have been proposed to pursue performance and efficiency, which are typically data-driven and hardly involve any particular considerations of the OPC problem, leading to potential performance or efficiency bottlenecks. In this paper, we propose CAMO, a reinforcement learning-based OPC system that specifically integrates important principles of the OPC problem. CAMO explicitly involves the spatial correlation among the movements of neighboring segments and an OPC-inspired modulation for movement action selection. Experiments are conducted on both via layer patterns and metal layer patterns. The results demonstrate that CAMO outperforms state-of-the-art OPC engines from both academia and industry.
PDF Accepted by DAC 2024
点此查看论文截图
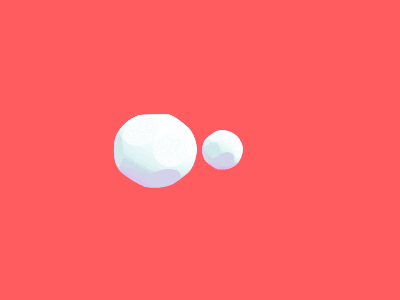
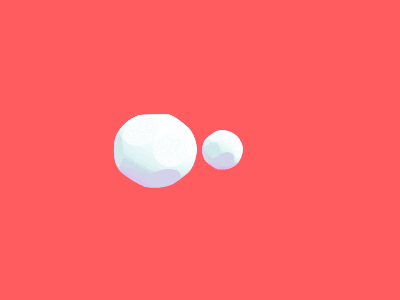
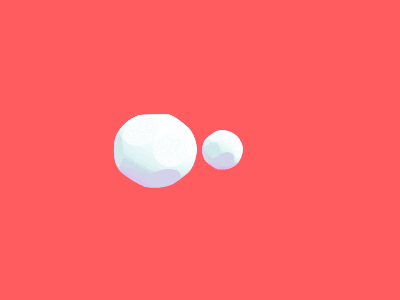
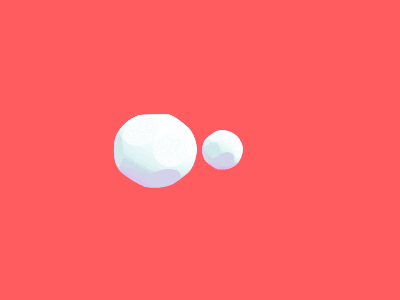
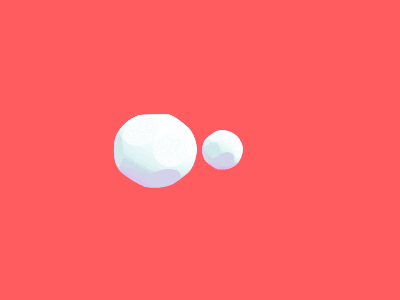
Learning to Control Camera Exposure via Reinforcement Learning
Authors:Kyunghyun Lee, Ukcheol Shin, Byeong-Uk Lee
Adjusting camera exposure in arbitrary lighting conditions is the first step to ensure the functionality of computer vision applications. Poorly adjusted camera exposure often leads to critical failure and performance degradation. Traditional camera exposure control methods require multiple convergence steps and time-consuming processes, making them unsuitable for dynamic lighting conditions. In this paper, we propose a new camera exposure control framework that rapidly controls camera exposure while performing real-time processing by exploiting deep reinforcement learning. The proposed framework consists of four contributions: 1) a simplified training ground to simulate real-world’s diverse and dynamic lighting changes, 2) flickering and image attribute-aware reward design, along with lightweight state design for real-time processing, 3) a static-to-dynamic lighting curriculum to gradually improve the agent’s exposure-adjusting capability, and 4) domain randomization techniques to alleviate the limitation of the training ground and achieve seamless generalization in the wild.As a result, our proposed method rapidly reaches a desired exposure level within five steps with real-time processing (1 ms). Also, the acquired images are well-exposed and show superiority in various computer vision tasks, such as feature extraction and object detection.
PDF Accepted at CVPR 2024, *First two authors contributed equally to this work. Project page link: https://sites.google.com/view/drl-ae
点此查看论文截图
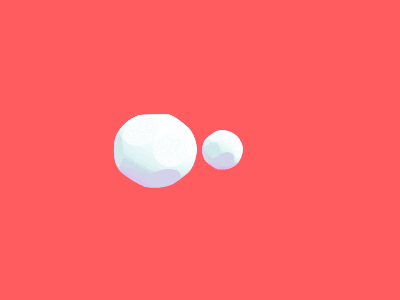
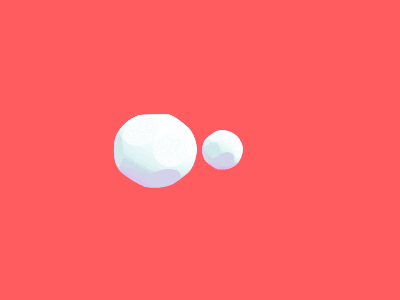
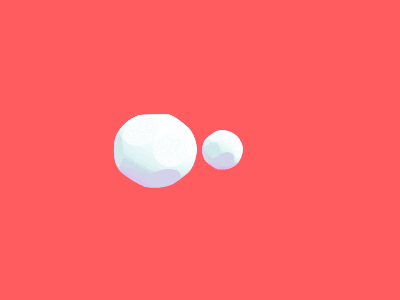
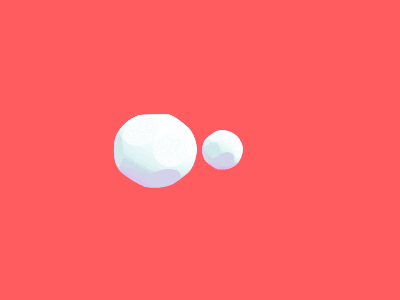