2024-04-01 更新
Hierarchical Recurrent Adapters for Efficient Multi-Task Adaptation of Large Speech Models
Authors:Tsendsuren Munkhdalai, Youzheng Chen, Khe Chai Sim, Fadi Biadsy, Tara Sainath, Pedro Moreno Mengibar
Parameter efficient adaptation methods have become a key mechanism to train large pre-trained models for downstream tasks. However, their per-task parameter overhead is considered still high when the number of downstream tasks to adapt for is large. We introduce an adapter module that has a better efficiency in large scale multi-task adaptation scenario. Our adapter is hierarchical in terms of how the adapter parameters are allocated. The adapter consists of a single shared controller network and multiple task-level adapter heads to reduce the per-task parameter overhead without performance regression on downstream tasks. The adapter is also recurrent so the entire adapter parameters are reused across different layers of the pre-trained model. Our Hierarchical Recurrent Adapter (HRA) outperforms the previous adapter-based approaches as well as full model fine-tuning baseline in both single and multi-task adaptation settings when evaluated on automatic speech recognition tasks.
PDF 5 pages, 3 figures, 5 tables
点此查看论文截图
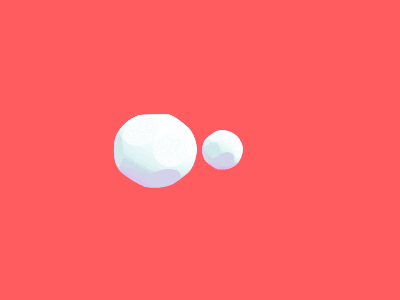
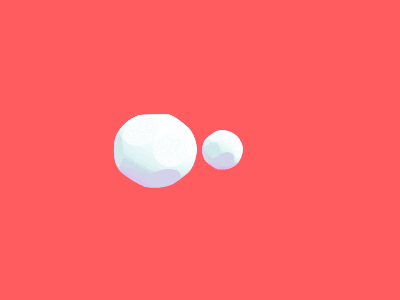
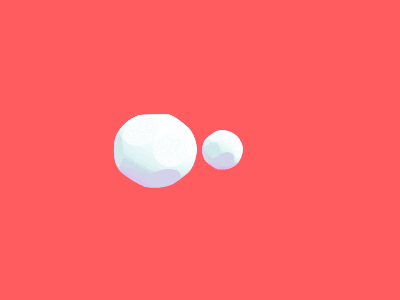
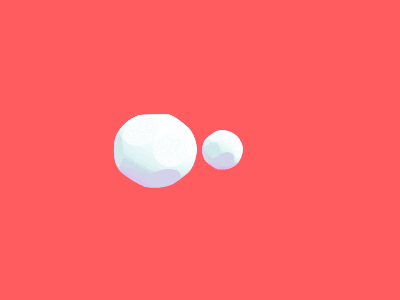
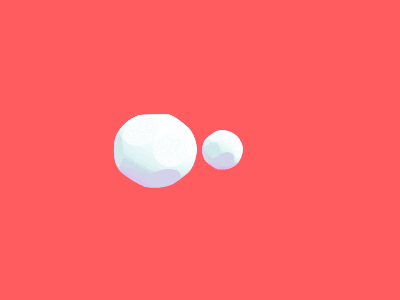
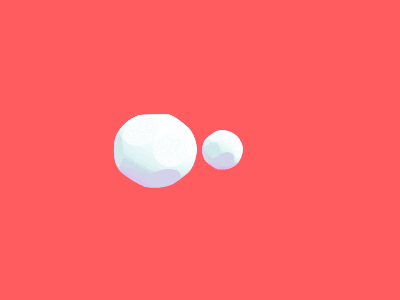
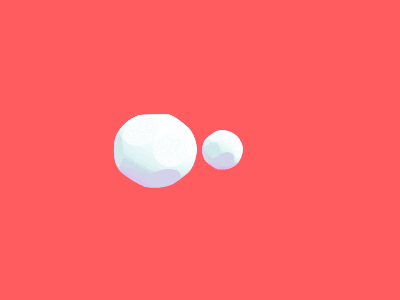